Blendedmvs: A Large-Scale Dataset For Generalized Multi-View Stereo Networks
2020 IEEE/CVF CONFERENCE ON COMPUTER VISION AND PATTERN RECOGNITION (CVPR)(2020)
摘要
While deep learning has recently achieved great success on multi-view stereo (MVS), limited training data makes the trained model hard to be generalized to unseen scenarios. Compared with other computer vision tasks, it is rather difficult to collect a large-scale MVS dataset as it requires expensive active scanners and labor-intensive process to obtain ground truth 3D structures. In this paper, we introduce BlendedMVS, a novel large-scale dataset, to provide sufficient training ground truth for learning-based MVS. To create the dataset, we apply a 3D reconstruction pipeline to recover high-quality textured meshes from images of well-selected scenes. Then, we render these mesh models to color images and depth maps. To introduce the ambient lighting information during training, the rendered color images are further blended with the input images to generate the training input. Our dataset contains over 17k high-resolution images covering a variety of scenes, including cities, architectures, sculptures and small objects. Extensive experiments demonstrate that BlendedMVS endows the trained model with significantly better generalization ability compared with other MVS datasets. The dataset and pretrained models are available at https://github.com/YoYo000/BlendedMVS.
更多查看译文
关键词
learning-based MVS,3D reconstruction pipeline,ambient lighting information,high-resolution imaging,pretrained models,generalized multiview stereo networks,deep learning,computer vision tasks,large-scale MVS dataset,ground truth 3D structures,color image rendering,high-quality textured mesh models,BlendedMVS
AI 理解论文
溯源树
样例
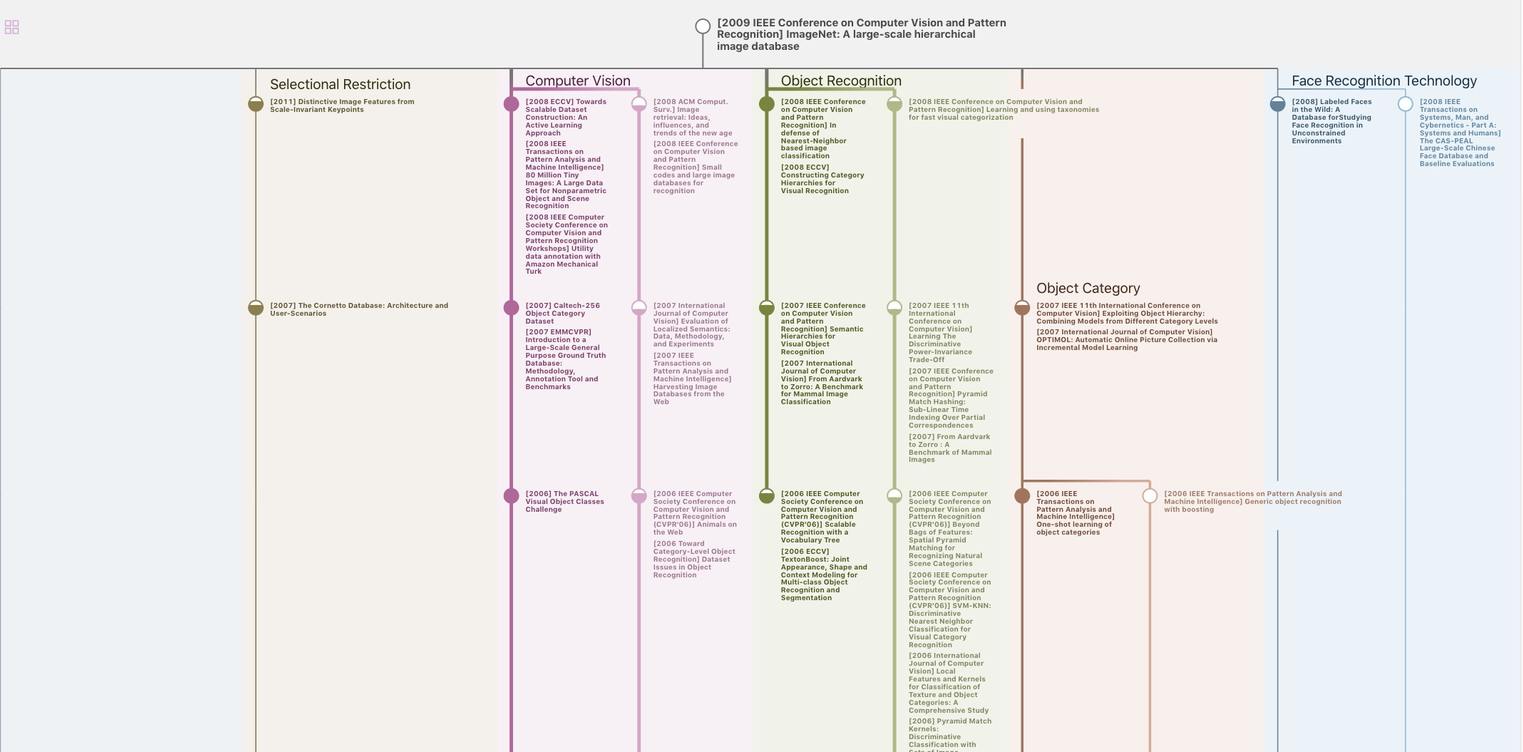
生成溯源树,研究论文发展脉络
Chat Paper
正在生成论文摘要