Gender Prediction Through Synthetic Resampling Of User Profiles Using Seqgans
COMPUTATIONAL DATA AND SOCIAL NETWORKS(2019)
摘要
Generative Adversarial Networks (GANs) have enabled researchers to achieve groundbreaking results on generating synthetic images. While GANs have been heavily used for generating synthetic image data, there is limited work on using GANs for synthetically resampling the minority class, particularly for text data. In this paper, we utilize Sequential Generative Adversarial Networks (SeqGAN) for creating synthetic user profiles from text data. The text data consists of articles that the users have read that are representative of the minority class. Our goal is to improve the predictive power of supervised learning algorithms for the gender prediction problem, using articles consumed by the user from a large health-based website as our data source. Our study shows that by creating synthetic user profiles for the minority class with SeqGANs and passing in the resampled training data to an XGBoost classifier, we achieve a gain of 2% in AUROC, as well as a 3% gain in both F1-Score and AUPR for gender prediction when compared to SMOTE. This is promising for the use of GANs in the application of text resampling.
更多查看译文
关键词
Gender prediction, Resampling, Adversarial, Topic modeling
AI 理解论文
溯源树
样例
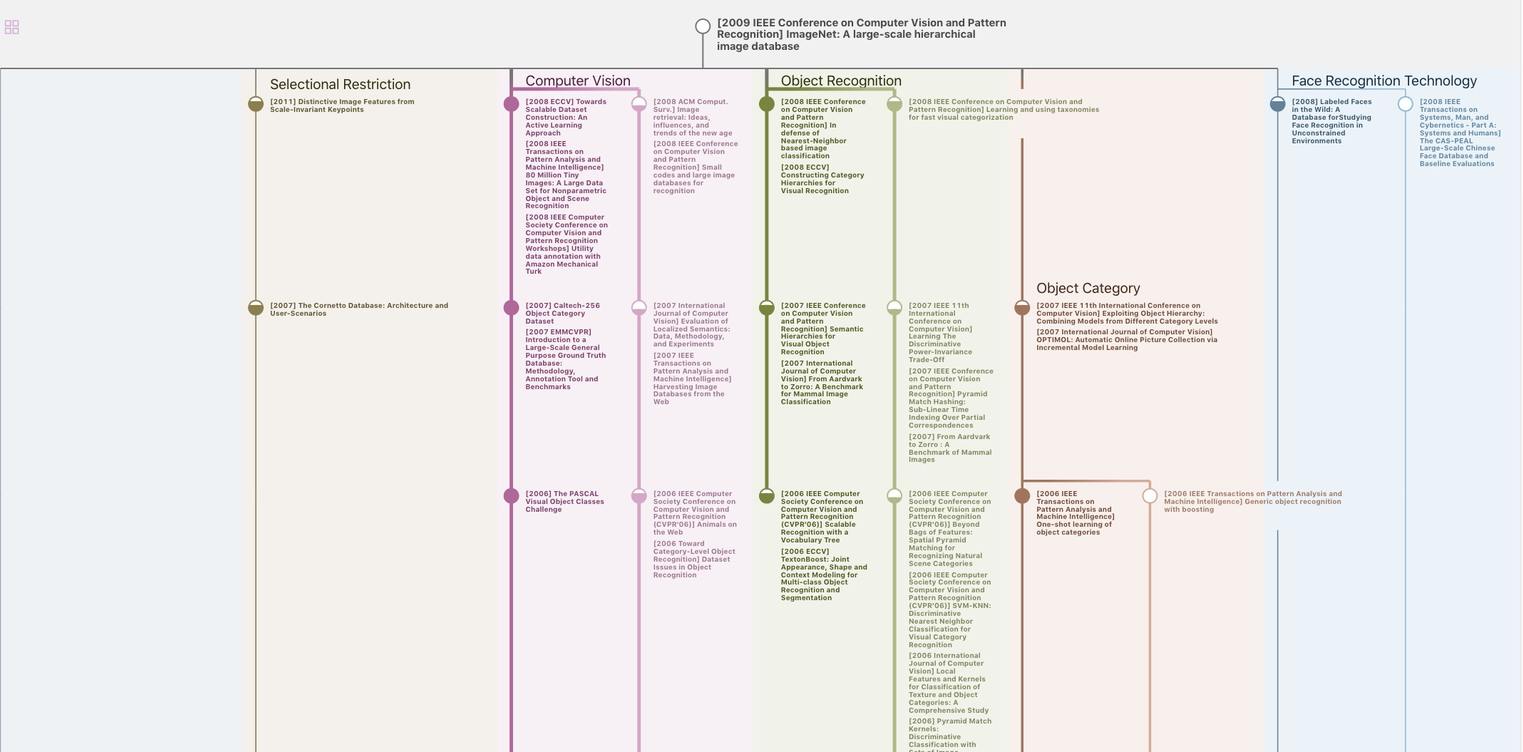
生成溯源树,研究论文发展脉络
Chat Paper
正在生成论文摘要