Text mining using nonnegative matrix factorization and latent semantic analysis
NEURAL COMPUTING & APPLICATIONS(2021)
摘要
Text clustering is considered one of the most important topics in modern data mining. Nevertheless, text data require tokenization which usually yields a very large and highly sparse term-document matrix, which is usually difficult to process using conventional machine learning algorithms. Methods such as latent semantic analysis have helped mitigate this issue, but are nevertheless not completely stable in practice. As a result, we propose a new feature agglomeration method based on nonnegative matrix factorization, which is employed to separate the terms into groups, and then each group’s term vectors are agglomerated into a new feature vector. Together, these feature vectors create a new feature space much more suitable for clustering. In addition, we propose a new deterministic initialization for spherical K -means, which proves very useful for this specific type of data. In order to evaluate the proposed method, we compare it to some of the latest research done in this field, as well as some of the most practiced methods. In our experiments, we conclude that the proposed method either significantly improves clustering performance or maintains the performance of other methods, while improving stability in results.
更多查看译文
关键词
Nonnegative matrix factorization, Text clustering, Latent semantic analysis, Dimensionality reduction
AI 理解论文
溯源树
样例
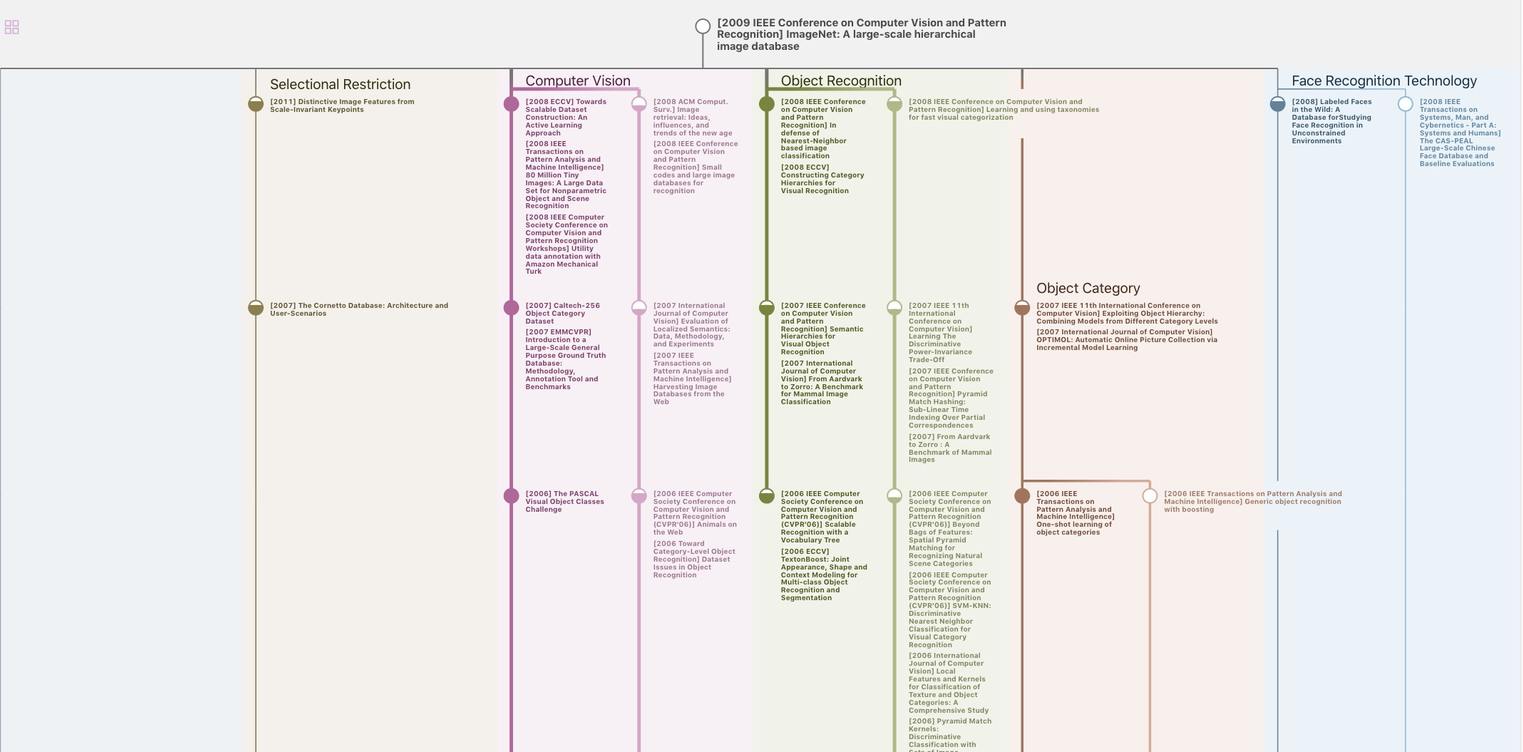
生成溯源树,研究论文发展脉络
Chat Paper
正在生成论文摘要