Prediction and Interpretable Visualization of Retrosynthetic Reactions using Graph Convolutional Networks.
JOURNAL OF CHEMICAL INFORMATION AND MODELING(2019)
摘要
Recently, many research groups have been addressing data-driven approaches for (retro)synthetic reaction prediction and retrosynthetic analysis. Although the performances of the data-driven approach have progressed because of recent advances of machine learning and deep learning techniques, problems such as improving capability of reaction prediction and the black-box problem of neural networks persist for practical use by chemists. To spread data-driven approaches to chemists, we focused on two challenges: improvement of retrosynthetic reaction prediction and interpretability of the prediction. In this paper, we propose an interpretable prediction framework using graph convolutional networks (GCN) for retrosynthetic reaction prediction and integrated gradients (IG) for visualization of contributions to the prediction to address these challenges. As a result, from the viewpoint of balanced accuracies, our model showed better performances than the approach using an extended-connectivity fingerprint. Furthermore, IG-based visualization of the GCN prediction successfully highlighted reaction-related atoms.
更多查看译文
关键词
retrosynthetic reactions,interpretable visualization,networks
AI 理解论文
溯源树
样例
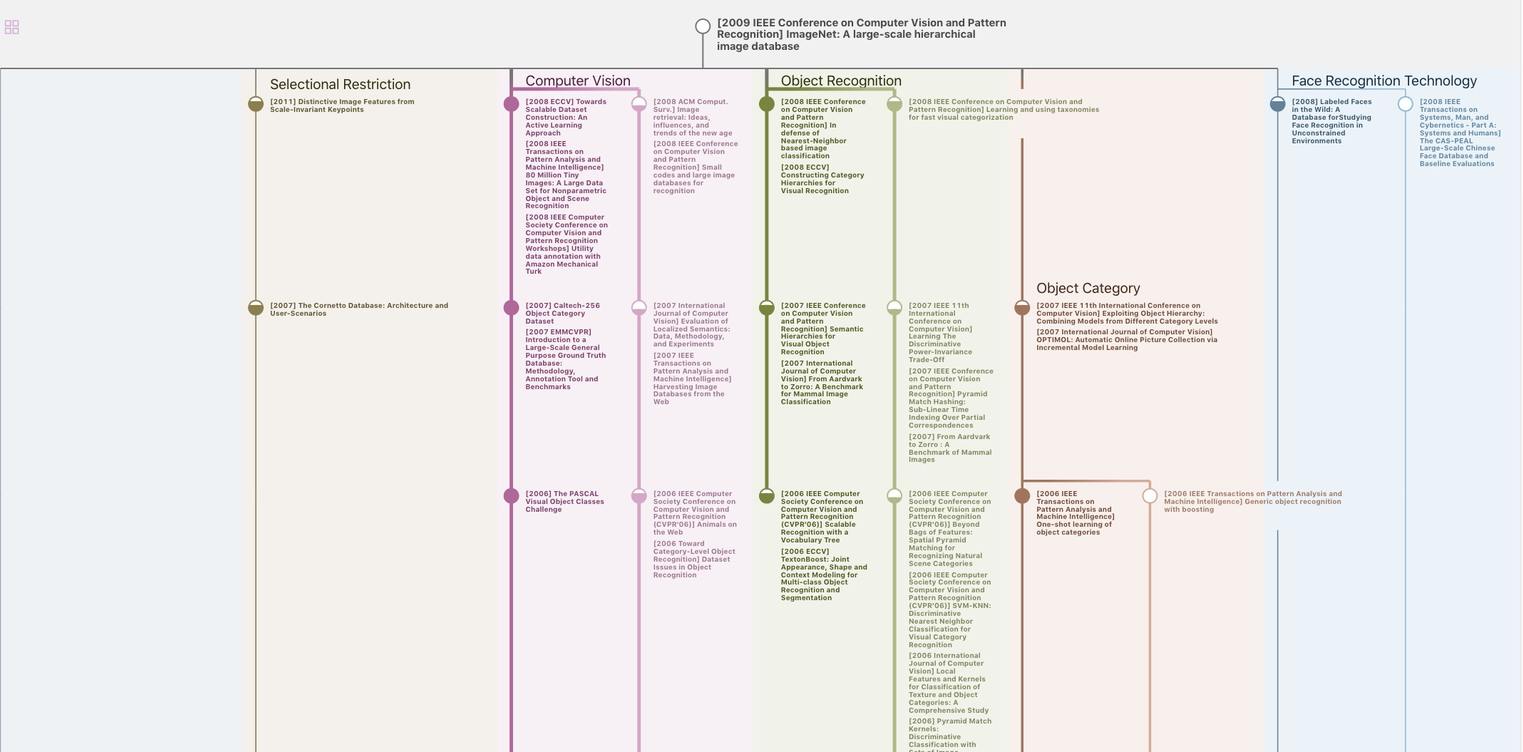
生成溯源树,研究论文发展脉络
Chat Paper
正在生成论文摘要