Deep Feature Extraction Based On Siamese Network And Auto-Encoder For Hyperspectral Image Classification
2019 IEEE INTERNATIONAL GEOSCIENCE AND REMOTE SENSING SYMPOSIUM (IGARSS 2019)(2019)
摘要
Hyperspectral image classification with limited training samples has become a hot research topic recently. Though deep convolution neural network shows powerful ability for feature extraction, its good performance often relies on sufficient training data. In this paper, we propose a multi-task learning framework based on siamese network and auto-encoder to fully exploit limited labeled samples' information and obtain discriminative features for classification of hyperspectral images. A low intraclass and high interclass variability of features can be learned by metric learning using our framework. And superpixel-based 3D sample preprocessing is applied to improve the classification accuracy on the hyperspectral images' boundaries. The experimental results demonstrate that our framework can achieve competitive results compared with the state-of-the-art methods.
更多查看译文
关键词
Siamese network, auto-encoder, deep feature extraction, superpixel, hyperspectral image classification
AI 理解论文
溯源树
样例
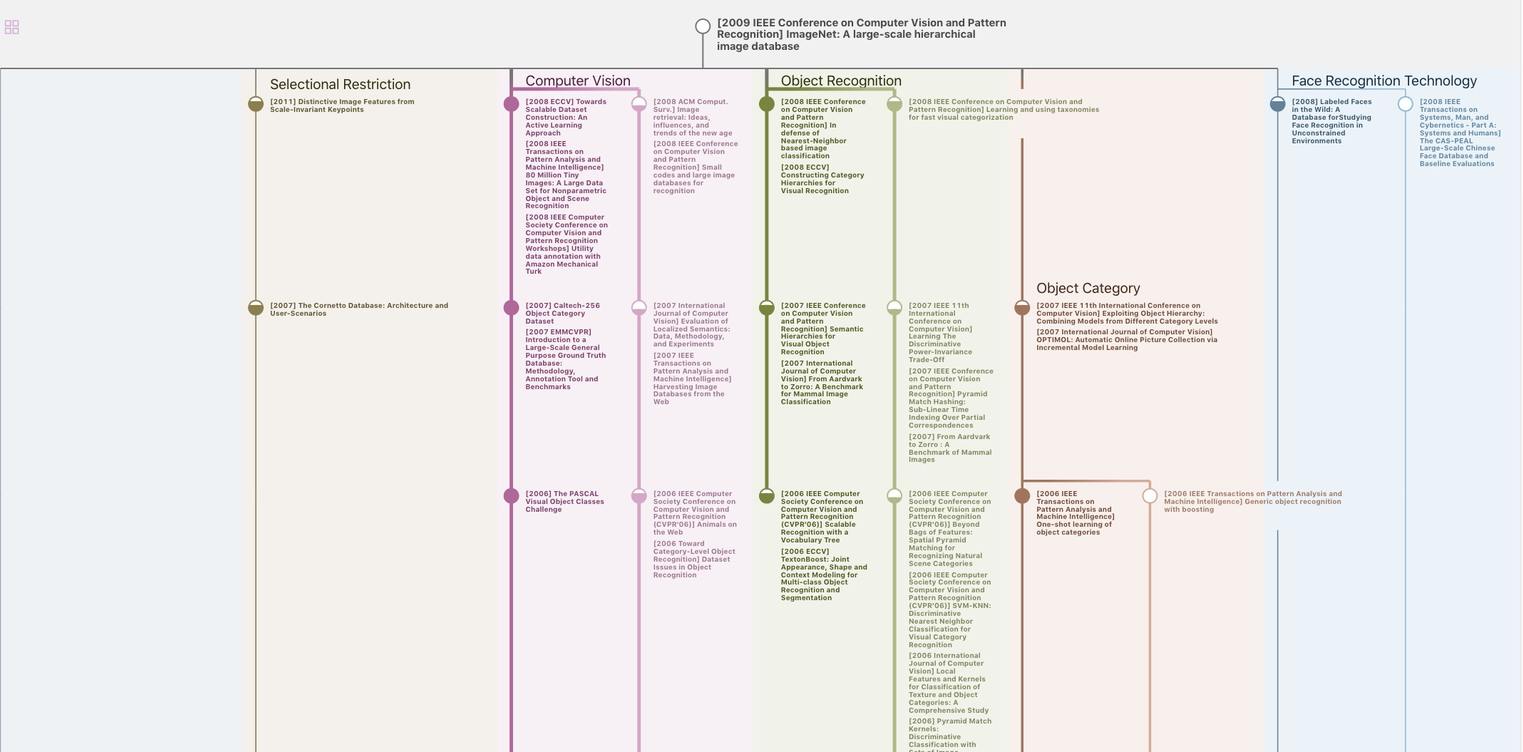
生成溯源树,研究论文发展脉络
Chat Paper
正在生成论文摘要