Interactive Supervision with t-SNE.
K-CAP(2019)
摘要
Knowledge capture from human experts in domain-specific settings can benefit from incisive use of machine intelligence to reduce expended time and effort. Such a capability can be of significant value to deep learning, given its demand for large labeled data. We propose an ML-based system for interactive labeling of image datasets to speed up class attribution performed by domain experts. The tool visualizes feature spaces and makes it directly editable through online integration of applied labels. We propose realistic annotation emulation to evaluate the system design of interactive active learning, based on our improved semi-supervised extension of t-SNE dimensionality reduction. We contribute globally normalized attractions, semi-supervised repulsion, smoothed label integration, and parameter optimization in our improved t-SNE. Our active learning tool can significantly increase labeling efficiency compared to uncertainty sampling, and we show that less than 100 labeling actions are typically sufficient for good classification on a variety of specialized image datasets. Our contribution is unique given that it needs to perform dimensionality reduction, feature space visualization and editing, interactive label propagation, low-complexity active learning, human perceptual modeling, annotation emulation and unsupervised feature extraction for specialized datasets in a production-quality implementation.
更多查看译文
关键词
semi-supervised learning, active learning, dimensionality reduction
AI 理解论文
溯源树
样例
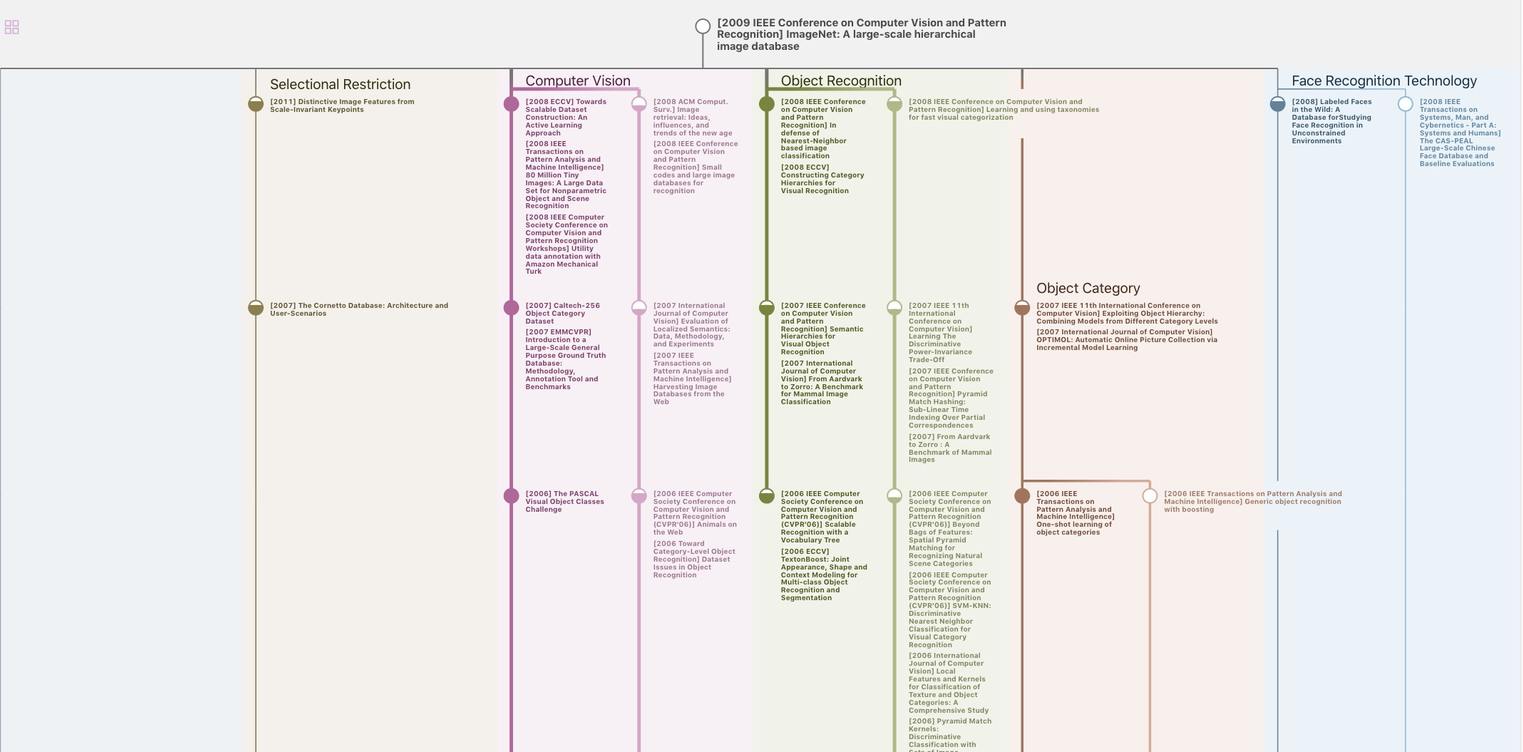
生成溯源树,研究论文发展脉络
Chat Paper
正在生成论文摘要