Development and Analysis of Neural Networks Realized in the Presence of Granular Data
IEEE Transactions on Neural Networks and Learning Systems(2020)
摘要
In this article, we propose a design and evaluation framework of granular neural networks realized in the presence of information granules. Neural networks realized in this manner are able to process both nonnumerical data, such as information granules as well as numerical data. Information granules are meaningful and semantically sound entities formed by organizing existing knowledge and available experimental data. The directional nature of mapping between the input and output data needs to be considered when building information granules. The development of neural networks advocated in this article is realized as a two-phase process. First, a collection of information granules is formed through granulation of numeric data in the input and output spaces. Second, neural networks are constructed on the basis of information granules rather than original (numeric) data. The proposed method leads to the construction of neural networks in a completely new way. In comparison with traditional (numeric) neural networks, the networks developed in the presence of granular data require shorter learning time. They also produce the results (outputs) that are information granules rather than numeric entities. The quality of granular outputs generated by our neural networks is evaluated in terms of the coverage and specificity criteria that are pertinent to the characterization of the information granules.
更多查看译文
关键词
Granular computing (GrC),granular neural network,information granule,principle of justifiable granularity
AI 理解论文
溯源树
样例
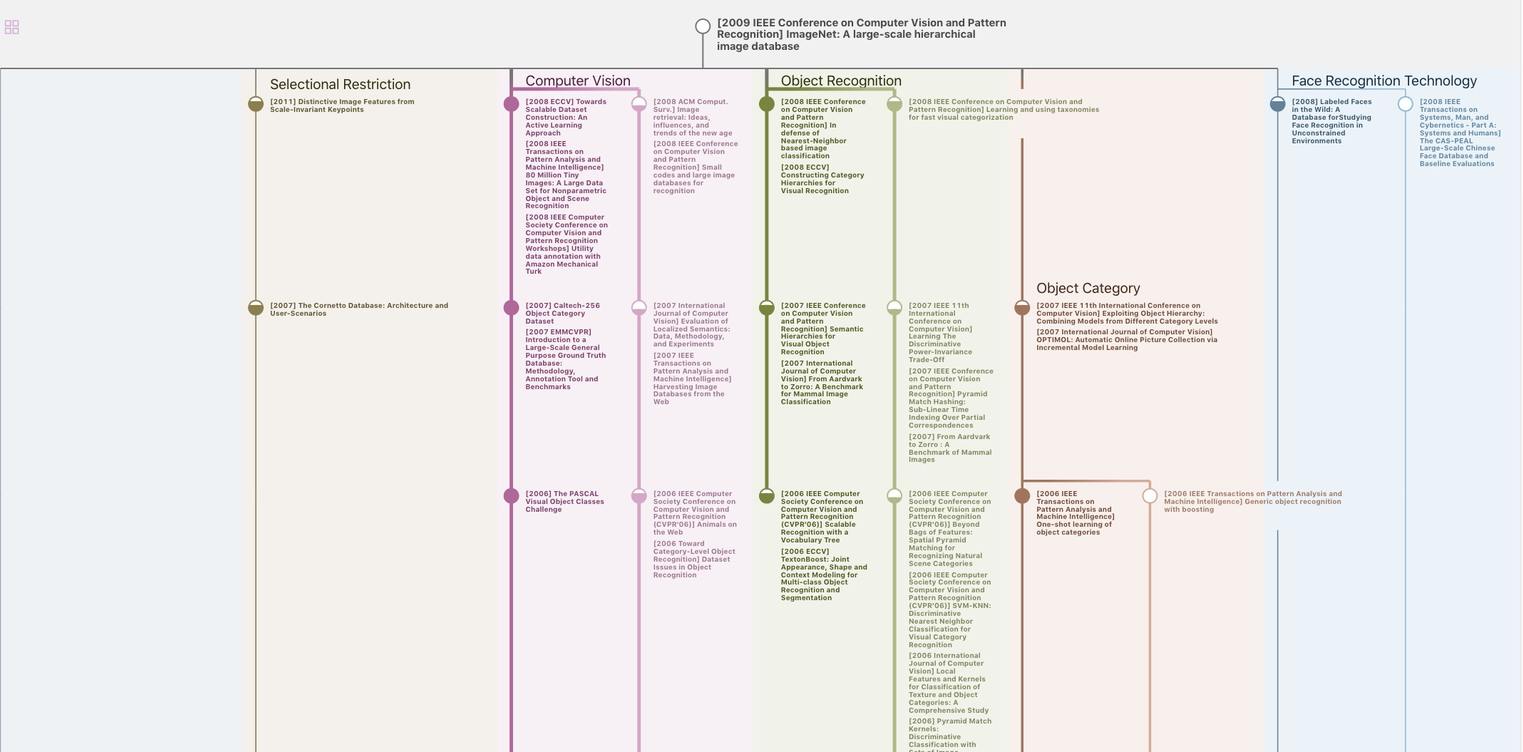
生成溯源树,研究论文发展脉络
Chat Paper
正在生成论文摘要