Semantic Segmentation Of Grey-Scale Traffic Scenes
IMAGE AND VIDEO TECHNOLOGY (PSIVT 2019)(2019)
摘要
In this paper we propose a novel architecture called KDNet that takes into account both spatial and temporal features for traffic scene labelling. One advantage of convolutional networks is the ability to yield hierarchical features that has been proved to bring high-quality result for scene labelling problem. We demonstrate that including temporal features has even better impact on segmenting the scene. We make use of convolutional long short-term memory cells in order to allow our model to take input at many different time steps. The backbone of our model is the well known fully convolutional network called FCN-8s. The model is built in an end-to-end manner, thus eliminating post-processing steps on its output. Our model outperforms FCN-8s at a significant margin on grey-scale video data.
更多查看译文
关键词
Semantic segmentation, Scene labelling, Per-pixel dense labelling, Grey-scale video data
AI 理解论文
溯源树
样例
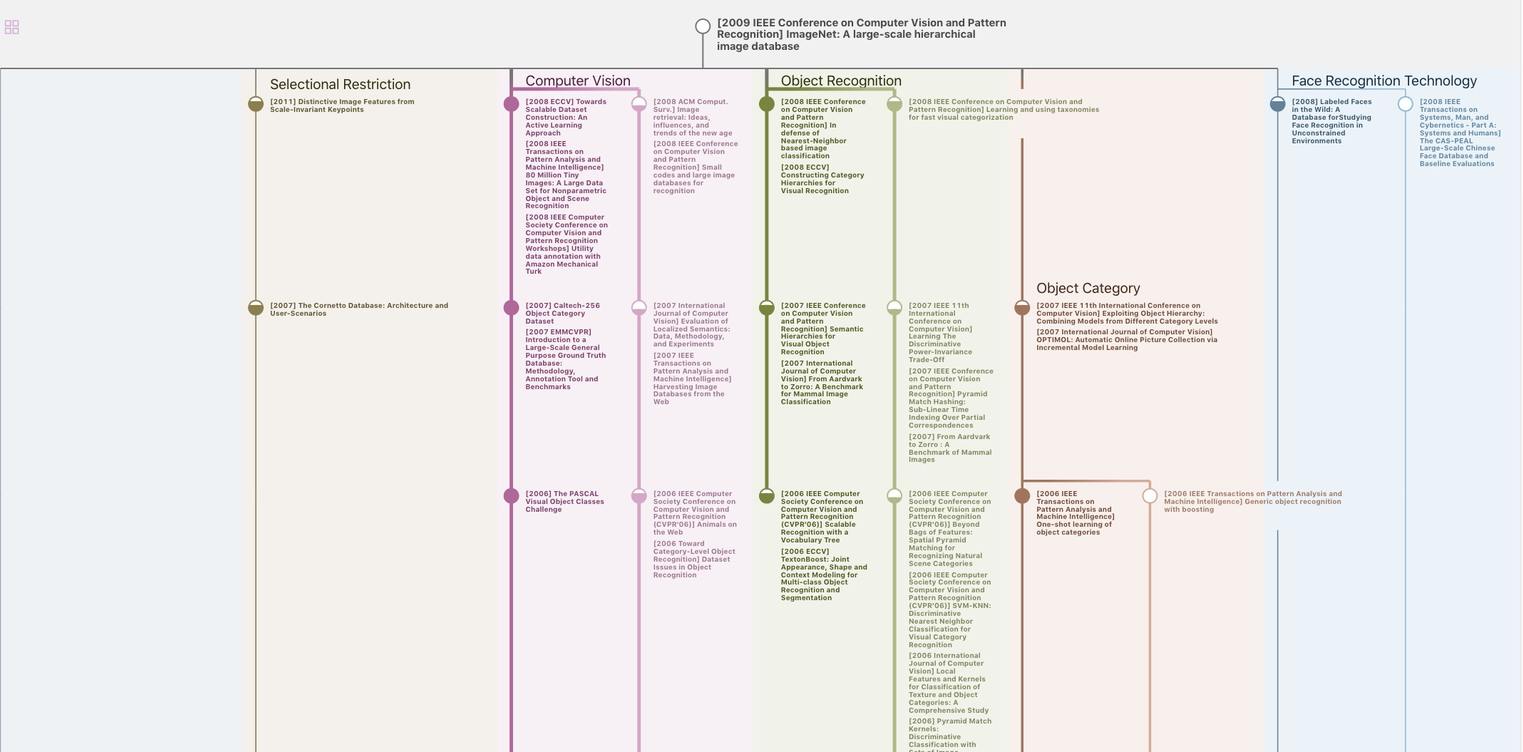
生成溯源树,研究论文发展脉络
Chat Paper
正在生成论文摘要