Non-Autoregressive Transformer Automatic Speech Recognition
arxiv(2019)
摘要
Recently very deep transformers start showing outperformed performance to traditional bi-directional long short-term memory networks by a large margin. However, to put it into production usage, inference computation cost and latency are still serious concerns in real scenarios. In this paper, we study a novel non-autoregressive transformers structure for speech recognition, which is originally introduced in machine translation. During training input tokens fed to the decoder are randomly replaced by a special mask token. The network is required to predict those mask tokens by taking both context and input speech into consideration. During inference, we start from all mask tokens and the network gradually predicts all tokens based on partial results. We show this framework can support different decoding strategies, including traditional left-to-right. A new decoding strategy is proposed as an example, which starts from the easiest predictions to difficult ones. Some preliminary results on Aishell and CSJ benchmarks show the possibility to train such a non-autoregressive network for ASR. Especially in Aishell, the proposed method outperformed Kaldi nnet3 and chain model setup and is quite closed to the performance of the start-of-the-art end-to-end model.
更多查看译文
AI 理解论文
溯源树
样例
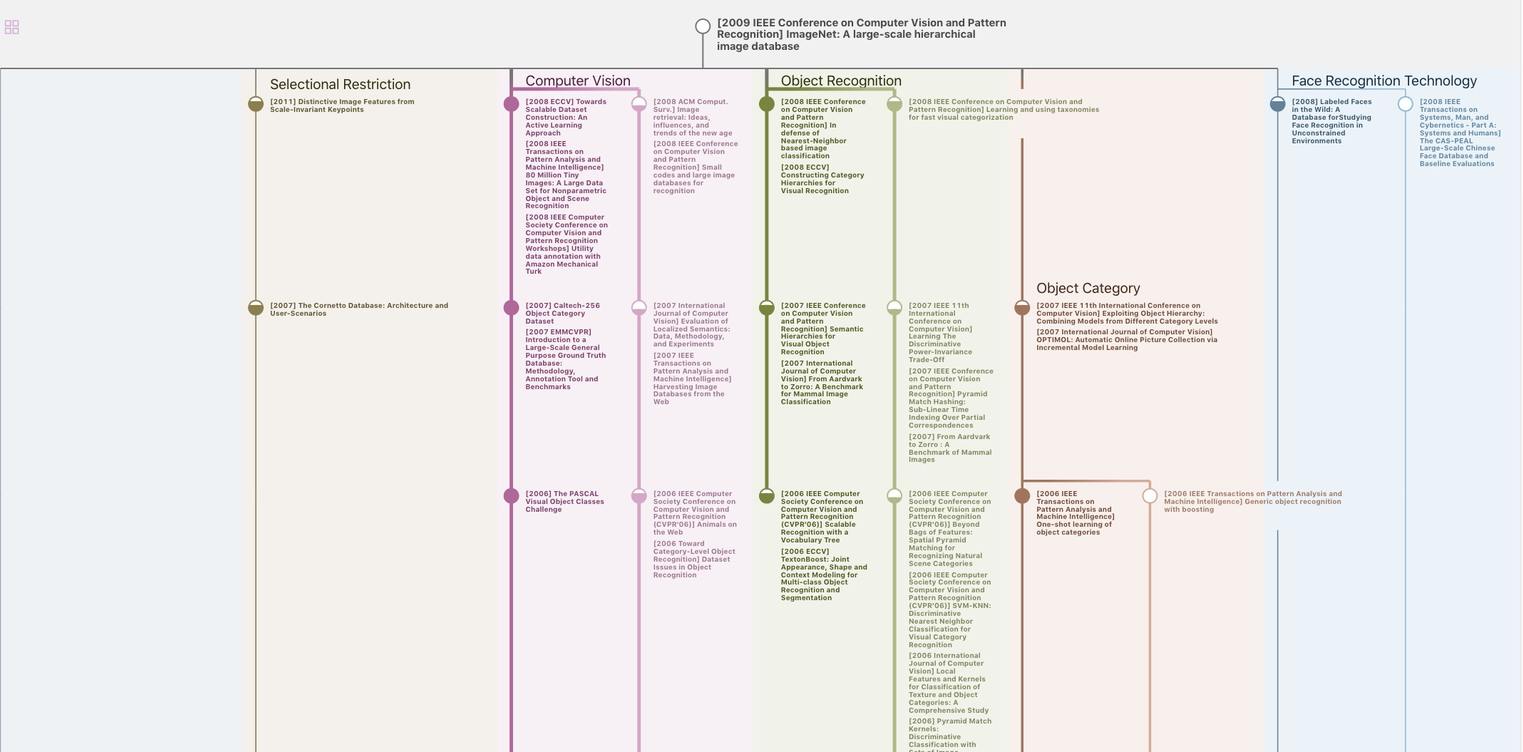
生成溯源树,研究论文发展脉络
Chat Paper
正在生成论文摘要