Controlling False Discovery Rate Using Gaussian Mirrors
Journal of the American Statistical Association(2021)
摘要
Simultaneously finding multiple influential variables and controlling the false discovery rate (FDR) for linear regression models is a fundamental problem. We here propose the Gaussian Mirror (GM) method, which creates for each predictor variable a pair of mirror variables by adding and subtracting a randomly generated Gaussian perturbation, and proceeds with a certain regression method, such as the ordinary least-square or the Lasso (the mirror variables can also be created after selection). The mirror variables naturally lead to test statistics effective for controlling the FDR. Under a mild assumption on the dependence among the covariates, we show that the FDR can be controlled at any designated level asymptotically. We also demonstrate through extensive numerical studies that the GM method is more powerful than many existing methods for selecting relevant variables subject to FDR control, especially for cases when the covariates are highly correlated and the influential variables are not overly sparse.
更多查看译文
关键词
False discovery rate,Genome-wide association study,High-dimensional linear regression,Post-selection inference
AI 理解论文
溯源树
样例
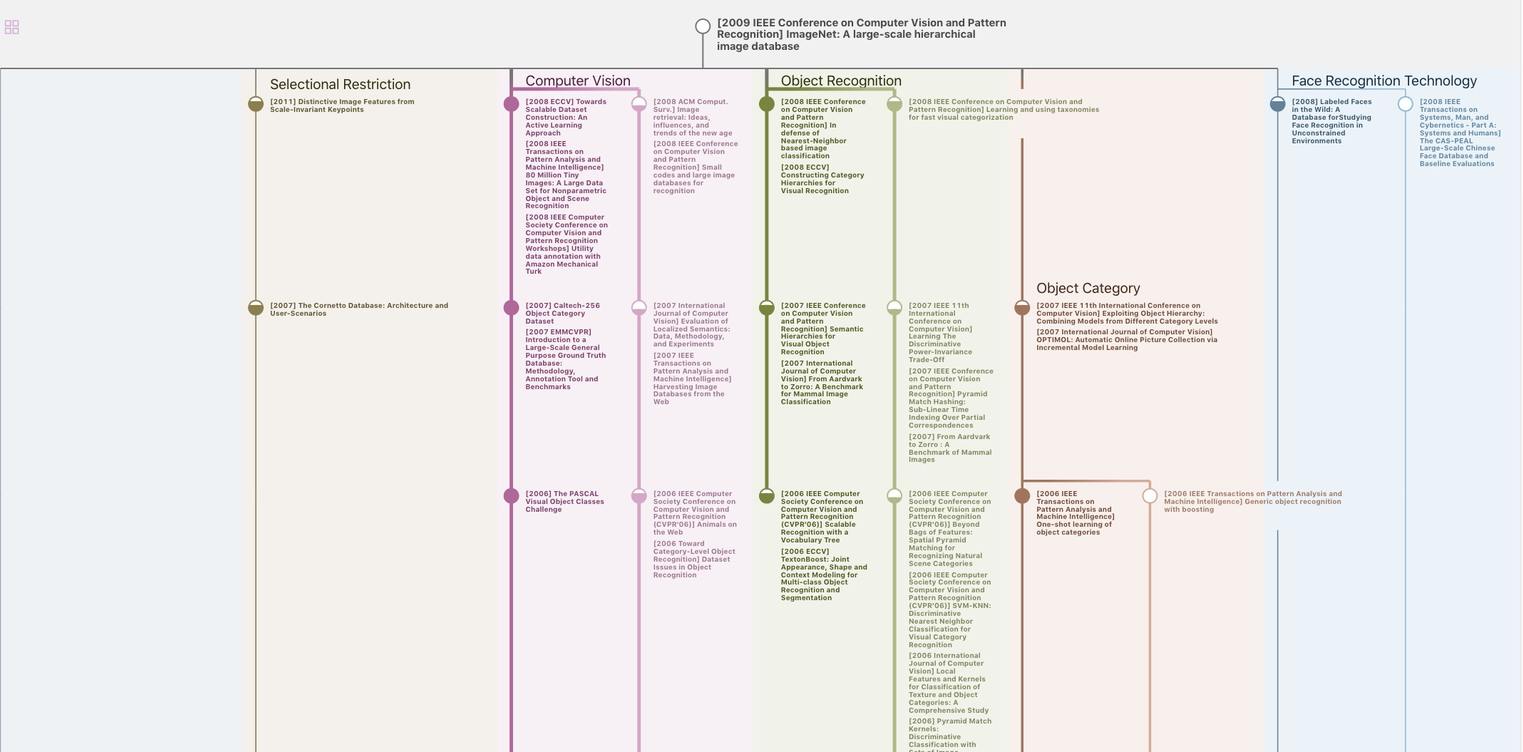
生成溯源树,研究论文发展脉络
Chat Paper
正在生成论文摘要