MR-based CT metal artifact reduction using Bayesian modelling.
PHYSICS IN MEDICINE AND BIOLOGY(2019)
摘要
Metal artifact reduction (MAR) algorithms reduce the errors caused by metal implants in x-ray computed tomography (CT) images and are an important part of error management in radiotherapy. A promising MAR approach is to leverage the information in magnetic resonance (MR) images that can be acquired for organ or tumor delineation. This is however complicated by the ambiguous relationship between CT values and conventional-sequence MR intensities as well as potential co-registration issues. In order to address these issues, this paper proposes a self-tuning Bayesian model for MR-based MAR that combines knowledge of the MR image intensities in local spatial neighborhoods with the information in an initial, corrupted CT reconstructed using filtered back projection. We demonstrate the potential of the resulting model in three widely-used MAR scenarios: image inpainting, sinogram inpainting and model-based iterative reconstruction. Compared to conventional alternatives in a retrospective study on nine head-and-neck patients with CT and T1-weighted MR scans, we find improvements in terms of image quality and quantitative CT value accuracy within each scenario. We conclude that the proposed model provides a versatile way to use the anatomical information in a co-acquired MR scan to boost the performance of MAR algorithms.
更多查看译文
关键词
computed tomography metal artifact reduction,medical imaging,Bayesian modelling,medical image analysis,radiotherapy,expectation-maximization
AI 理解论文
溯源树
样例
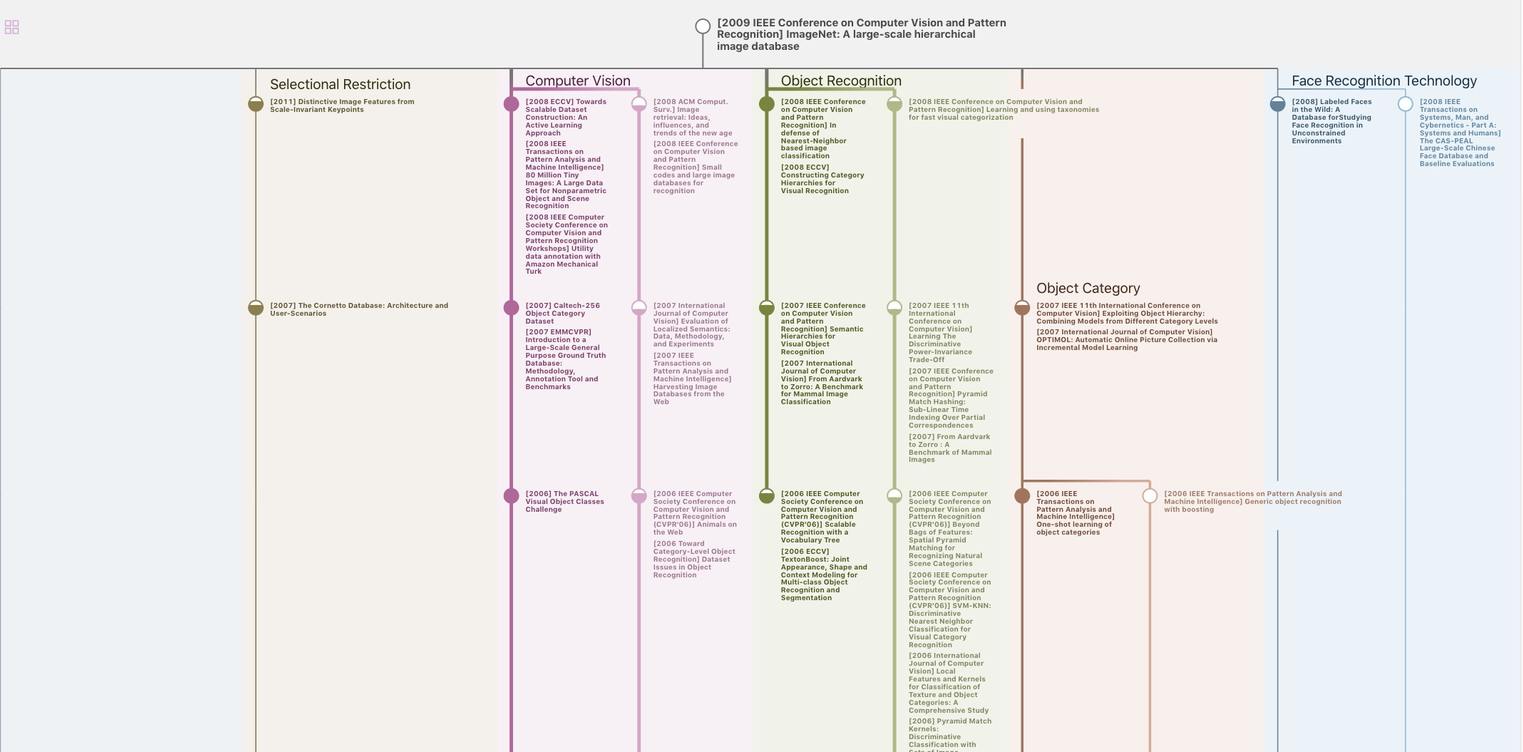
生成溯源树,研究论文发展脉络
Chat Paper
正在生成论文摘要