HyperSched - Dynamic Resource Reallocation for Model Development on a Deadline.
SoCC '19: ACM Symposium on Cloud Computing Santa Cruz CA USA November, 2019(2019)
摘要
Prior research in resource scheduling for machine learning training workloads has largely focused on minimizing job completion times. Commonly, these model training workloads collectively search over a large number of parameter values that control the learning process in a hyperparameter search. It is preferable to identify and maximally provision the best-performing hyperparameter configuration (trial) to achieve the highest accuracy result as soon as possible.
To optimally trade-off evaluating multiple configurations and training the most promising ones by a fixed deadline, we design and build HyperSched---a dynamic application-level resource scheduler to track, identify, and preferentially allocate resources to the best performing trials to maximize accuracy by the deadline. HyperSched leverages three properties of a hyperparameter search workload overlooked in prior work -- trial disposability, progressively identifiable rankings among different configurations, and space-time constraints -- to outperform standard hyperparameter search algorithms across a variety of benchmarks.
更多查看译文
关键词
Machine Learning Scheduling, Hyperparameter Optimization, Distributed Machine Learning
AI 理解论文
溯源树
样例
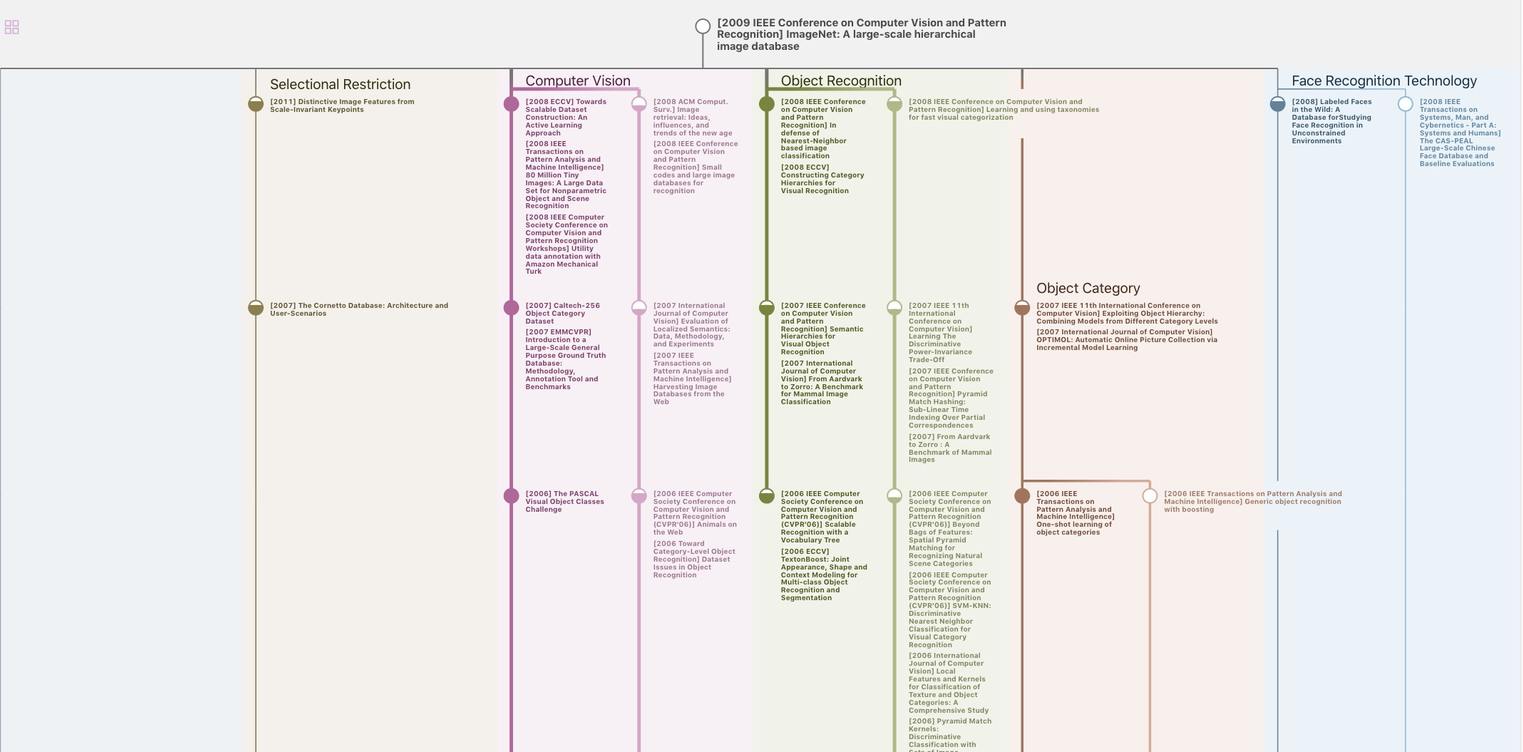
生成溯源树,研究论文发展脉络
Chat Paper
正在生成论文摘要