TITAN: A Spatiotemporal Feature Learning Framework for Traffic Incident Duration Prediction
SIGSPATIAL/GIS(2019)
摘要
Critical incident stages identification and reasonable prediction of traffic incident duration are essential in traffic incident management. In this paper, we propose a traffic incident duration prediction model that simultaneously predicts the impact of the traffic incidents and identifies the critical groups of temporal features via a multi-task learning framework. First, we formulate a sparsity optimization problem that extracts low-level temporal features based on traffic speed readings and then generalizes higher level features as phases of traffic incidents. Second, we propose novel constraints on feature similarity exploiting prior knowledge about the spatial connectivity of the road network to predict the incident duration. The proposed problem is challenging to solve due to the orthogonality constraints, non-convexity objective, and non-smoothness penalties. We develop an algorithm based on the alternating direction method of multipliers (ADMM) framework to solve the proposed formulation. Extensive experiments and comparisons to other models on real-world traffic data and traffic incident records justify the efficacy of our model.
更多查看译文
关键词
intelligent transportation systems, feature learning, incident impact analysis
AI 理解论文
溯源树
样例
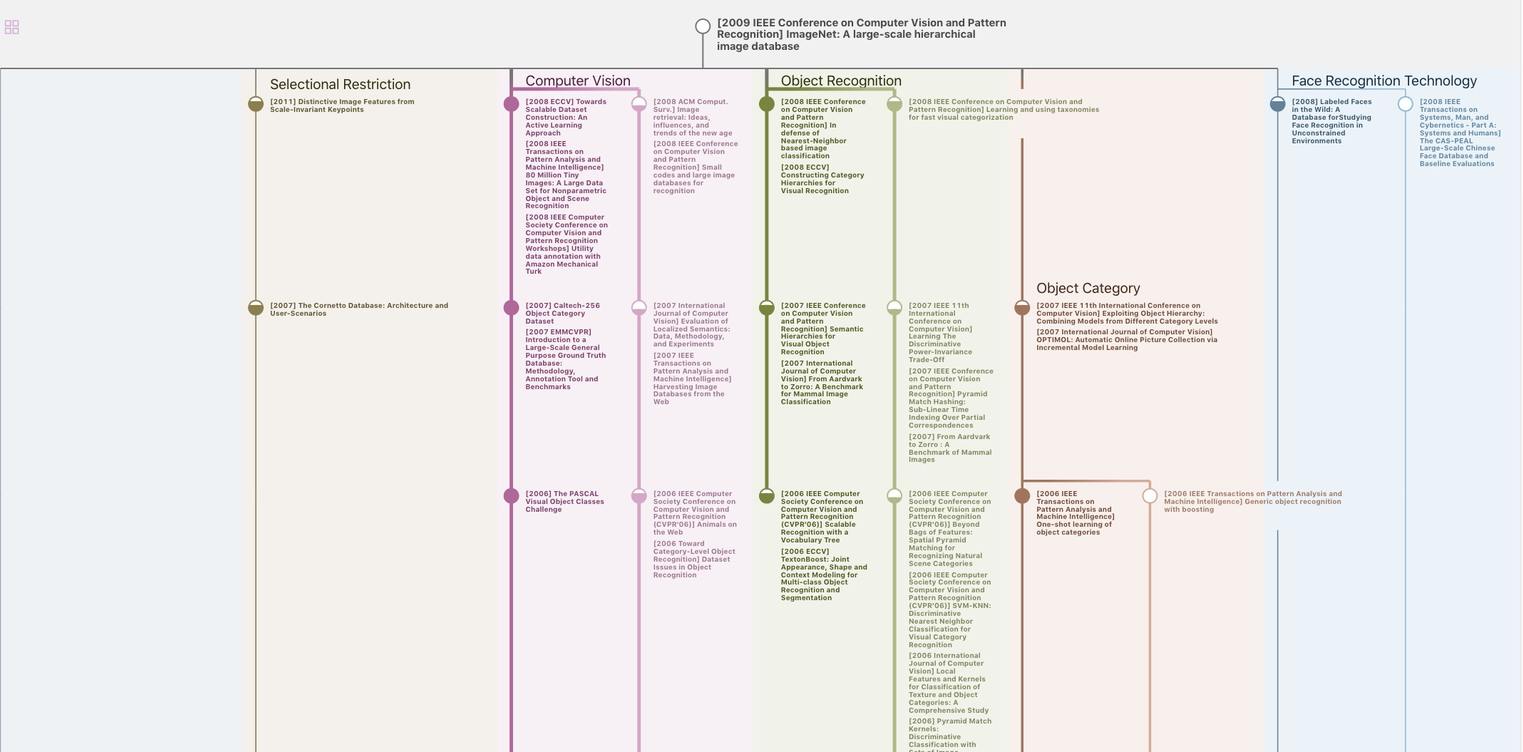
生成溯源树,研究论文发展脉络
Chat Paper
正在生成论文摘要