Throughput Prediction of Asynchronous SGD in TensorFlow
ICPE '20: ACM/SPEC International Conference on Performance Engineering Edmonton AB Canada April, 2020(2020)
摘要
Modern machine learning frameworks can train neural networks using multiple nodes in parallel, each computing parameter updates with stochastic gradient descent (SGD) and sharing them asynchronously through a central parameter server. Due to communication overhead and bottlenecks, the total throughput of SGD updates in a cluster scales sublinearly, saturating as the number of nodes increases. In this paper, we present a solution to predicting training throughput from profiling traces collected from a single-node configuration. Our approach is able to model the interaction of multiple nodes and the scheduling of concurrent transmissions between the parameter server and each node. By accounting for the dependencies between received parts and pending computations, we predict overlaps between computation and communication and generate synthetic execution traces for configurations with multiple nodes. We validate our approach on TensorFlow training jobs for popular image classification neural networks, on AWS and on our in-house cluster, using nodes equipped with GPUs or only with CPUs. We also investigate the effects of data transmission policies used in TensorFlow and the accuracy of our approach when combined with optimizations of the transmission schedule.
更多查看译文
关键词
Distributed Machine Learning, TensorFlow, SGD, Performance
AI 理解论文
溯源树
样例
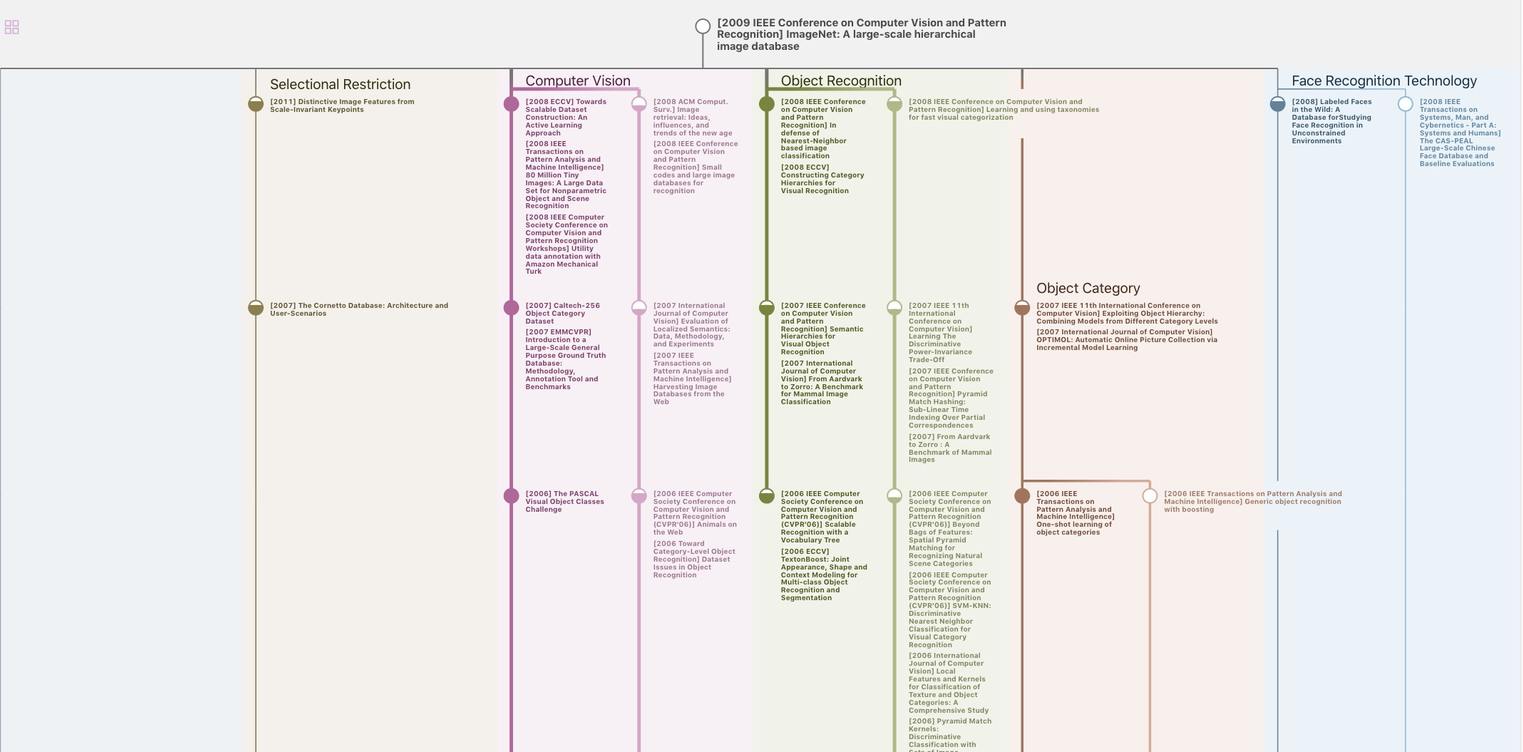
生成溯源树,研究论文发展脉络
Chat Paper
正在生成论文摘要