Semantic Head Enhanced Pedestrian Detection in a Crowd
Neurocomputing(2020)
摘要
Pedestrian detection in a crowd is a challenging task because of intra-class occlusion. More prior information is needed for the detector to be robust against it. Human head area is naturally a strong cue because of its stable appearance, visibility and relative location to body. Inspired by it, we adopt an extra branch to conduct semantic head detection in parallel with traditional body branch. Instead of manually labeling the head regions, we use weak annotations inferred directly from body boxes, which are named as ‘semantic head’. In this way, the head detection is formulated into using a special part of labeled box to detect the corresponding part of human body, which surprisingly improves the performance and robustness to occlusion. Moreover, the head-body alignment structure is explicitly explored by introducing Alignment Loss, which functions in a self-supervised manner. Based on these, we propose the head-body alignment net (HBAN) in this work, which aims to enhance pedestrian detection by utilizing the human head prior. Comprehensive evaluations are conducted to demonstrate the effectiveness of HBAN on CityPersons dataset. HBAN achieves about 5% increase for heavily occluded pedestrians over baseline and reaches the state-of-the-art performance.
更多查看译文
关键词
Pedestrian detection,Occlusion,Semantic head,Head-Body alignment
AI 理解论文
溯源树
样例
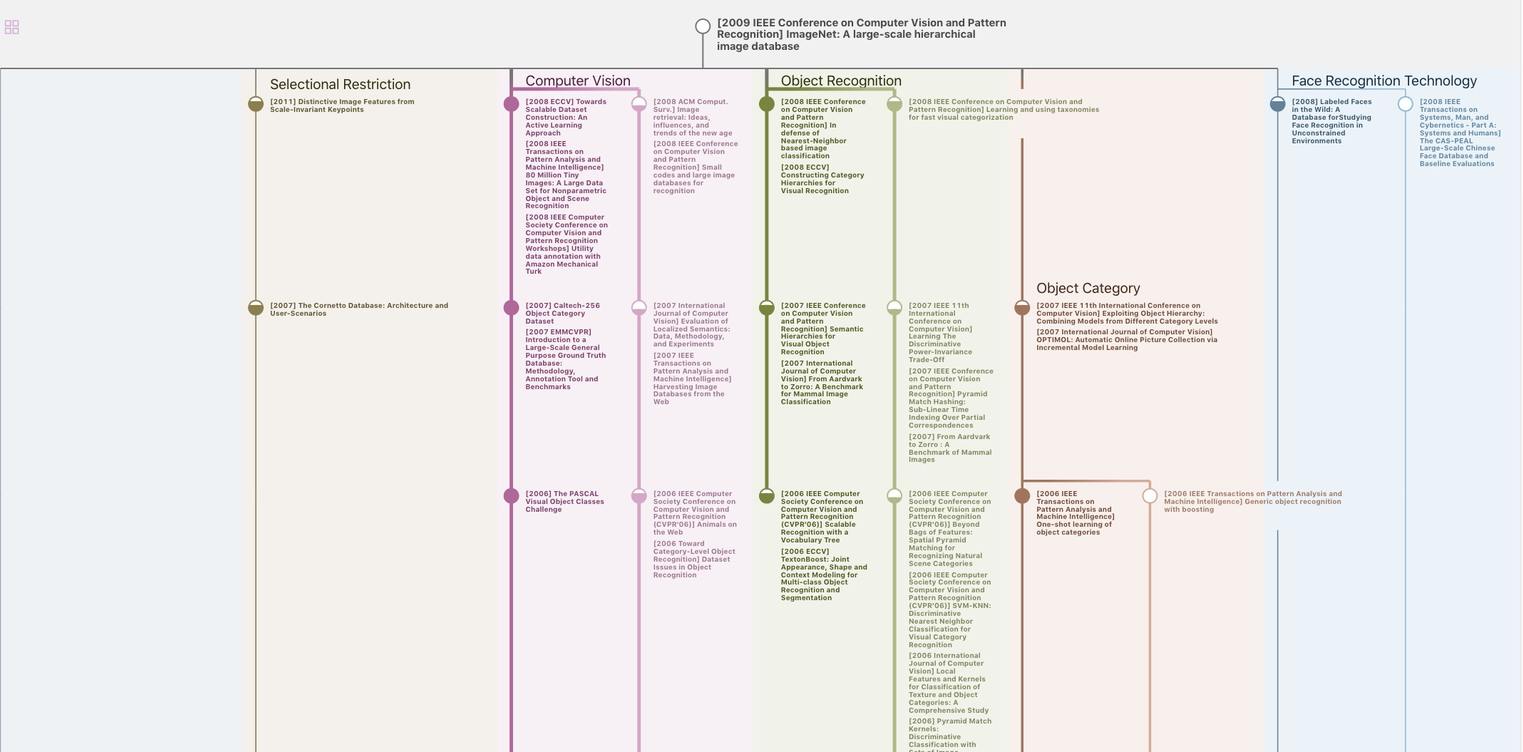
生成溯源树,研究论文发展脉络
Chat Paper
正在生成论文摘要