A Holistic Energy-Efficient Real-Time Scheduler for Mixed Stream and Batch Processing Workloads
IEEE Transactions on Parallel and Distributed Systems(2019)
摘要
In recent years we have experienced a wide adoption of novel distributed processing frameworks such as Apache Spark for handling batch and stream processing big data applications. An important aspect that has not been examined in these systems yet, is the energy consumption during the applications’ execution. Reducing the energy consumption of modern datacenters is a necessity, as datacenters contribute over 2 percent of the total US electric usage. However, efficiently scheduling applications in distributed processing systems can be challenging as there is a trade-off between minimizing the datacenter's energy usage and satisfying the application performance requirements. In this work we propose, ExpREsS, a scheduler for orchestrating the execution of Spark applications in a way that enables us to minimize the energy consumption while ensuring that the applications’ performance requirements are met. Our approach exploits time-series segmentation for capturing the applications’ energy usage and execution times, and then applies a novel DVFS technique to minimize the energy consumption. In order to tackle the limited number of application's profiling runs, we exploit regression techniques to predict the applications’ execution times and power consumption. Our detailed experimental evaluation using realistic workloads on our local cluster illustrates the working and benefits of our approach.
更多查看译文
关键词
Power demand,Energy consumption,Sparks,Cluster computing,Central Processing Unit,Time-frequency analysis,Distributed processing
AI 理解论文
溯源树
样例
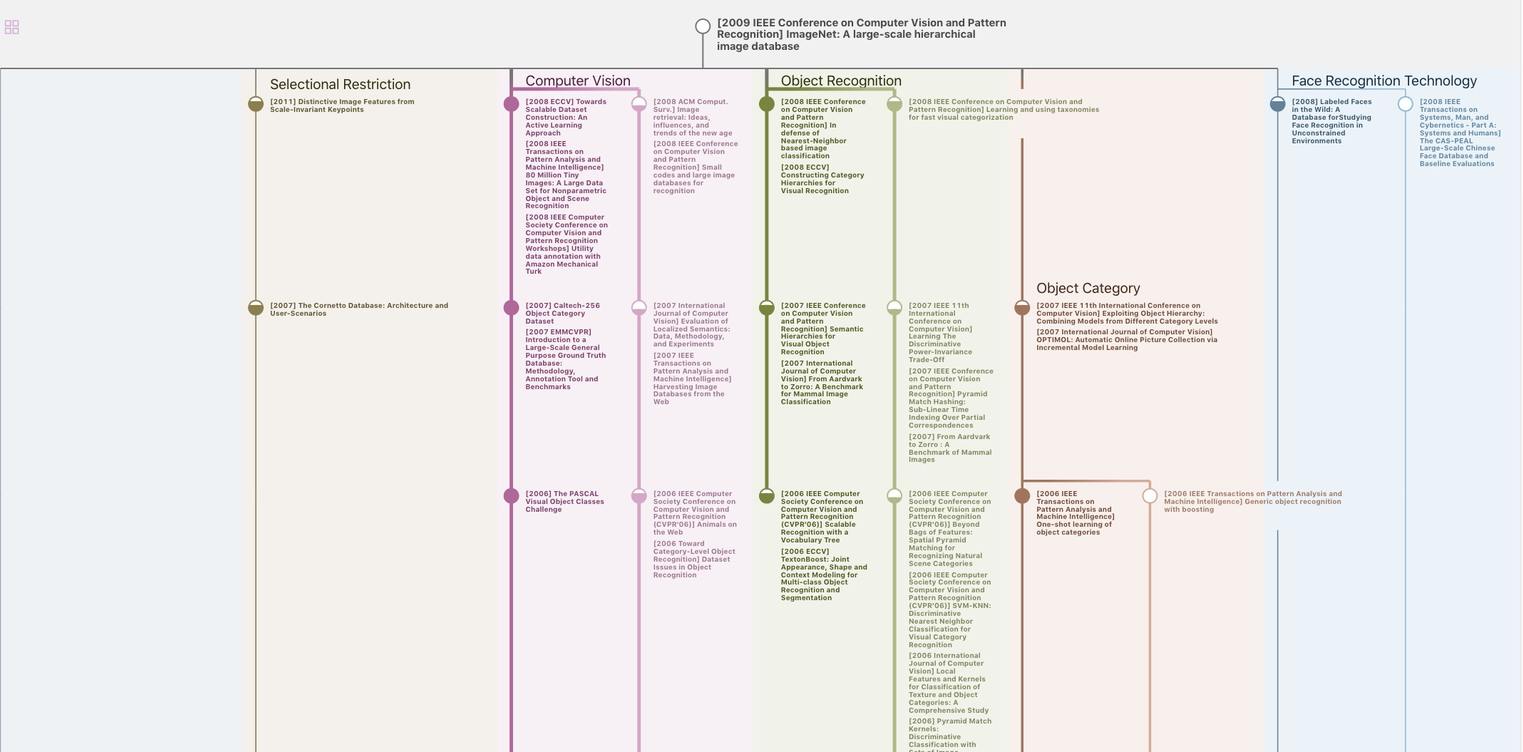
生成溯源树,研究论文发展脉络
Chat Paper
正在生成论文摘要