Fine-Grained Neural Architecture Search
arxiv(2019)
摘要
We present an elegant framework of fine-grained neural architecture search (FGNAS), which allows to employ multiple heterogeneous operations within a single layer and can even generate compositional feature maps using several different base operations. FGNAS runs efficiently in spite of significantly large search space compared to other methods because it trains networks end-to-end by a stochastic gradient descent method. Moreover, the proposed framework allows to optimize the network under predefined resource constraints in terms of number of parameters, FLOPs and latency. FGNAS has been applied to two crucial applications in resource demanding computer vision tasks---large-scale image classification and image super-resolution---and demonstrates the state-of-the-art performance through flexible operation search and channel pruning.
更多查看译文
AI 理解论文
溯源树
样例
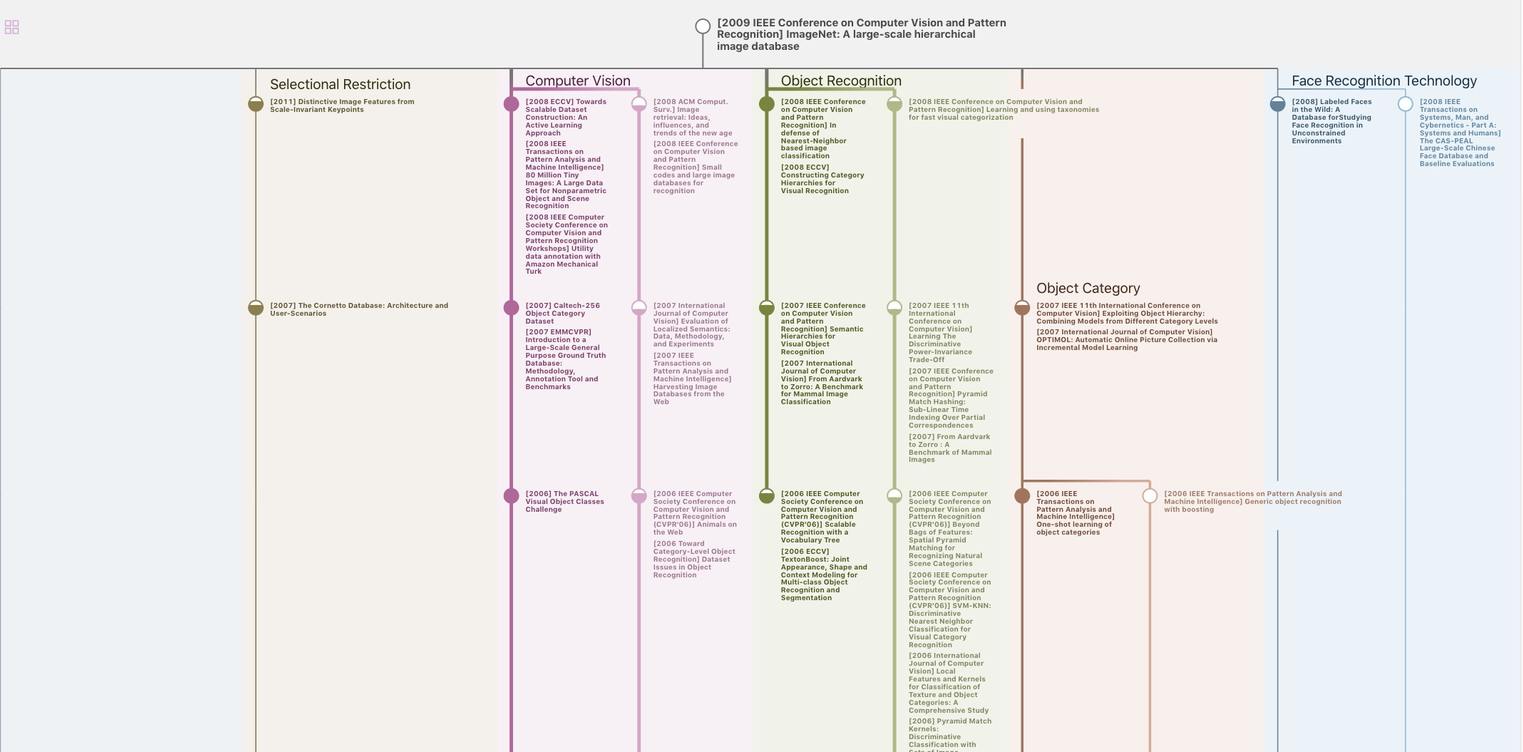
生成溯源树,研究论文发展脉络
Chat Paper
正在生成论文摘要