Generative temporal link prediction via self-tokenized sequence modeling
World Wide Web(2020)
摘要
We formalize networks with evolving structures as temporal networks and propose a generative link prediction model, Generative Link Sequence Modeling (GLSM), to predict future links for temporal networks. GLSM captures the temporal link formation patterns from the observed links with a sequence modeling framework and has the ability to generate the emerging links by inferring from the probability distribution on the potential future links. To avoid overfitting caused by treating each link as a unique token, we propose a self-tokenization mechanism to transform each raw link in the network to an abstract aggregation token automatically. The self-tokenization is seamlessly integrated into the sequence modeling framework, which allows the proposed GLSM model to have the generalization capability to discover link formation patterns beyond raw link sequences. We compare GLSM with the existing state-of-art methods on five real-world datasets. The experimental results demonstrate that GLSM obtains future positive links effectively in a generative fashion while achieving the best performance (2-10% improvements on AUC) among other alternatives.
更多查看译文
关键词
Temporal link prediction, Sequence modeling, Recurrent neural network, Self-tokenization mechanism
AI 理解论文
溯源树
样例
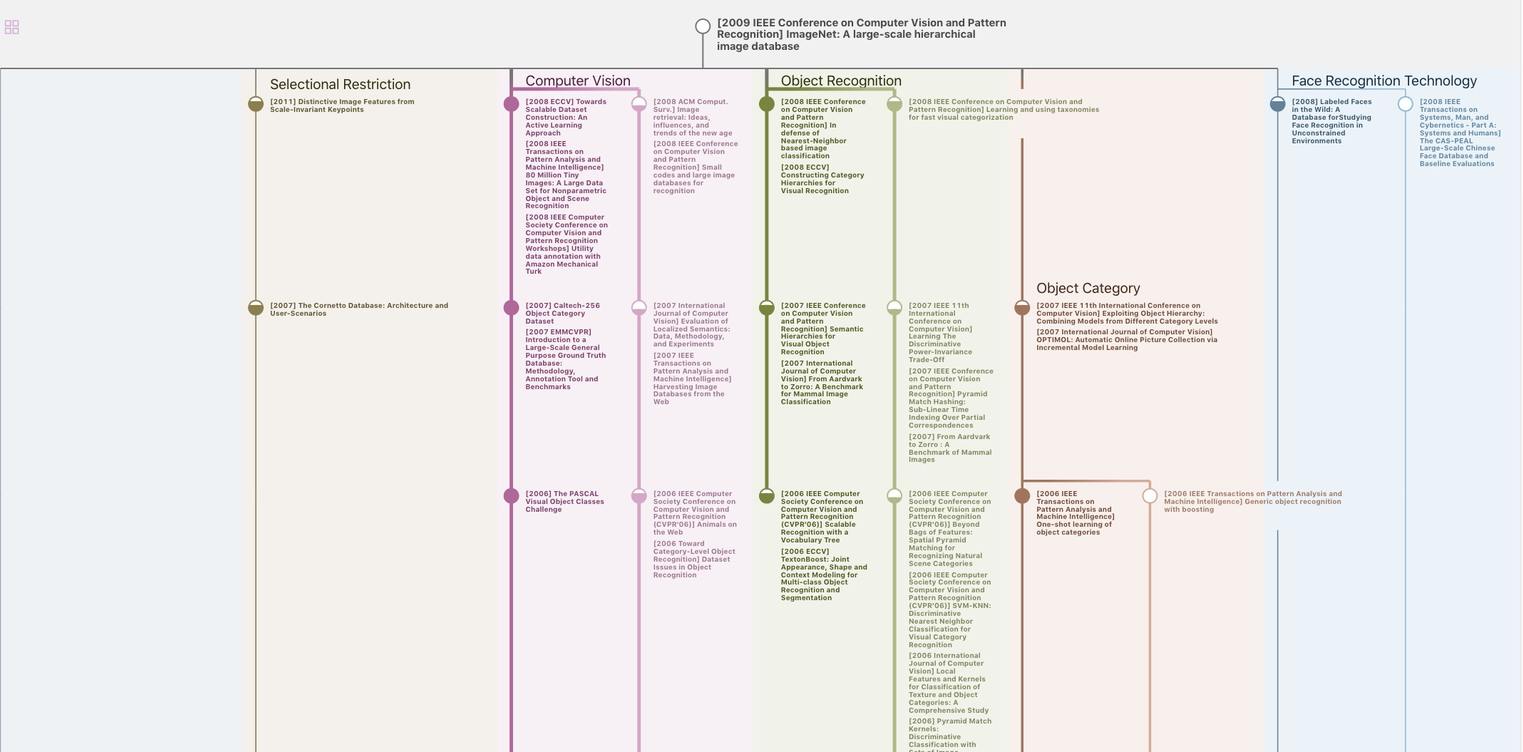
生成溯源树,研究论文发展脉络
Chat Paper
正在生成论文摘要