Variational Bayesian Point Set Registration
2019 IEEE 90TH VEHICULAR TECHNOLOGY CONFERENCE (VTC2019-FALL)(2019)
摘要
Point set registration presents unique significance in Lidar-based intelligent vehicle localization and mapping. It involves registering point sets of the same scene observed from different positions by determining their relative spatial transformation. However, due to the noise and outliers in the point sets and initial misalignment, existing methods suffer from the issues of low accuracy or large computational cost. In this paper, we propose a novel Bayesian state space model to describe the sequential point registration problem. Specifically, we specify the transformations to be the latent states and further assume that they vary smoothly across time. The point clouds are represented as Gaussian mixture models that change accordingly with the transformation. We develop a stochastic variational Bayesian inference algorithm to learning the distributions of the transformation, which automatically strikes a balance between mapping every two consecutive point clouds and the temporal smoothness of the transformation. Experimental results based synthetic data show that the proposed variational Bayesian point set registration (VB-PSR) algorithm achieves higher accuracy with comparable or less time and resources, in comparison with the state-of-the-art methods.
更多查看译文
关键词
variational Bayesian point set registration,point set registration,relative spatial transformation,sequential point registration problem,stochastic variational Bayesian inference algorithm,point clouds,Bayesian state space model,Gaussian mixture models,VB-PSR,intelligent vehicle localization and mapping,Lidar
AI 理解论文
溯源树
样例
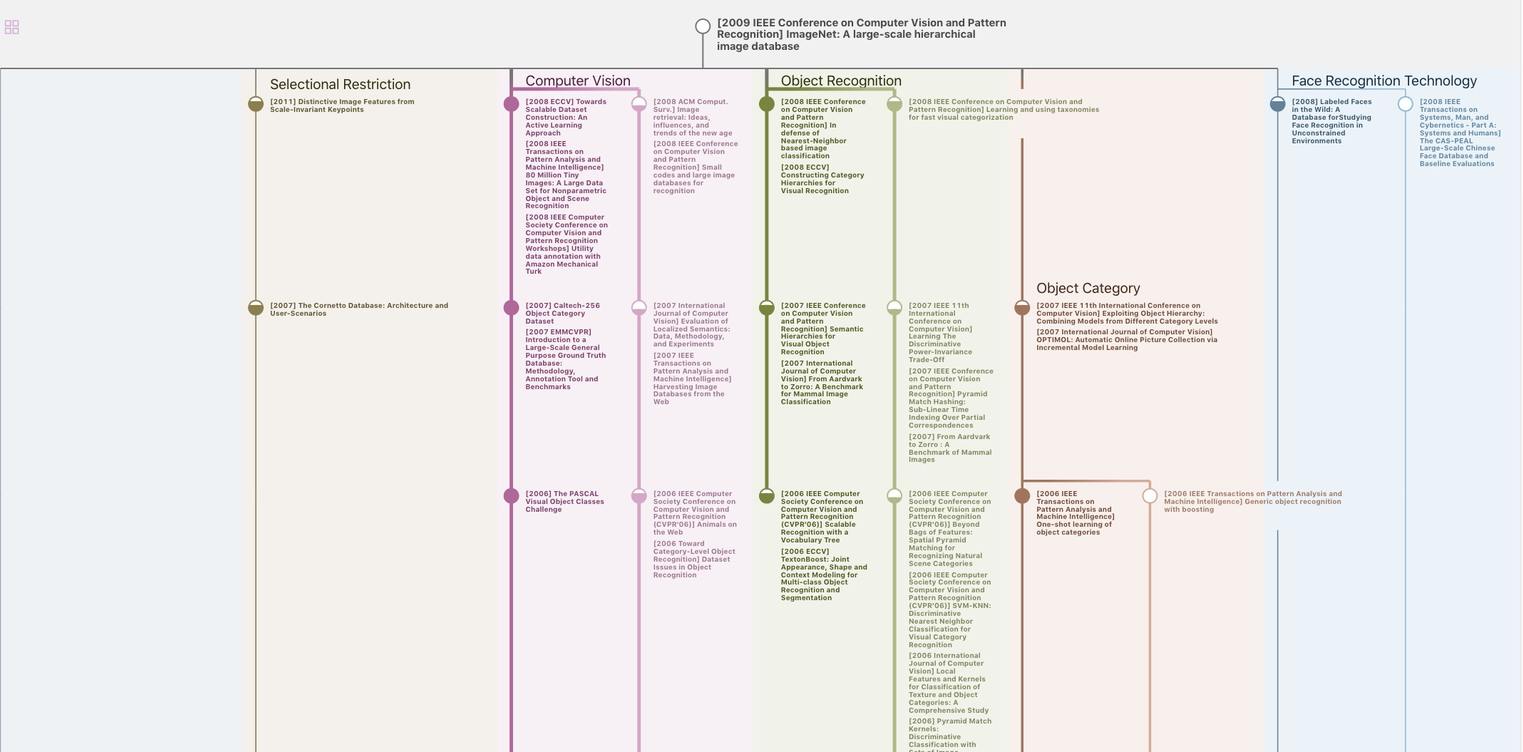
生成溯源树,研究论文发展脉络
Chat Paper
正在生成论文摘要