Financial ratios and stock returns reappraised through a topological data analysis lens
EUROPEAN JOURNAL OF FINANCE(2024)
摘要
Firm financials are well-established predictors of stock returns, being the basis for both the traditional econometric, and growing Machine Learning, asset pricing literature. Employing topological data analysis ball mapper (TDABM), we revisit the association between seven of the most commonly studied financial ratios and stock returns. Upon outlining the methodology to the finance literature, this paper offers three key contributions to the study of asset pricing. Firstly, the characteristic space is visualised to showcase non-monotonic relationships in multiple dimensions that were as yet unseen. Secondly, the means through which neural networks and random forest regressions fit stock returns is also visualised, showing where Machine Learning is contributing to understanding. Finally, an initial application of TDABM for the segmentation of the cross-section is posited, with significant abnormal returns identified. Collectively these three expositions signpost the value of TDABM for financial researchers and practitioners alike. The scope for benefit is limited only by the availability of information to the analyst.
更多查看译文
关键词
Stock returns,anomalies,topological data analysis,data science,mispricing
AI 理解论文
溯源树
样例
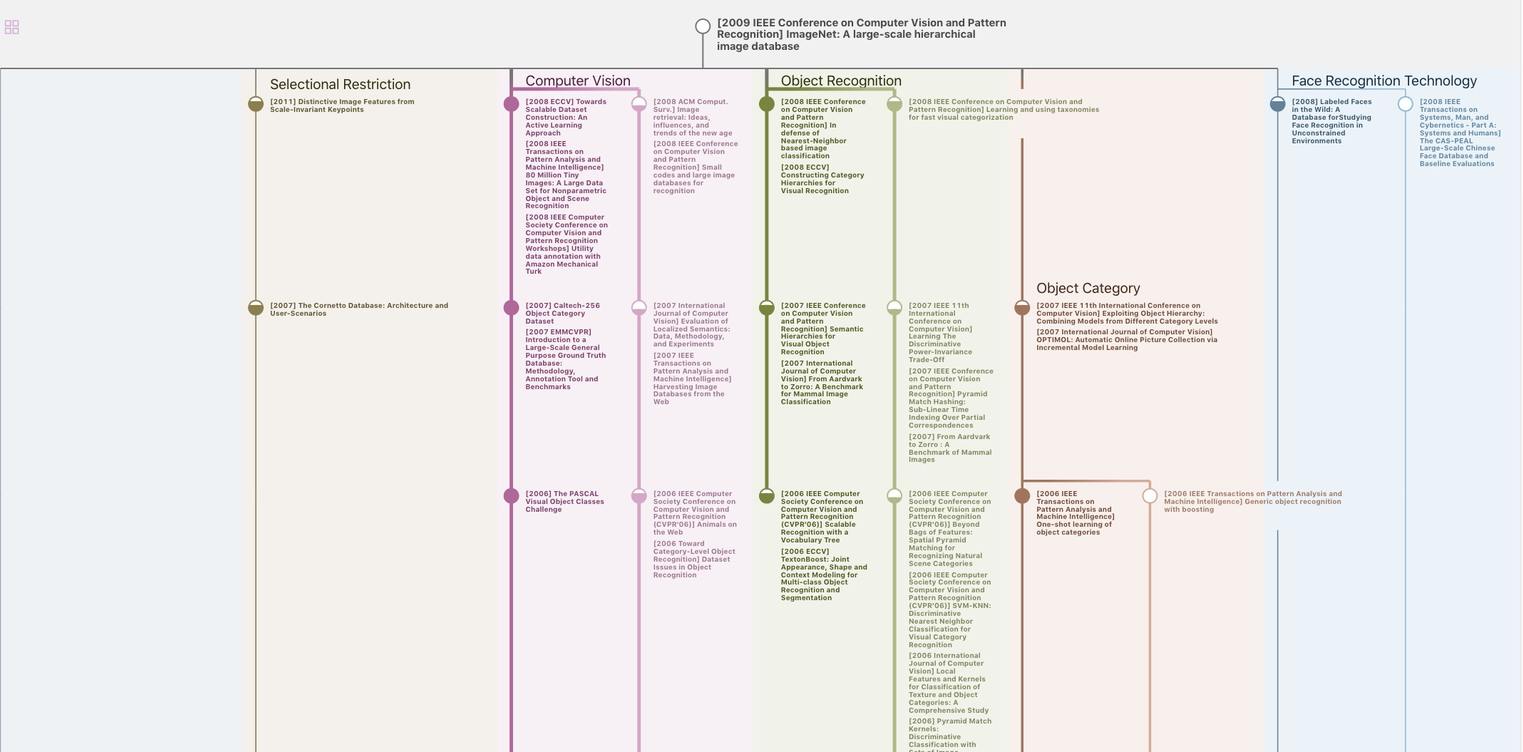
生成溯源树,研究论文发展脉络
Chat Paper
正在生成论文摘要