ScienceExamCER: A High-Density Fine-Grained Science-Domain Corpus for Common Entity Recognition
LREC(2020)
摘要
Named entity recognition identifies common classes of entities in text, but these entity labels are generally sparse, limiting utility to downstream tasks. In this work we present ScienceExamCER, a densely-labeled semantic classification corpus of 133k mentions in the science exam domain where nearly all (96%) of content words have been annotated with one or more fine-grained semantic class labels including taxonomic groups, meronym groups, verb/action groups, properties and values, and synonyms. Semantic class labels are drawn from a manually-constructed fine-grained typology of 601 classes generated through a data-driven analysis of 4,239 science exam questions. We show an off-the-shelf BERT-based named entity recognition model modified for multi-label classification achieves an accuracy of 0.85 F1 on this task, suggesting strong utility for downstream tasks in science domain question answering requiring densely-labeled semantic classification.
更多查看译文
关键词
named entity recognition, corpus, science
AI 理解论文
溯源树
样例
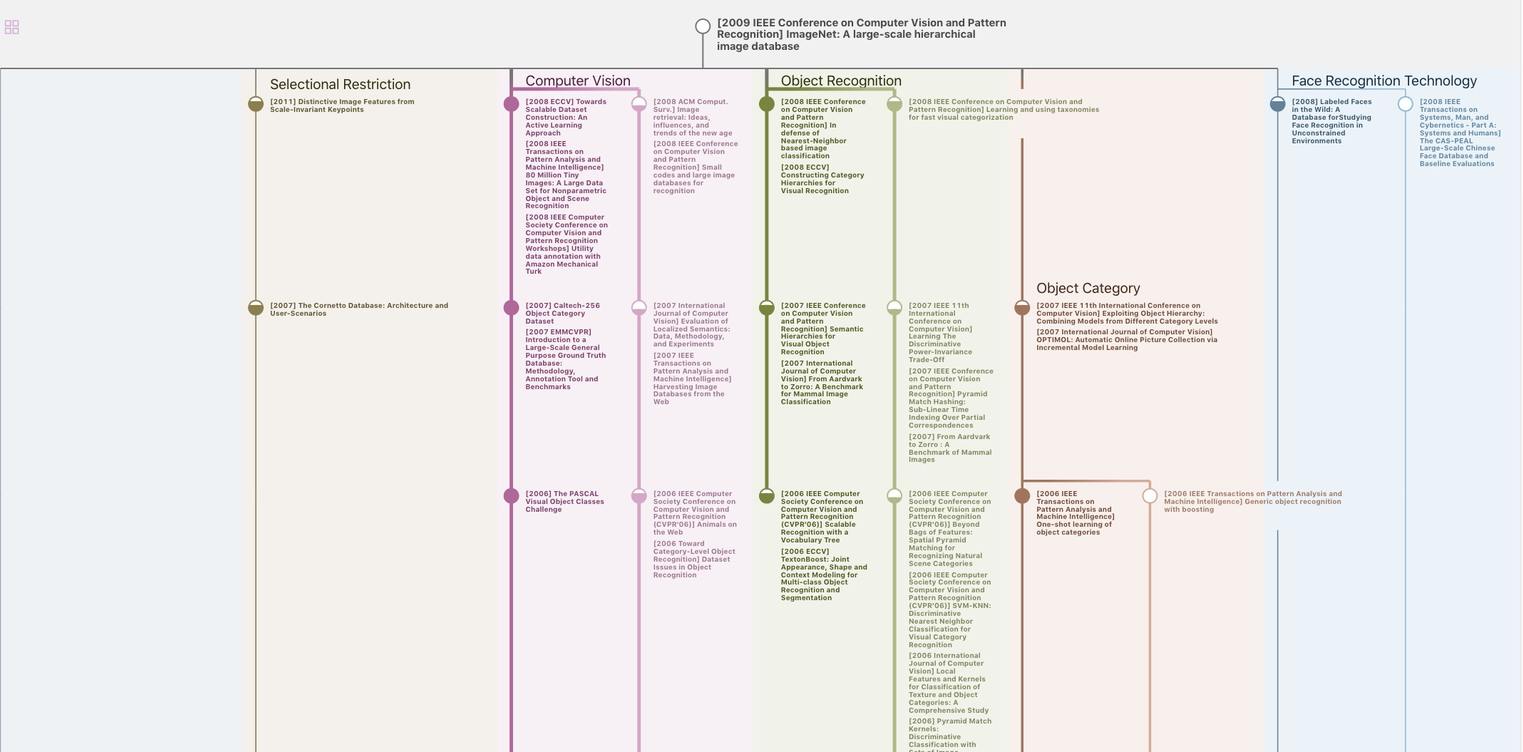
生成溯源树,研究论文发展脉络
Chat Paper
正在生成论文摘要