Projecting Flood-Inducing Precipitation with a Bayesian Analogue Model
Journal of Agricultural, Biological and Environmental Statistics(2020)
摘要
The hazard of pluvial flooding is largely influenced by the spatial and temporal dependence characteristics of precipitation. When extreme precipitation possesses strong spatial dependence, the risk of flooding is amplified due to catchment factors such as topography that cause runoff accumulation. Temporal dependence can also increase flood risk as storm water drainage systems operating at capacity can be overwhelmed by heavy precipitation occurring over multiple days. While transformed Gaussian processes are common choices for modeling precipitation, their weak tail dependence may lead to underestimation of flood risk. Extreme value models such as the generalized Pareto processes for threshold exceedances and max-stable models are attractive alternatives, but are difficult to fit when the number of observation sites is large, and are of little use for modeling the bulk of the distribution, which may also be of interest to water management planners. While the atmospheric dynamics governing precipitation are complex and difficult to fully incorporate into a parsimonious statistical model, non-mechanistic analogue methods that approximate those dynamics have proven to be promising approaches to capturing the temporal dependence of precipitation. In this paper, we present a Bayesian analogue method that leverages large, synoptic-scale atmospheric patterns to make precipitation forecasts. Changing spatial dependence across varying intensities is modeled as a mixture of spatial Student-t processes that can accommodate both strong and weak tail dependence. The proposed model demonstrates improved performance at capturing the distribution of extreme precipitation over Community Atmosphere Model (CAM) 5.2 forecasts. Supplementary materials accompanying this paper appear online.
更多查看译文
关键词
Dynamical system, Extreme value analysis, Stochastic weather generator, Student-t mixture
AI 理解论文
溯源树
样例
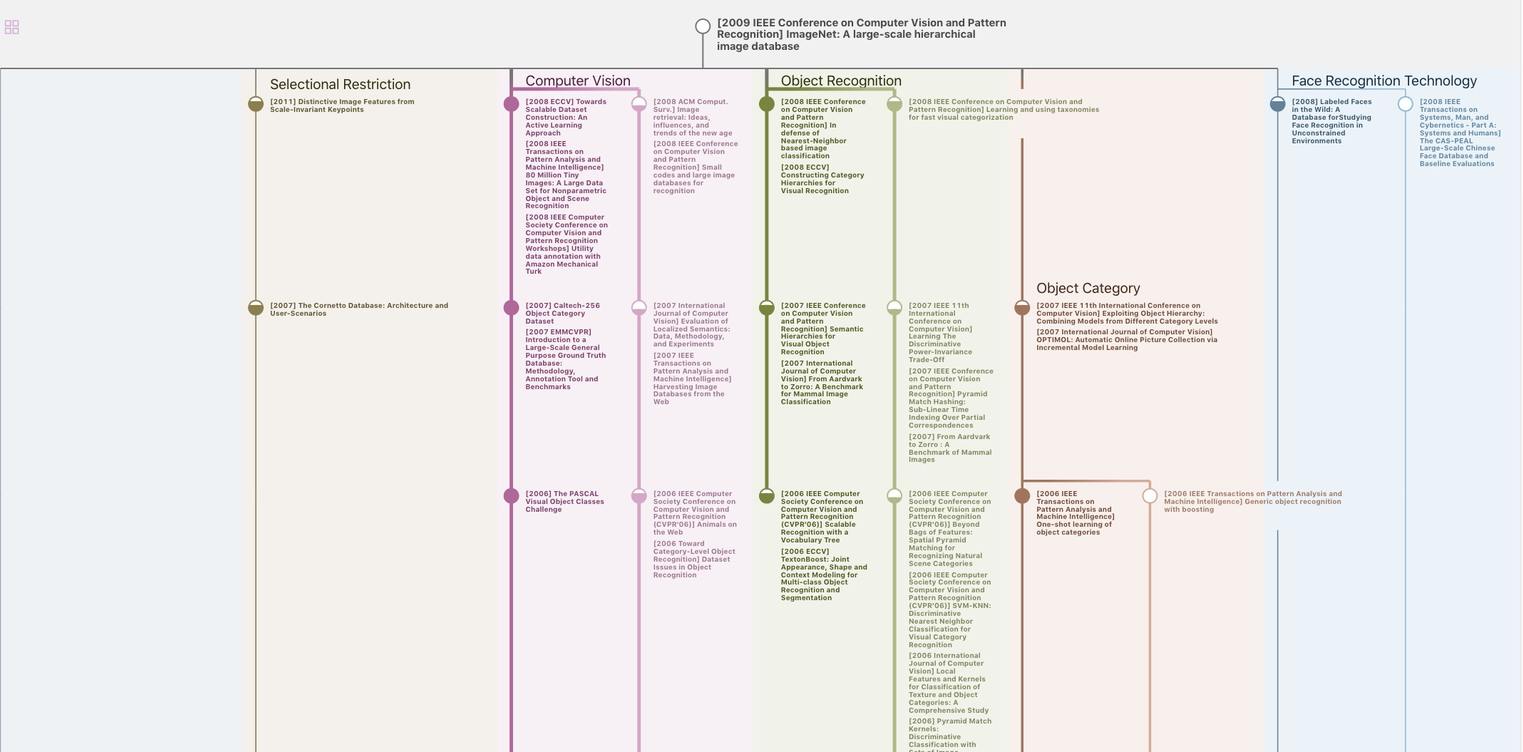
生成溯源树,研究论文发展脉络
Chat Paper
正在生成论文摘要