Fairness through Equality of Effort
WWW '20: The Web Conference 2020 Taipei Taiwan April, 2020(2020)
摘要
Fair machine learning is receiving an increasing attention in machine learning fields. Researchers in fair learning have developed correlation or association-based measures such as demographic disparity, mistreatment disparity, calibration, causal-based measures such as total effect, direct and indirect discrimination, and counterfactual fairness, and fairness notions such as equality of opportunity and equalized odds that consider both decisions in the training data and decisions made by predictive models. In this paper, we develop a new causal-based fairness notation, called equality of effort. Different from existing fairness notions which mainly focus on discovering the disparity of decisions between two groups of individuals, the proposed equality of effort notation helps answer questions like to what extend a legitimate variable should change to make a particular individual achieve a certain outcome level and addresses the concerns whether the efforts made to achieve the same outcome level for individuals from the protected group and that from the unprotected group are different. We develop algorithms for determining whether an individual or a group of individuals is discriminated in terms of equality of effort. We also develop an optimization-based method for removing discriminatory effects from the data if discrimination is detected. We conduct empirical evaluations to compare the equality of effort and existing fairness notion and show the effectiveness of our proposed algorithms.
更多查看译文
关键词
Fairness, Equality of Effort, Causality
AI 理解论文
溯源树
样例
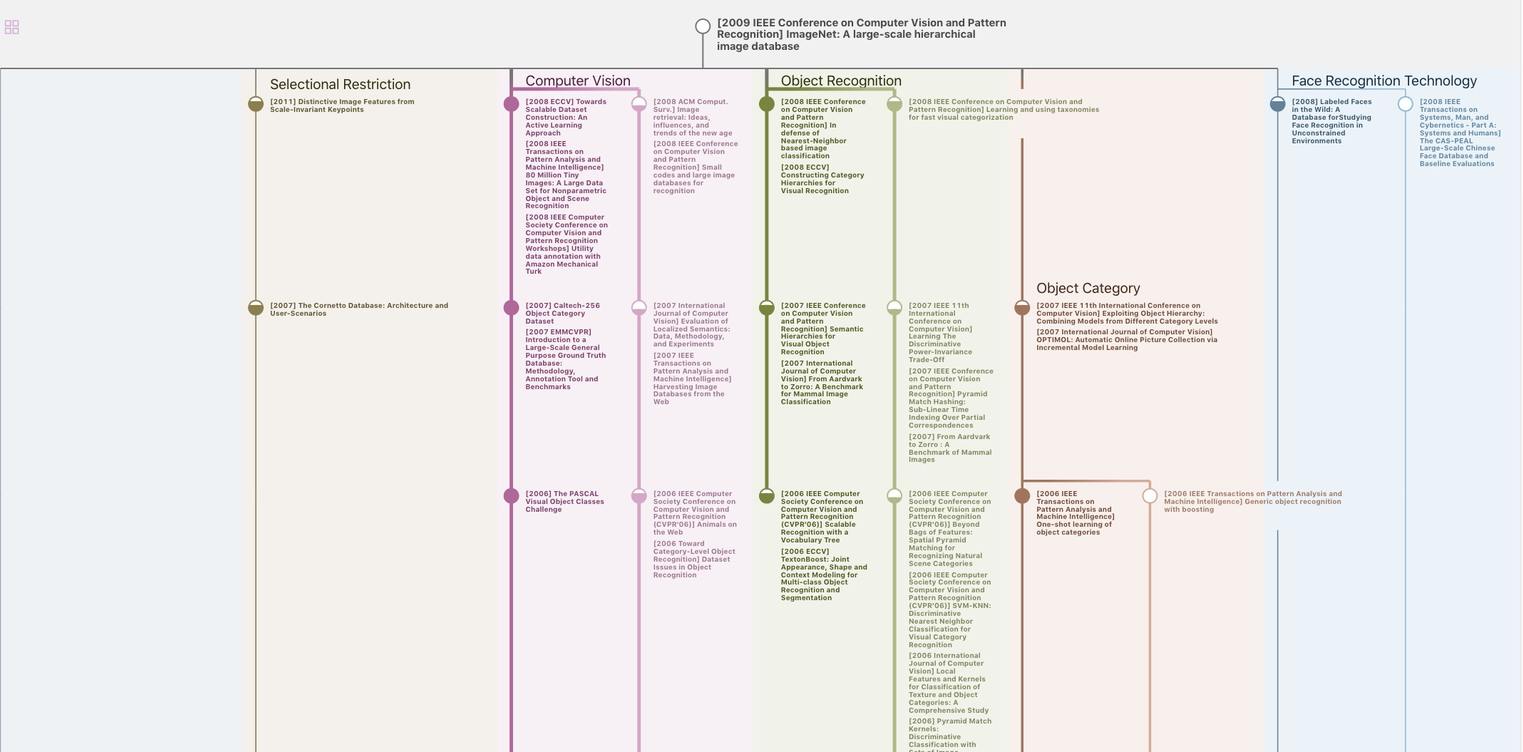
生成溯源树,研究论文发展脉络
Chat Paper
正在生成论文摘要