DRiLLS - Deep Reinforcement Learning for Logic Synthesis.
ASP-DAC(2020)
摘要
Logic synthesis requires extensive tuning of the synthesis optimization flow where the quality of results (QoR) depends on the sequence of optimizations used. Efficient design space exploration is challenging due to the exponential number of possible optimization permutations. Therefore, automating the optimization process is necessary. In this work, we propose a novel reinforcement learning-based methodology that navigates the optimization space without human intervention. We demonstrate the training of an Advantage Actor Critic (A2C) agent that seeks to minimize area subject to a timing constraint. Using the proposed methodology, designs can be optimized autonomously with no-humans in-loop. Evaluation on the comprehensive EPFL benchmark suite shows that the agent outperforms existing exploration methodologies and improves QoRs by an average of 13%.
更多查看译文
关键词
DRiLLS,deep reinforcement learning,logic synthesis,QoR,design space exploration,exponential number,optimization process,reinforcement learning-based methodology,advantage actor critic agent,EPFL benchmark suit,A2C agent,timing constraint,quality of results
AI 理解论文
溯源树
样例
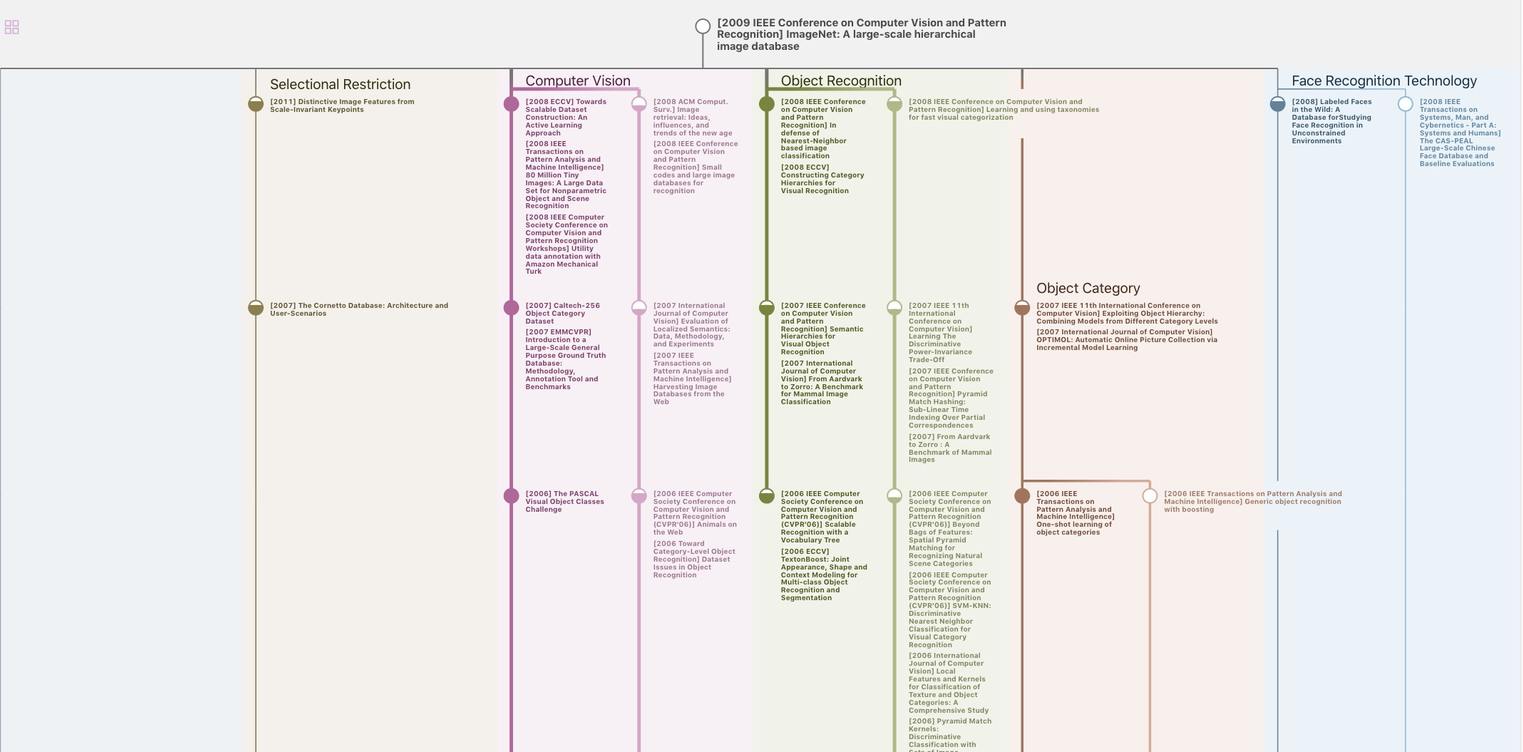
生成溯源树,研究论文发展脉络
Chat Paper
正在生成论文摘要