Estimating Higher-Order Moments Using Symmetric Tensor Decomposition
SIAM JOURNAL ON MATRIX ANALYSIS AND APPLICATIONS(2020)
摘要
We consider the problem of decomposing higher-order moment tensors, i.e., the sum of symmetric outer products of data vectors. Such a decomposition can be used to estimate the means in a Gaussian mixture model and for other applications in machine learning. The dth-order empirical moment tensor of a set of p observations of n variables is a symmetric d-way tensor. Our goal is to find a low-rank tensor approximation comprising r << p symmetric outer products. The challenge is that forming the empirical moment tensors costs O(pn(d)) operations and O(n(d)) storage, which may be prohibitively expensive; additionally, the algorithm to compute the low-rank approximation costs O(n(d)) per iteration. Our contribution is avoiding formation of the moment tensor, computing the low-rank tensor approximation of the moment tensor implicitly using O(pnr) operations per iteration and no extra memory. This advance opens the door to more applications of higher-order moments since they can now be efficiently computed. We present numerical evidence of the computational savings and show an example of estimating the means for higher-order moments.
更多查看译文
关键词
higher-order moments,higher-order cumulants,Gaussian mixture models,symmetric tensor decomposition,implicit tensor formation
AI 理解论文
溯源树
样例
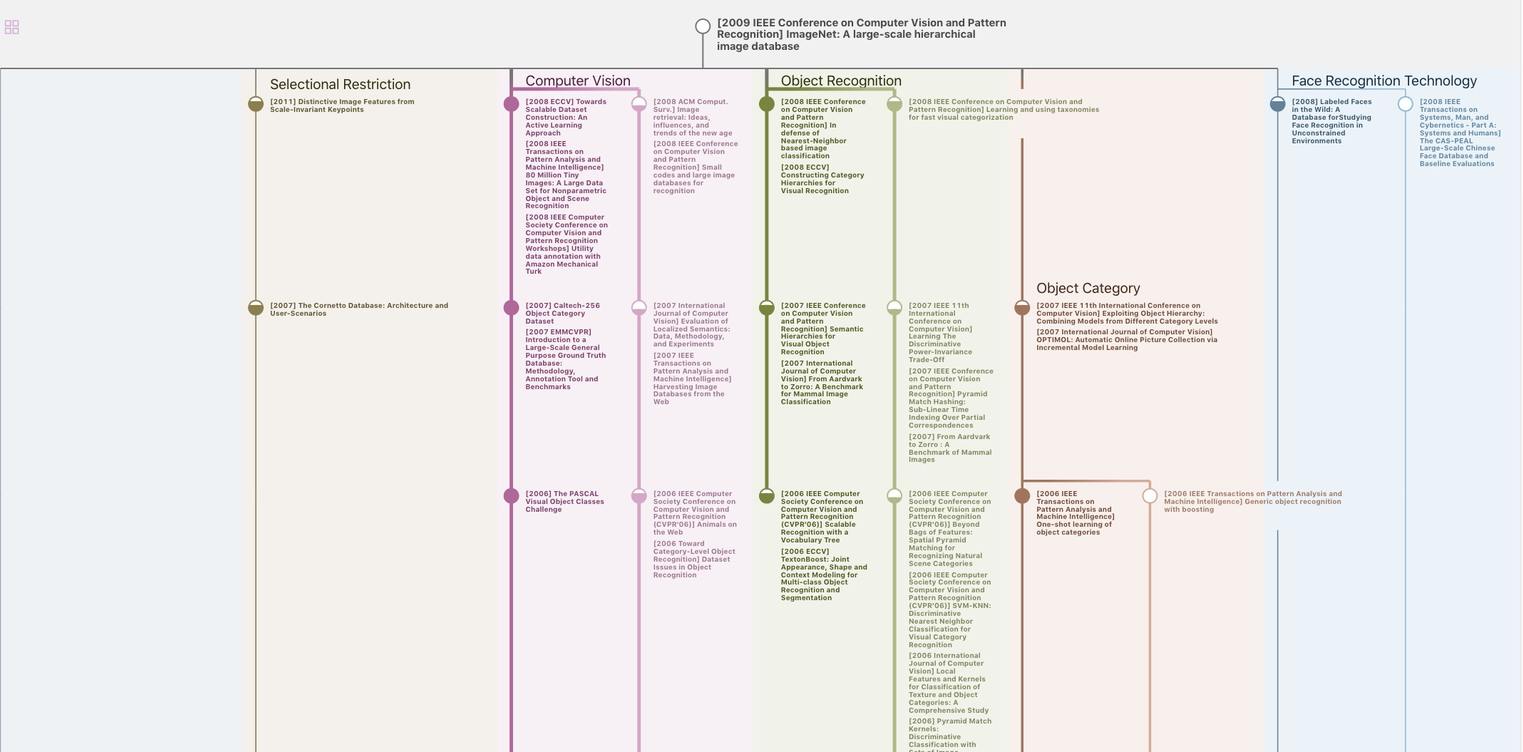
生成溯源树,研究论文发展脉络
Chat Paper
正在生成论文摘要