Epistemic Sets Applied to Best-of-n Problems.
ECSQARU(2019)
摘要
Epistemic sets are a simple and efficient way of representing uncertain beliefs in AI, in which an agent identifies those states or worlds that she deems to be possible. We investigate their application to multi-agent distributed learning and decision making, in particular to best-of-n problems in which a population of agents must reach a consensus by identifying the best out of n possible alternatives or choices, each of different quality. We show that, despite their limited representational power, epistemic sets can be effectively deployed by agents engaged in a learning process in which they receive evidence directly from the environment and also pool or fuse their beliefs with those of other agents, in order to solve a best-of-n problem. We describe an analytical model of such a system based on ordinary differential equations and conduct a fixed point analysis so as to obtain insights into macro-level convergence properties. We then conduct a series of agent-based simulation experiments to investigate the robustness of the epistemic set approach. The results suggest that when applied to best-of-n problems epistemic sets are robust to noise and scalable to large state spaces, even when the population size is relatively small. This in turn supports the claim that they have potential applications in decentralised AI and swarm robotics at a range of different scales.
更多查看译文
关键词
problems,best-of-n
AI 理解论文
溯源树
样例
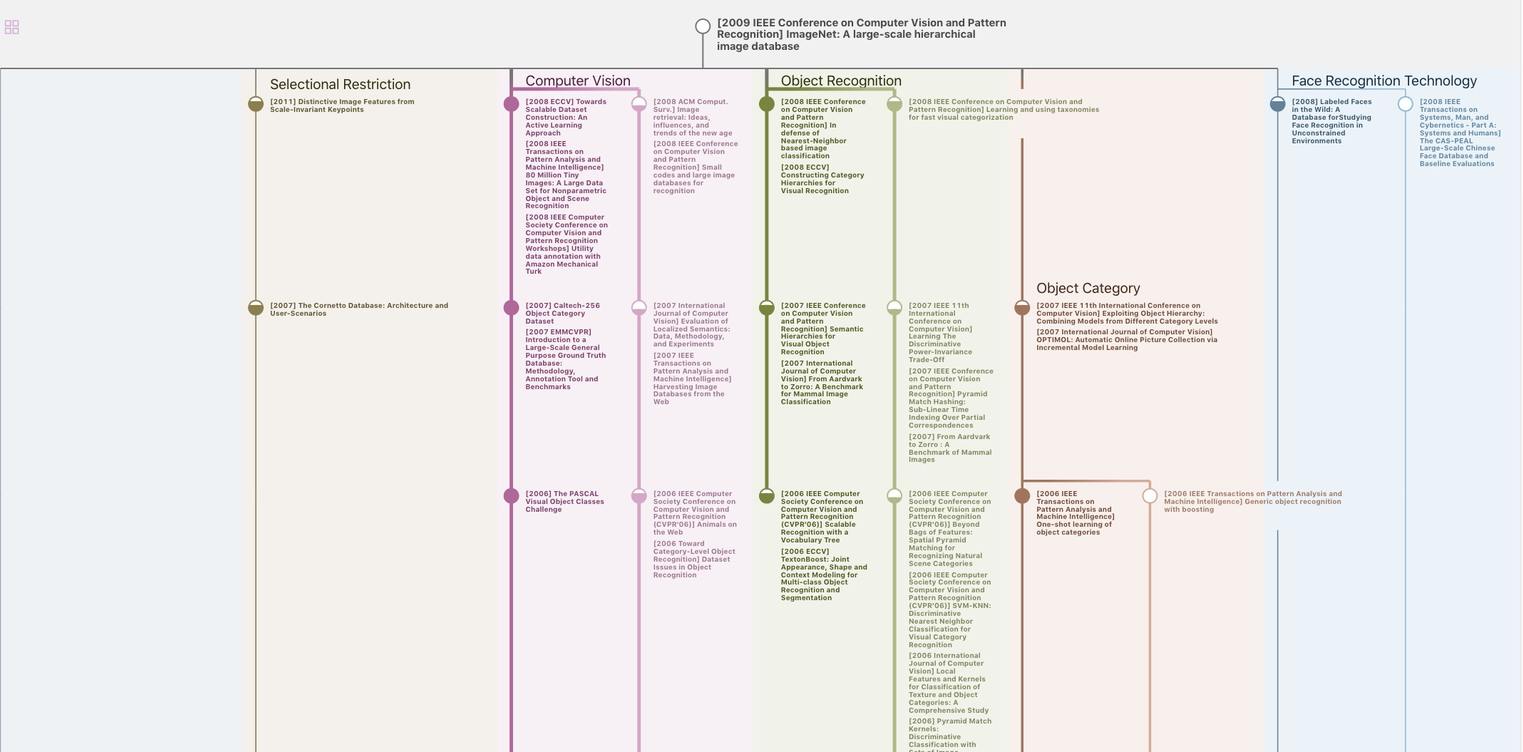
生成溯源树,研究论文发展脉络
Chat Paper
正在生成论文摘要