Combining Dispersion Modeling and Monitoring Data for Community-Scale Air Quality Characterization.
ATMOSPHERE(2019)
摘要
Spatially and temporally resolved air quality characterization is critical for community-scale exposure studies and for developing future air quality mitigation strategies. Monitoring-based assessments can characterize local air quality when enough monitors are deployed. However, modeling plays a vital role in furthering the understanding of the relative contributions of emissions sources impacting the community. In this study, we combine dispersion modeling and measurements from the Kansas City TRansportation local-scale Air Quality Study (KC-TRAQS) and use data fusion methods to characterize air quality. The KC-TRAQS study produced a rich dataset using both traditional and emerging measurement technologies. We used dispersion modeling to support field study design and analysis. In the study design phase, the presumptive placement of fixed monitoring sites and mobile monitoring routes have been corroborated using a research screening tool C-PORT to assess the spatial and temporal coverage relative to the entire study area extent. In the analysis phase, dispersion modeling was used in combination with observations to help interpret the KC-TRAQS data. We extended this work to use data fusion methods to combine observations from stationary, mobile measurements, and dispersion model estimates.
更多查看译文
关键词
near-source,dispersion modeling,rail yard,air pollution
AI 理解论文
溯源树
样例
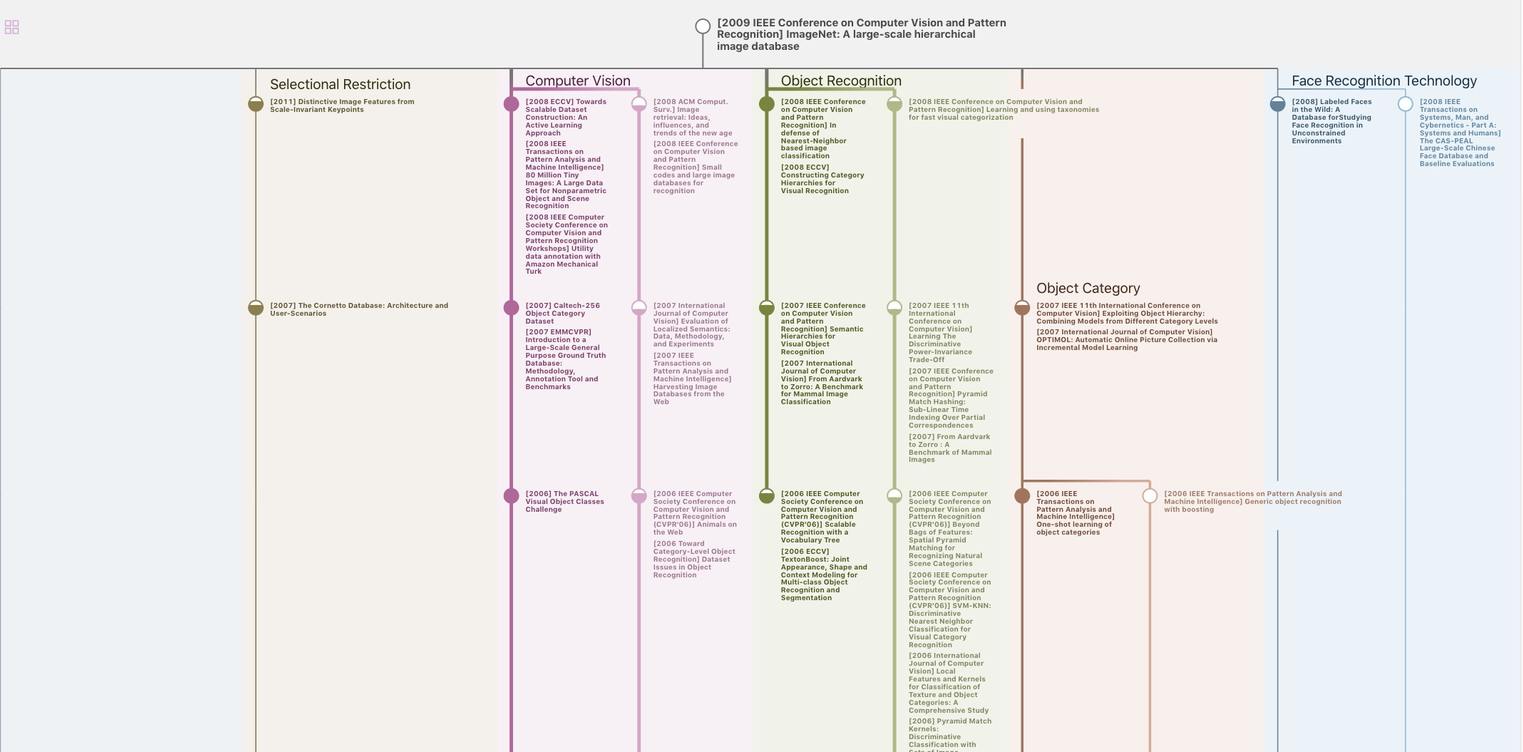
生成溯源树,研究论文发展脉络
Chat Paper
正在生成论文摘要