Multiscale Permutation Entropy: Statistical Characterization On Autoregressive And Moving Average Processes
2019 27TH EUROPEAN SIGNAL PROCESSING CONFERENCE (EUSIPCO)(2019)
摘要
Multiscale Permutation Entropy (MPE), an extension of Permutation Entropy (PE), was proposed to better capture the information content in long range trends. This technique has been extensively used in biomedical applications for diagnosis purposes. Although PE theory is well established and explored, there is still a lack of theoretical development for MPE. In the present paper, we expand the theory by formulating an explicit MPE model of first order Autoregressive (AR) and Moving Average (MA) processes, which are well known and used in signal modeling. We first build the autocorrelation function of coarse grained AR and MA models, which are a prerequisite for MPE calculation. Next, we use the resulting autocorrelation functions to establish the theoretical value of MPE as a function of time scale and AR or MA parameters. The theoretical result is tested against MPE measurements from simulations. We found the MPE of the 1 order AR model to converge to the maximum entropy with increasing time scale. Nonetheless, the convergence is not always monotonic. For AR parameter values greater than the Golden Ratio, the MPE curve presents a local minimum at a time scale different than one, which implies a more regular structure than the one measured with PE. The MPE of the 1 order MA model converges rapidly to the maximum entropy with increasing time scales, regardless of the MA parameter value, which is in accordance to our expectations.
更多查看译文
关键词
Autoregressive model, Moving Average model, Multiscale Permutation Entropy, Coarse-graining procedure
AI 理解论文
溯源树
样例
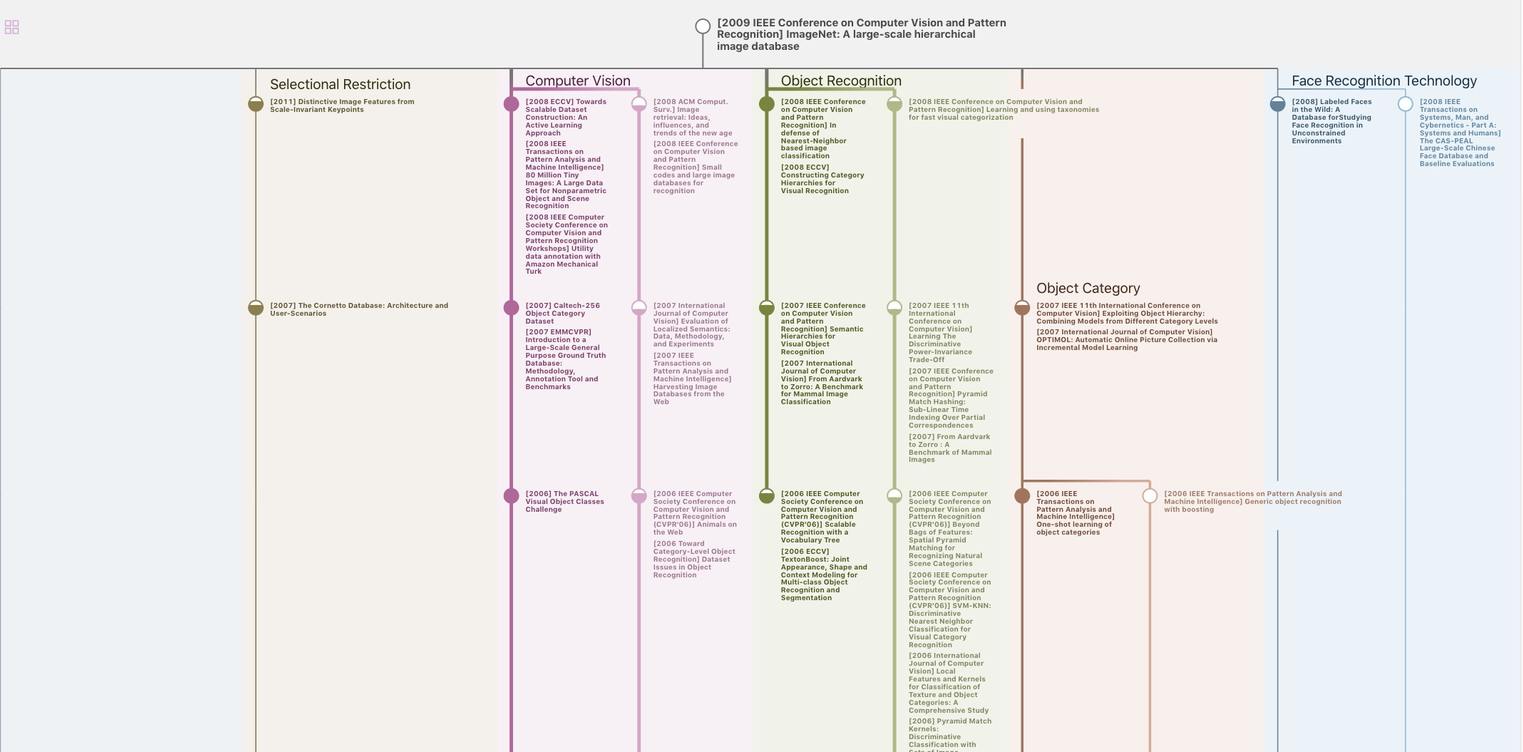
生成溯源树,研究论文发展脉络
Chat Paper
正在生成论文摘要