Continual adaptation for efficient machine communication
CoNLL(2020)
摘要
To communicate with new partners in new contexts, humans rapidly form new linguistic conventions. Recent language models trained with deep neural networks are able to comprehend and produce the existing conventions present in their training data, but are not able to flexibly and interactively adapt those conventions on the fly as humans do. We introduce a repeated reference task as a benchmark for models of adaptation in communication and propose a regularized continual learning framework that allows an artificial agent initialized with a generic language model to more accurately and efficiently communicate with a partner over time. We evaluate this framework through simulations on COCO and in real-time reference game experiments with human partners.
更多查看译文
关键词
continual adaptation,communication,machine
AI 理解论文
溯源树
样例
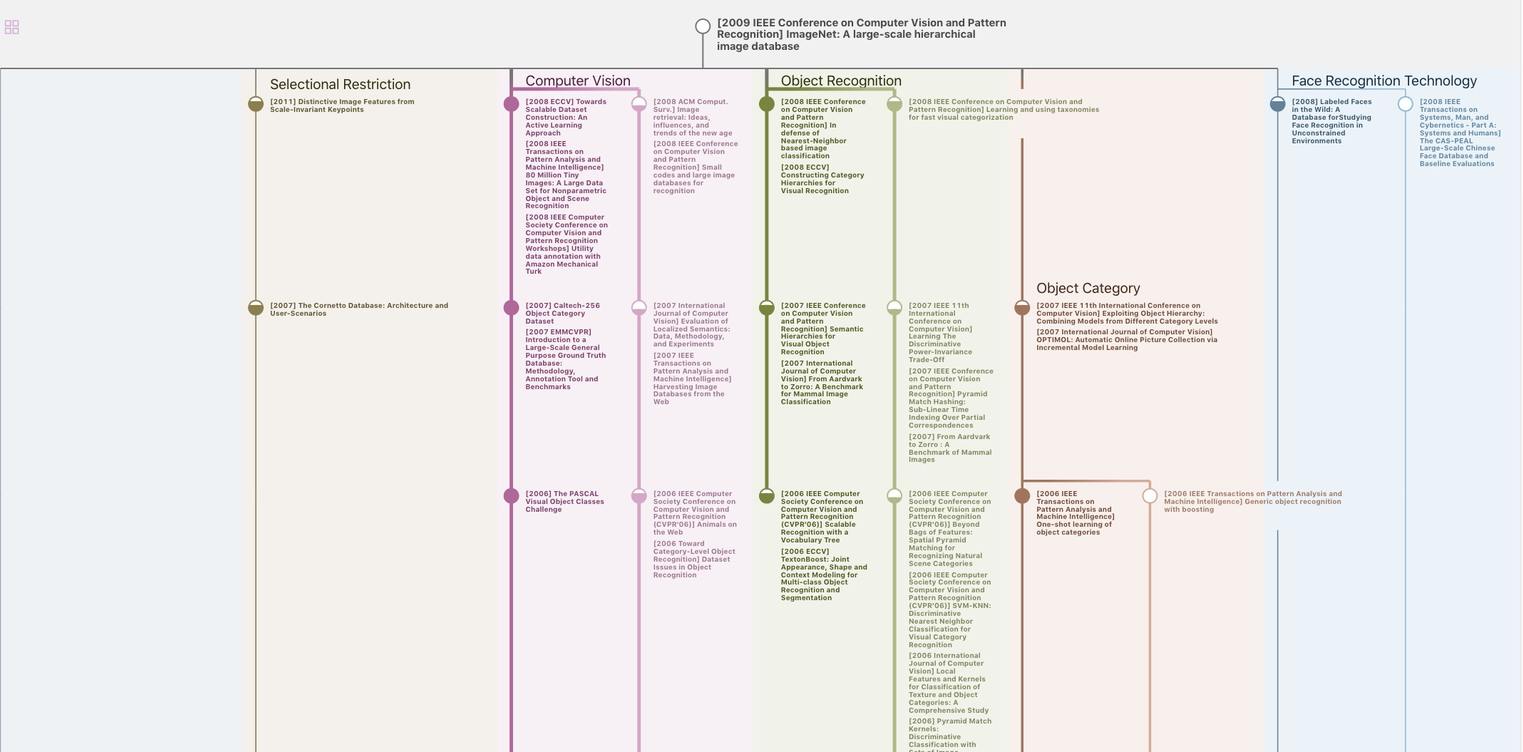
生成溯源树,研究论文发展脉络
Chat Paper
正在生成论文摘要