Deep Spectral Super-Resolution With Noisy Input
2019 IEEE INTERNATIONAL GEOSCIENCE AND REMOTE SENSING SYMPOSIUM (IGARSS 2019)(2019)
摘要
Learning based methods, e.g., sparse coding or deep convolutional neural networks (DCNNs) have underpinned much of recent progress in increasing the spectral resolution of an RGB image for hyperspectral image (HSI) super-resolution. However, these methods suffer severe performance loss, when the test RGB image distributed differently from the training set, e.g., being corrupted with random noise. To mitigate this problem, we propose an unsupervised deep spectral super-resolution method, which employs a DCNN to generate the latent HSI from an input RGB and encourages it to fit the input RGB image through down-sampling in spectral domain as well as a sparse gradient prior in spatial domain. Due to the powerful capacity of DCNN in capturing the low-level image statistics, the proposed method is able to automatically accommodate the noise corruption in the input RGB image. Experimental results shows the superior performance of the proposed method.
更多查看译文
关键词
Spectral super-resolution, unsupervised learning, deep convolutional neural networks
AI 理解论文
溯源树
样例
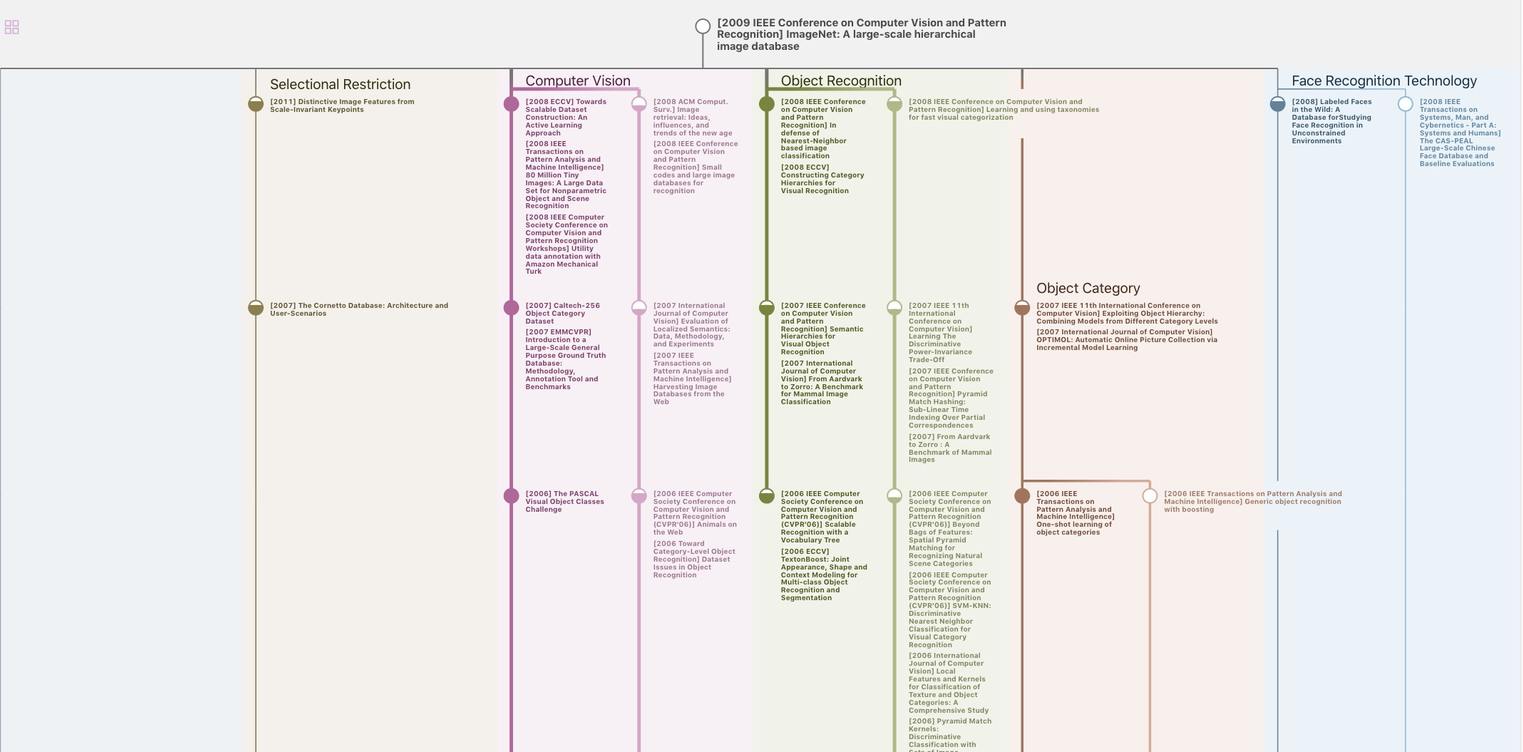
生成溯源树,研究论文发展脉络
Chat Paper
正在生成论文摘要