Vehicle-Rear: A New Dataset To Explore Feature Fusion For Vehicle Identification Using Convolutional Neural Networks
IEEE ACCESS(2021)
摘要
This work addresses the problem of vehicle identification through non-overlapping cameras. As our main contribution, we introduce a novel dataset for vehicle identification, called Vehicle-Rear, that contains more than three hours of high-resolution videos, with accurate information about the make, model, color and year of nearly 3,000 vehicles, in addition to the position and identification of their license plates. To explore our dataset we design a two-stream Convolutional Neural Network (CNN) that simultaneously uses two of the most distinctive and persistent features available: the vehicle's appearance and its license plate. This is an attempt to tackle a major problem: false alarms caused by vehicles with similar designs or by very close license plate identifiers. In the first network stream, shape similarities are identified by a Siamese CNN that uses a pair of low-resolution vehicle patches recorded by two different cameras. In the second stream, we use a CNN for Optical Character Recognition (OCR) to extract textual information, confidence scores, and string similarities from a pair of high-resolution license plate patches. Then, features from both streams are merged by a sequence of fully connected layers for decision. In our experiments, we compared the two-stream network against several well-known CNN architectures using single or multiple vehicle features. The architectures, trained models, and dataset are publicly available at https://github.com/icarofua/vehicle-rear.
更多查看译文
关键词
Licenses, Cameras, Feature extraction, Shape, Optical character recognition software, Videos, Roads, Vehicle identification, vehicle matching, multi-stream neural networks, feature fusion
AI 理解论文
溯源树
样例
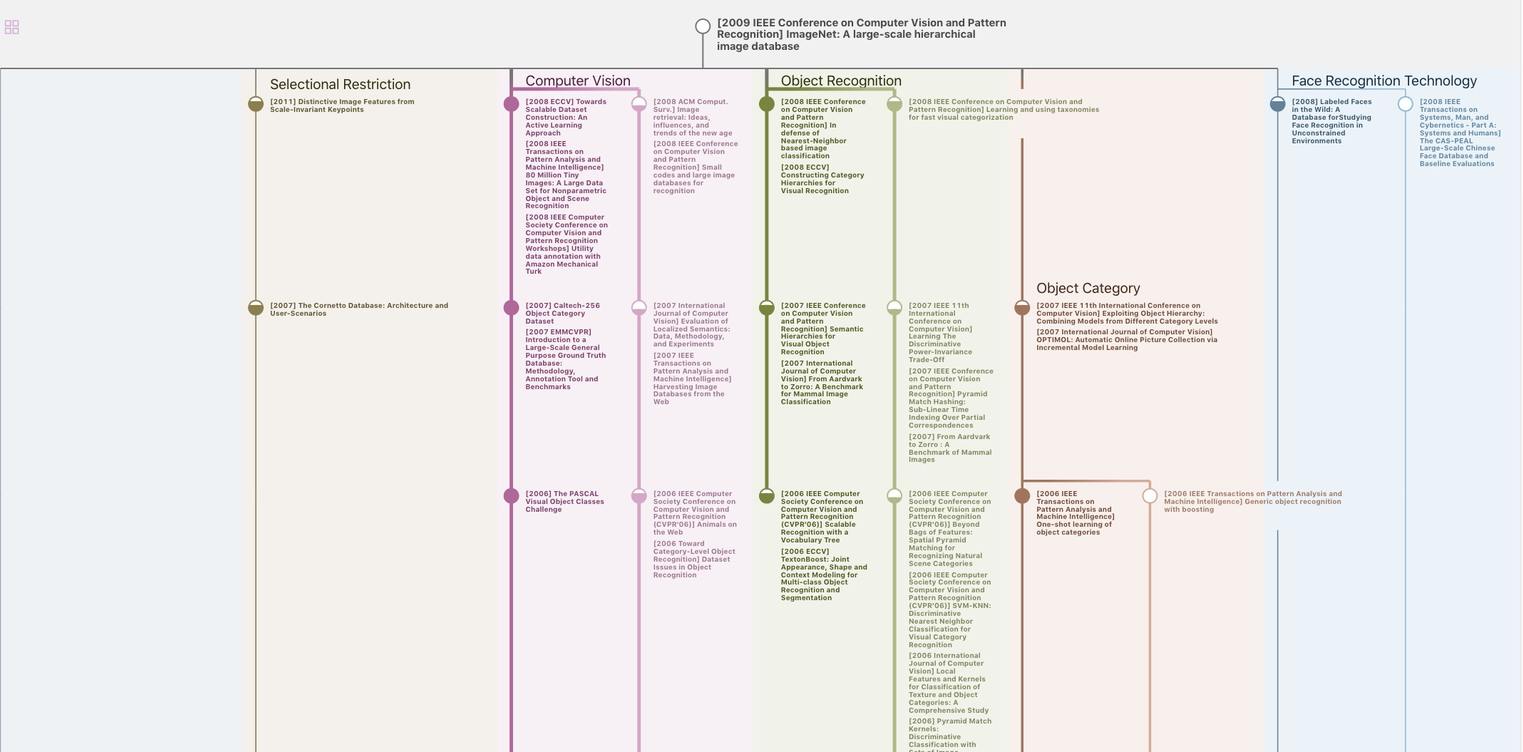
生成溯源树,研究论文发展脉络
Chat Paper
正在生成论文摘要