Superpixel-Guided Sparse Unmixing For Remotely Sensed Hyperspectral Imagery
2019 IEEE INTERNATIONAL GEOSCIENCE AND REMOTE SENSING SYMPOSIUM (IGARSS 2019)(2019)
摘要
Sparse representation-based approaches have been successfully applied to remotely sensed hyperspectral image unmixing. In recent years, sparse unmixing techniques have incorporated spatial information into the sparse unmixing model, achieving improved fractional abundance results. Most spatial-based sparse unmixing methods utilize regular-shaped neighborhoods (e.g., a cross or a square window) to characterize the spatial-contextual information around each pixel. However, the spatial characteristics of natural scenes are not always uniform, but vary according to the observed objects. Therefore, assuming uniform spatial neighborhoods may not be consistent with real spatial structures in the scene. Super-pixels offer a good solution to this problem since they can better characterize such spatial structures. Based on this observation, in this paper we develop a new superpixel-guided sparse unmixing (SPGSU) method for hyperspectral scenes. The proposed SPGSU includes the spatial correlation through a superpixel-based technique rather than assuming predefined pixel grids. Each superpixel can be regarded as a small spatial region, whose shape and size can be adaptively changed to accommodate different spatial structures. Our experimental results, conducted using simulated data sets, quantitatively indicate that our newly proposed method produces better results than other advanced spectral unmixing methods.
更多查看译文
关键词
Hyperspectral imaging, spatial-based sparse unmixing, superpixels, spatially-weighted unmixing
AI 理解论文
溯源树
样例
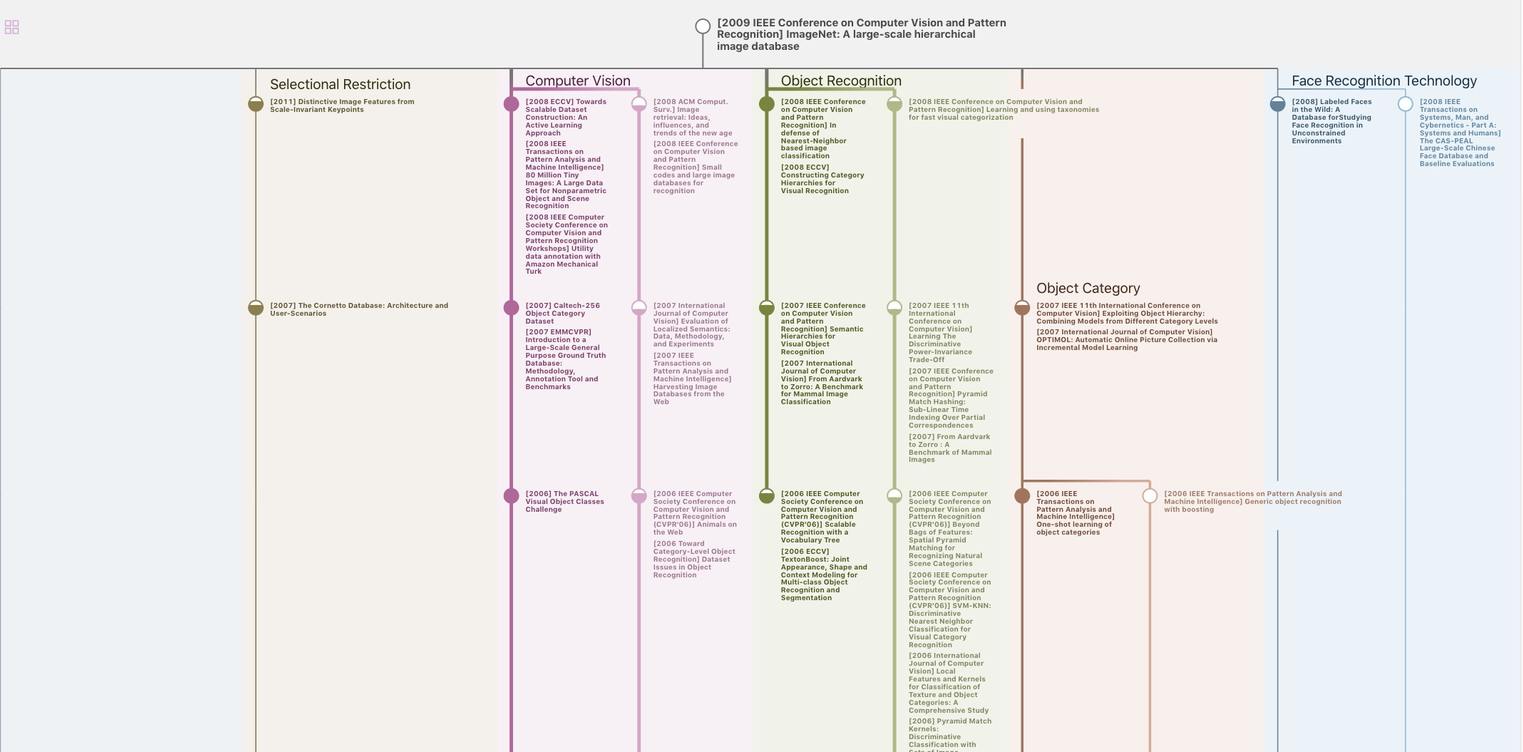
生成溯源树,研究论文发展脉络
Chat Paper
正在生成论文摘要