On The Choice Of Graph Neural Network Architectures
2020 IEEE INTERNATIONAL CONFERENCE ON ACOUSTICS, SPEECH, AND SIGNAL PROCESSING(2020)
摘要
Seminal works on graph neural networks have primarily targeted semi-supervised node classification problems with few observed labels and high-dimensional signals. With the development of graph networks, this setup has become a de facto benchmark for a significant body of research. Interestingly, several works have recently shown that in this particular setting, graph neural networks do not perform much better than predefined low-pass filters followed by a linear classifier. However, when learning from little data in a high-dimensional space, it is not surprising that simple and heavily regularized methods are near-optimal. In this paper, we show empirically that in settings with fewer features and more training data, more complex graph networks significantly outperform simple models, and propose a few insights towards the proper choice of graph network architectures. We finally outline the importance of using sufficiently diverse benchmarks (including lower dimensional signals as well) when designing and studying new types of graph neural networks.
更多查看译文
关键词
Graph neural networks, Semi-supervised learning, High-dimensional classification, Node classification
AI 理解论文
溯源树
样例
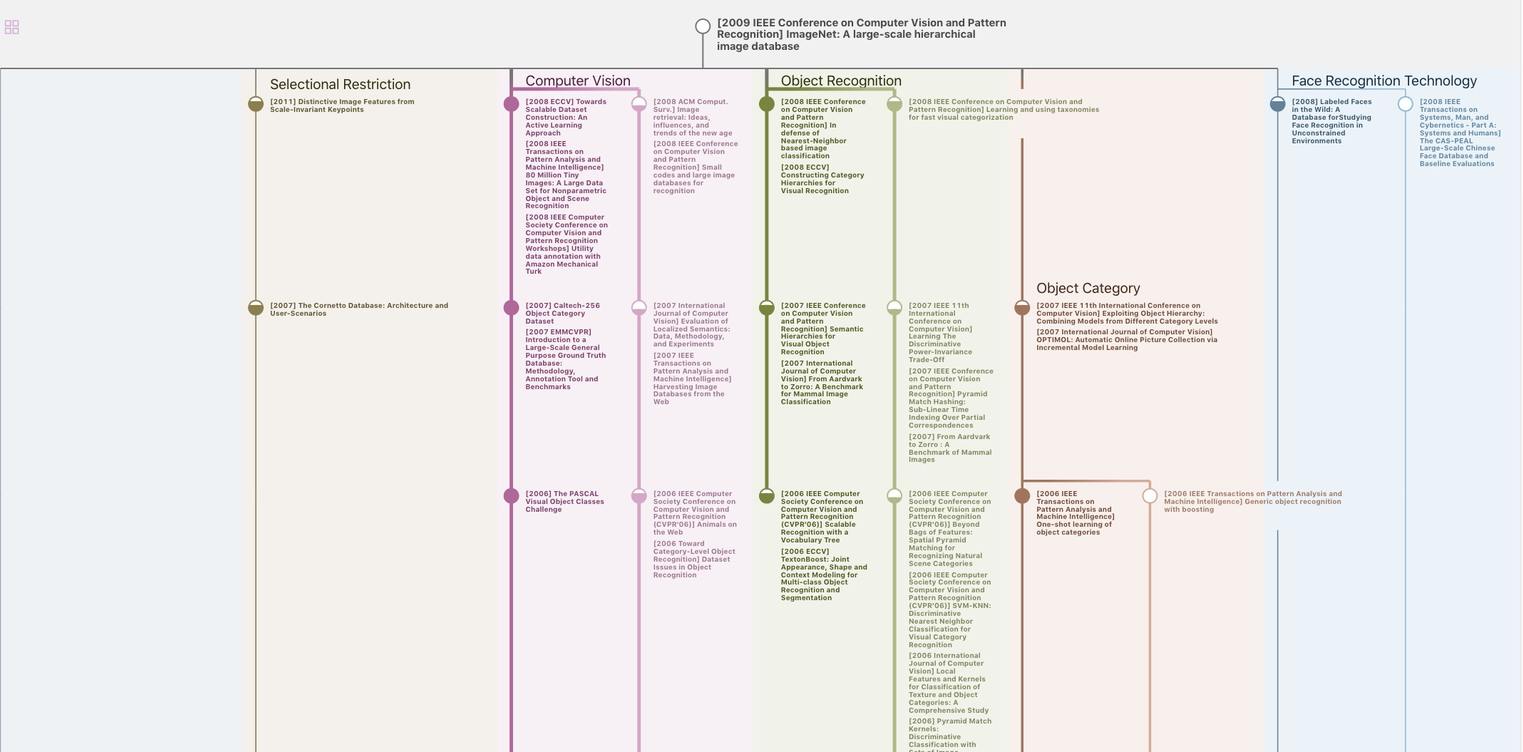
生成溯源树,研究论文发展脉络
Chat Paper
正在生成论文摘要