Relation Learning on Social Networks with Multi-Modal Graph Edge Variational Autoencoders.
PROCEEDINGS OF THE 13TH INTERNATIONAL CONFERENCE ON WEB SEARCH AND DATA MINING (WSDM '20)(2020)
摘要
While node semantics have been extensively explored in social networks, little research attention has been paid to pro le edge semantics, i.e., social relations. Ideal edge semantics should not only show that two users are connected, but also why they know each other and what they share in common. However, relations in social networks are often hard to pro le, due to noisy multi-modal signals and limited user-generated ground-truth labels.
In this work, we aim to develop a uni ed and principled frame- work that can pro le user relations as edge semantics in social networks by integrating multi-modal signals in the presence of noisy and incomplete data. Our framework is also exible towards limited or missing supervision. Speci cally, we assume a latent distribution of multiple relations underlying each user link, and learn them with multi-modal graph edge variational autoencoders. We encode the network data with a graph convolutional network, and decode arbitrary signals with multiple reconstruction networks. Extensive experiments and case studies on two public DBLP author networks and two internal LinkedIn member networks demonstrate the superior e ectiveness and e ciency of our proposed model.
更多查看译文
关键词
relation learning, social networks, graph variational autoencoder
AI 理解论文
溯源树
样例
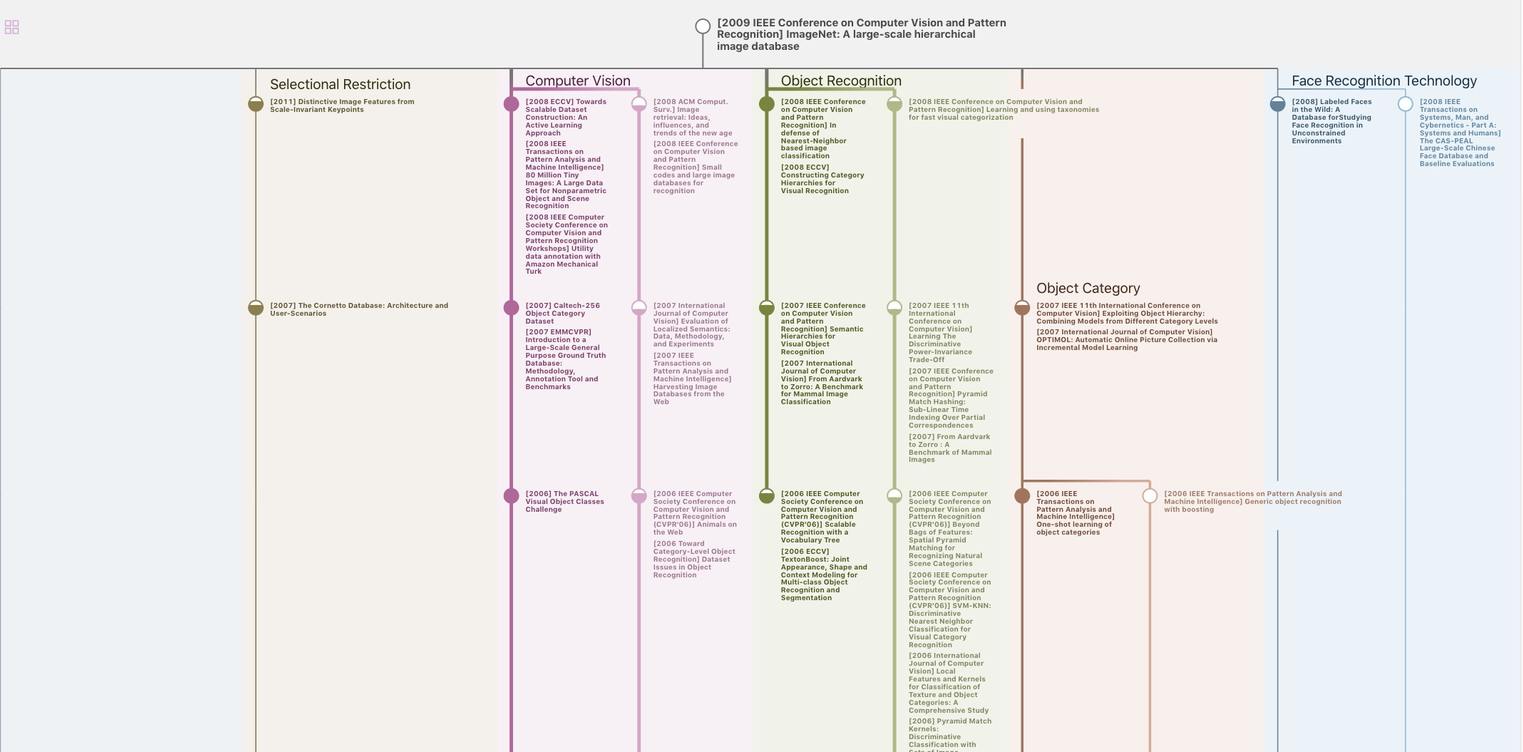
生成溯源树,研究论文发展脉络
Chat Paper
正在生成论文摘要