Deep Multiple Instance Learning for Human Trajectory Identification.
SIGSPATIAL/GIS(2019)
摘要
Extracting identifiable information from human trajectories is a fundamental task in many location-based services (LBS). However, various mobility patterns underlain in human trajectories are difficult to model by existing models. Moreover, we could hardly define a clear user set for user identification because the set of users are dynamic and changing everyday. Bearing these in mind, we apply a deep multiple instance learning method to handle the multimodal mobility patterns in a weak-supervised learning way, and address the dynamic user set problems via a pairwise loss with negative sampling. We utilize a multi-head attention mechanism to automatically extract multiple aspects and match the corresponding information between query trajectories and historical trajectories. Our method shows a good identification accuracy on three human GPS trajectory data sets comparing with baseline methods.
更多查看译文
关键词
Human trajectory modeling, neural networks, attention model
AI 理解论文
溯源树
样例
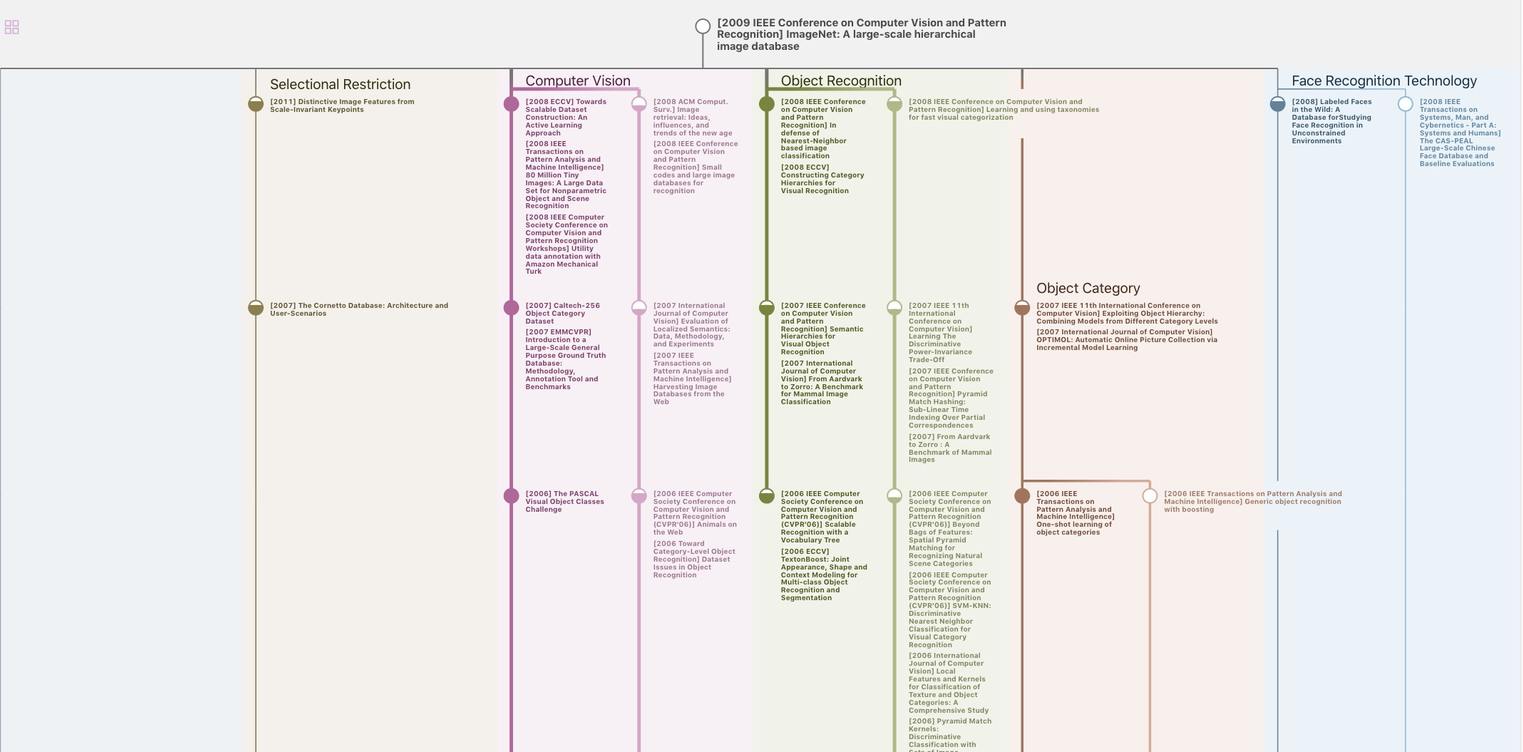
生成溯源树,研究论文发展脉络
Chat Paper
正在生成论文摘要