Predicting Unobserved Space for Planning via Depth Map Augmentation
2019 19th International Conference on Advanced Robotics (ICAR)(2019)
摘要
Safe and efficient path planning is crucial for autonomous mobile robots. A prerequisite for path planning is to have a comprehensive understanding of the 3D structure of the robot's environment. On Micro Air Vehicles (MAVs) this is commonly achieved using low-cost sensors, such as stereo or RGB-D cameras. These sensors may fail to provide depth measurements in textureless or IR-absorbing areas and have limited effective range. In path planning, this results in inefficient trajectories or failure to recognize a feasible path to the goal, hence significantly impairing the robot's mobility. Recent advances in deep learning enables us to exploit prior experience about the shape of the world and hence to infer complete depth maps from color images and additional sparse depth measurements. In this work, we present an augmented planning system and investigate the effects of employing state-of-the-art depth completion techniques, specifically trained to augment sparse depth maps originating from RGB-D sensors, semi-dense methods and stereo matchers. We extensively evaluate our approach in online path planning experiments based on simulated data, as well as global path planning experiments based on real world Micro Air Vehicle (MAV) data. We show that our augmented system, provided with only sparse depth perception, can reach on-par performance to ground truth depth input in simulated online planning experiments. On real world MAV data the augmented system demonstrates superior performance compared to a planner based on very dense RGB-D depth maps.
更多查看译文
关键词
unobserved space,depth map augmentation,safe path planning,autonomous mobile robots,MicroAir Vehicles,low-cost sensors,RGB-D cameras,IR-absorbing areas,sparse depth measurements,augmented planning system,sparse depth maps,online path planning experiments,global path planning experiments,augmented system,sparse depth perception,truth depth input,dense RGB-D depth maps,depth completion techniques,MicroAir Vehicle data,MAV data
AI 理解论文
溯源树
样例
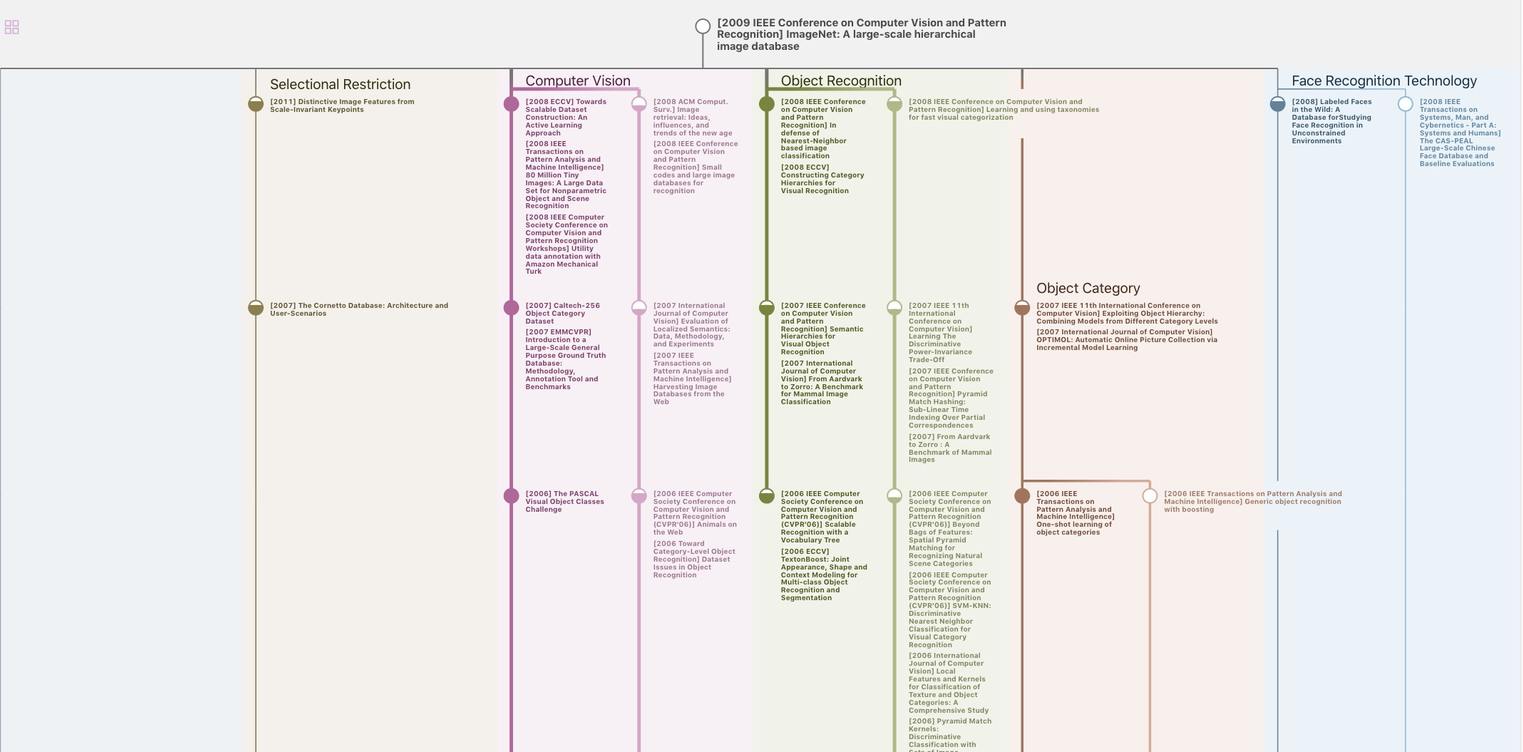
生成溯源树,研究论文发展脉络
Chat Paper
正在生成论文摘要