Global metabolic profiling to model biological processes of aging in twins.
AGING CELL(2020)
摘要
Aging is intimately linked to system-wide metabolic changes that can be captured in blood. Understanding biological processes of aging in humans could help maintain a healthy aging trajectory and promote longevity. We performed untargeted plasma metabolomics quantifying 770 metabolites on a cross-sectional cohort of 268 healthy individuals including 125 twin pairs covering human lifespan (from 6 months to 82 years). Unsupervised clustering of metabolic profiles revealed 6 main aging trajectories throughout life that were associated with key metabolic pathways such as progestin steroids, xanthine metabolism, and long-chain fatty acids. A random forest (RF) model was successful to predict age in adult subjects (>= 16 years) using 52 metabolites (R-2 = .97). Another RF model selected 54 metabolites to classify pediatric and adult participants (out-of-bag error = 8.58%). These RF models in combination with correlation network analysis were used to explore biological processes of healthy aging. The models highlighted established metabolites, like steroids, amino acids, and free fatty acids as well as novel metabolites and pathways. Finally, we show that metabolic profiles of twins become more dissimilar with age which provides insights into nongenetic age-related variability in metabolic profiles in response to environmental exposure.
更多查看译文
关键词
aging,LC-MS,machine learning,metabolomics,random forest,twins
AI 理解论文
溯源树
样例
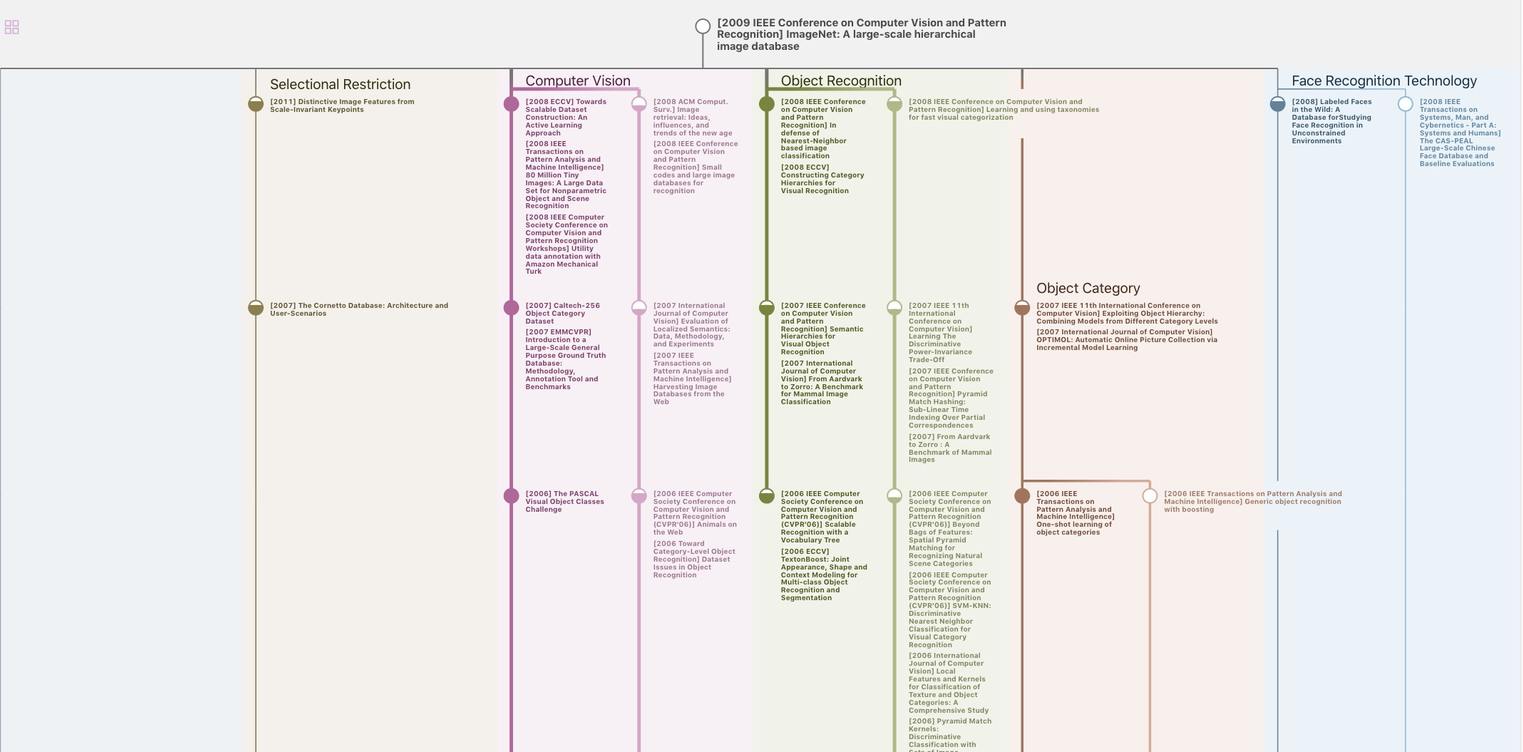
生成溯源树,研究论文发展脉络
Chat Paper
正在生成论文摘要