Mimic The Raw Domain: Accelerating Action Recognition in the Compressed Domain
CVPR Workshops(2020)
摘要
Video understanding usually requires expensive computation that prohibits its deployment, yet videos contain significant spatiotemporal redundancy that can be exploited. In particular, operating directly on the motion vectors and residuals in the compressed video domain can significantly accelerate the compute, by not using the raw videos which demand colossal storage capacity. Existing methods approach this task as a multiple modalities problem. In this paper we are approaching the task in a completely different way; we are looking at the data from the compressed stream as a one unit clip and propose that the residual frames can replace the original RGB frames from the raw domain. Furthermore, we are using teacher-student method to aid the network in the compressed domain to mimic the teacher network in the raw domain. We show experiments on three leading datasets (HMDB51, UCF1, and Kinetics) that approach state-of-the-art accuracy on raw video data by using compressed data. Our model MFCD-Net outperforms prior methods in the compressed domain and more importantly, our model has 11X fewer parameters and 3X fewer Flops, dramatically improving the efficiency of video recognition inference. This approach enables applying neural networks exclusively in the compressed domain without compromising accuracy while accelerating performance.
更多查看译文
关键词
compressed video domain,compressed stream,raw domain,compressed domain,raw video data,compressed data,video recognition inference,action recognition,video understanding
AI 理解论文
溯源树
样例
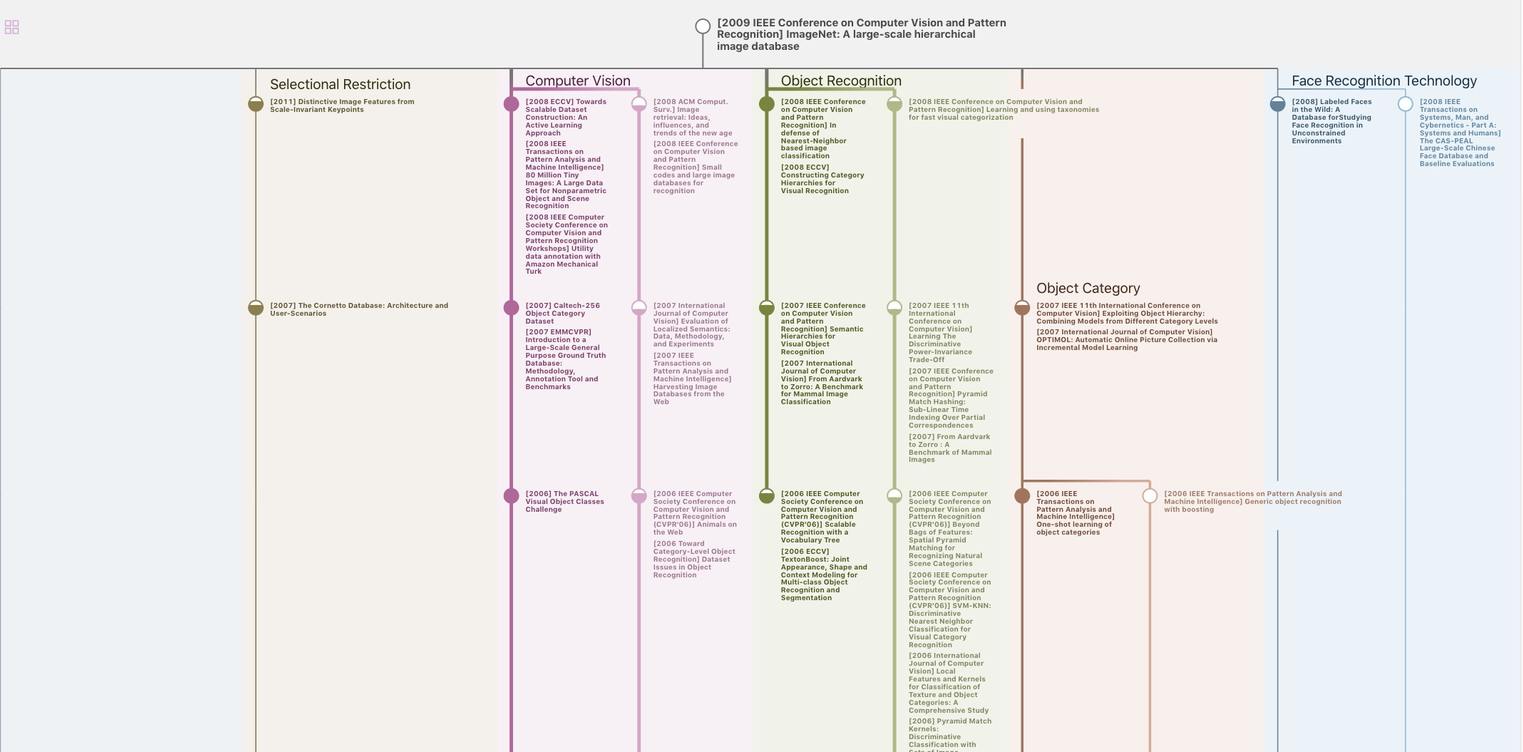
生成溯源树,研究论文发展脉络
Chat Paper
正在生成论文摘要