A Self-Tuned Architecture for Human Activity Recognition Based on a Dynamical Recurrence Analysis of Wearable Sensor Data
2019 27th European Signal Processing Conference (EUSIPCO)(2019)
摘要
Human activity recognition (HAR) is encountered in a plethora of applications, such as pervasive health care systems and smart homes. The majority of existing HAR techniques employs features extracted from symbolic or frequency-domain representations of the associated data, whilst ignoring completely the behavior of the underlying data generating dynamical system. To address this problem, this work proposes a novel self-tuned architecture for feature extraction and activity recognition by modeling directly the inherent dynamics of wearable sensor data in higher-dimensional phase spaces, which encode state recurrences for each individual activity. Experimental evaluation on real data of leisure activities demonstrates an improved recognition accuracy of our method when compared against a state-of-the-art motif-based approach using symbolic representations.
更多查看译文
关键词
Human activity recognition,recurrence quantification analysis,nonlinear data analysis,motif discovery,wearable sensors
AI 理解论文
溯源树
样例
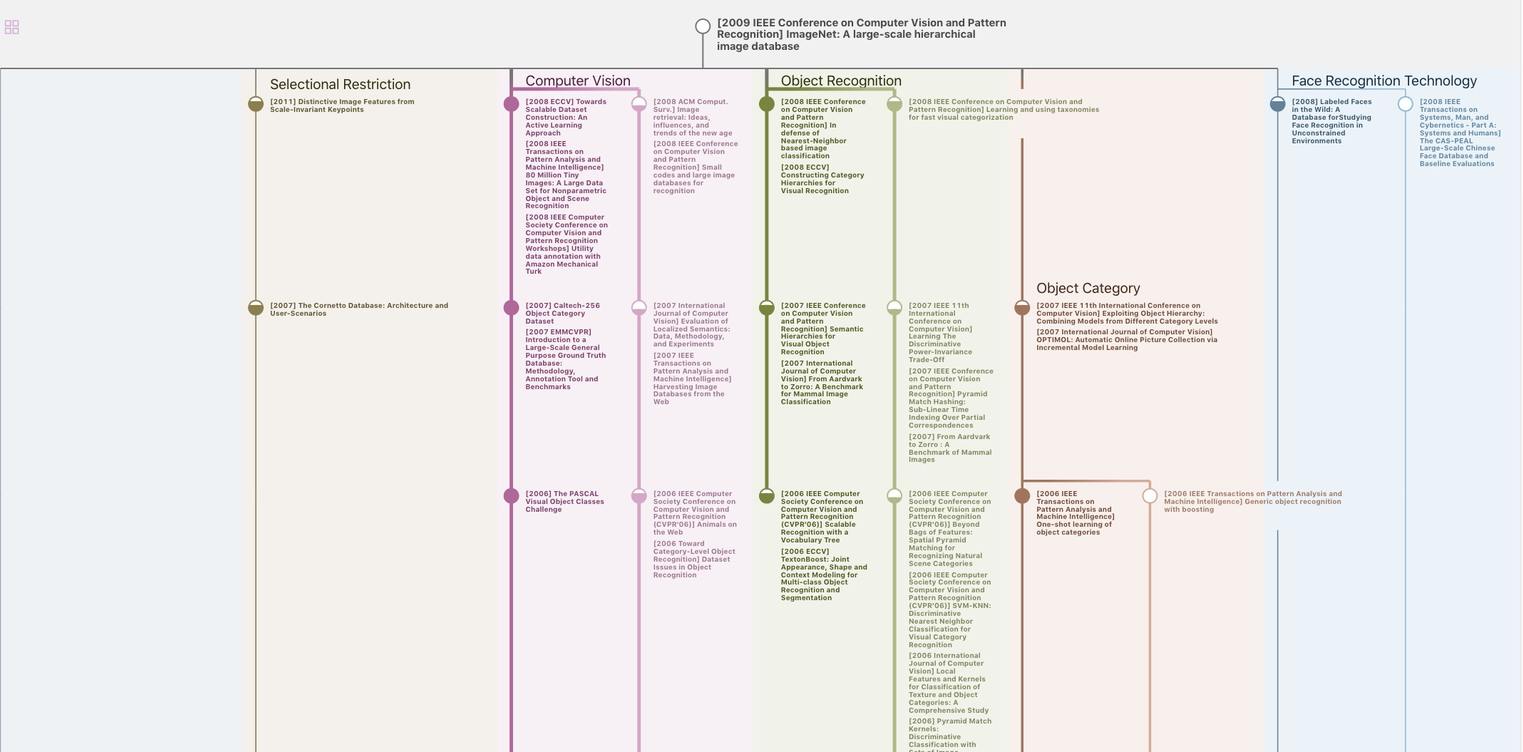
生成溯源树,研究论文发展脉络
Chat Paper
正在生成论文摘要