Potential Field: Interpretable and Unified Representation for Trajectory Prediction
arxiv(2019)
摘要
Predicting an agent's future trajectory is a challenging task given the complicated stimuli (environmental/inertial/social) of motion. Prior works learn individual stimulus from different modules and fuse the representations in an end-to-end manner, which makes it hard to understand what are actually captured and how they are fused. In this work, we borrow the notion of potential field from physics as an interpretable and unified representation to model all stimuli. This allows us to not only supervise the intermediate learning process, but also have a coherent method to fuse the information of different sources. From the generated potential fields, we further estimate future motion direction and speed, which are modeled as Gaussian distributions to account for the multi-modal nature of the problem. The final prediction results are generated by recurrently moving past location based on the estimated motion direction and speed. We show state-of-the-art results on the ETH, UCY, and Stanford Drone datasets.
更多查看译文
AI 理解论文
溯源树
样例
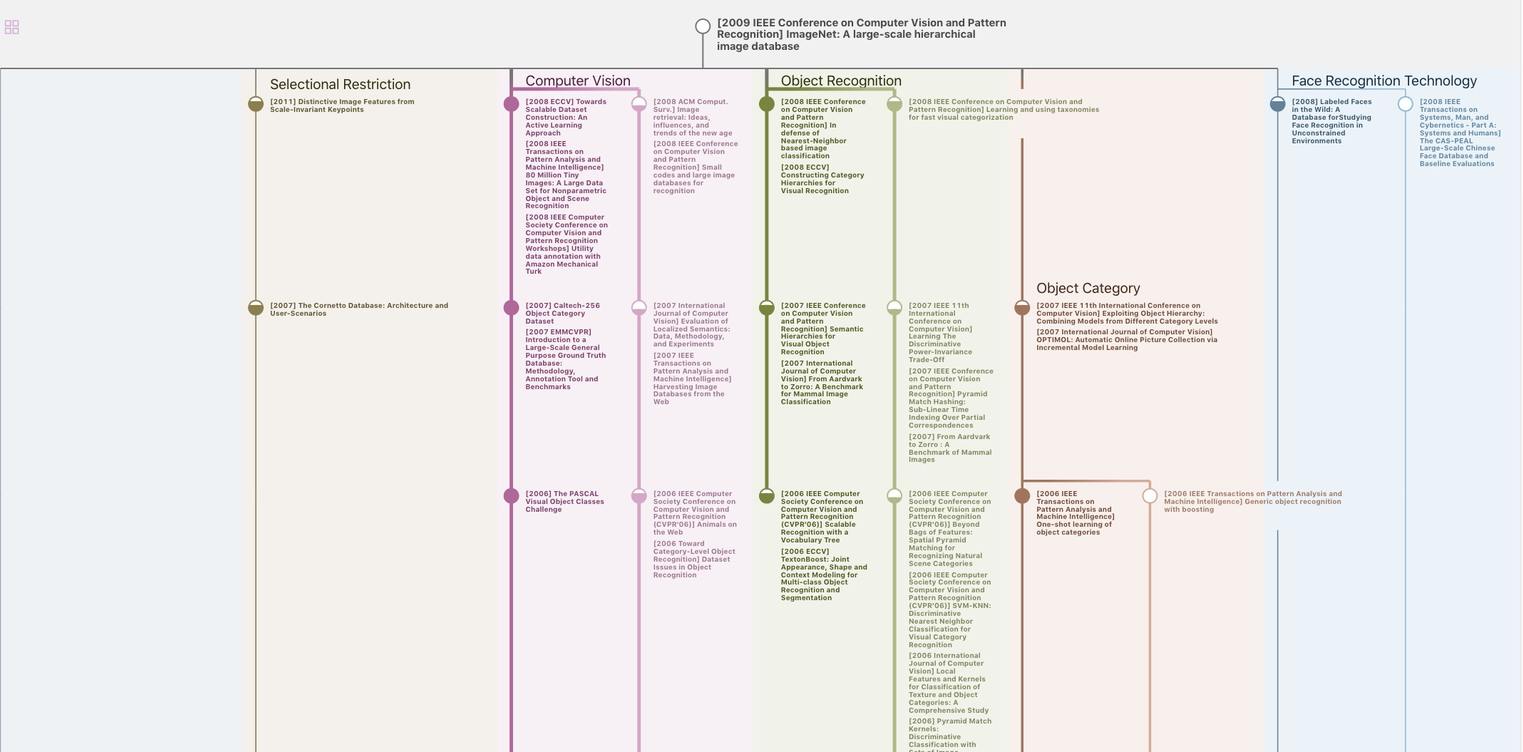
生成溯源树,研究论文发展脉络
Chat Paper
正在生成论文摘要