An Application of Random Walk Resampling to Phylogenetic HMM Inference and Learning
IEEE Transactions on NanoBioscience(2019)
摘要
Statistical resampling methods are widely used for confidence interval placement and as a data perturbation technique for statistical inference and learning. An important assumption of popular resampling methods such as the standard bootstrap is that input observations are identically and independently distributed (i.i.d.). However, within the area of computational biology and bioinformatics, many different factors can contribute to intra-sequence dependence, such as recombination and other evolutionary processes governing sequence evolution. The SEquential RESampling (“SERES”) framework was previously proposed to relax the simplifying assumption of i.i.d. input observations. SERES resampling takes the form of random walks on an input of either aligned or unaligned biomolecular sequences. This study introduces the first application of SERES random walks on aligned sequence inputs and is also the first to demonstrate the utility of SERES as a data perturbation technique to yield improved statistical estimates. We focus on the classical problem of recombination-aware local genealogical inference. We show in a simulation study that coupling SERES resampling and re-estimation with recHMM, a hidden Markov model-based method, produces local genealogical inferences with consistent and often large improvements in terms of topological accuracy. We further evaluate method performance using empirical HIV genome sequence datasets.
更多查看译文
关键词
Hidden Markov models,Computational modeling,Phylogeny,Bioinformatics,Biological system modeling,Inference algorithms,Perturbation methods
AI 理解论文
溯源树
样例
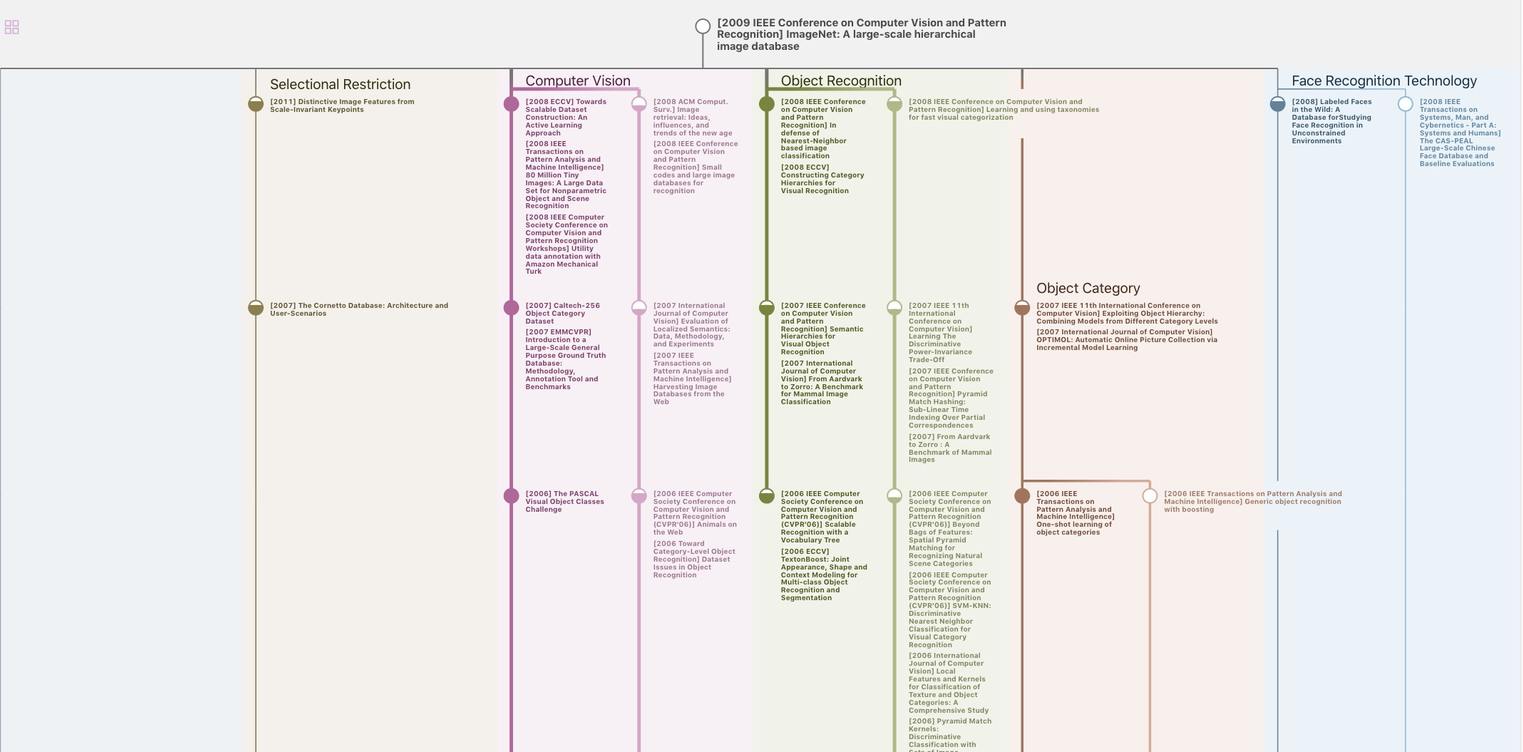
生成溯源树,研究论文发展脉络
Chat Paper
正在生成论文摘要