Transforming Wikipedia Into Augmented Data for Query-Focused Summarization
IEEE-ACM TRANSACTIONS ON AUDIO SPEECH AND LANGUAGE PROCESSING(2022)
摘要
The limited size of existing query-focused summarization datasets renders training data-driven summarization models challenging. Meanwhile, the manual construction of a query-focused summarization corpus is costly and time-consuming. In this paper, we use Wikipedia to automatically collect a large query-focused summarization dataset (named WikiRef) of more than 280,000 examples, which can serve as a means of data augmentation. We also develop a BERT-based query-focused summarization model (Q-BERT) to extract sentences from the documents as summaries. To better adapt a huge model containing millions of parameters to tiny benchmarks, we identify and fine-tune only a sparse subnetwork, which corresponds to a small fraction of the whole model parameters. Experimental results on three DUC benchmarks show that the model pre-trained on WikiRef has already achieved reasonable performance. After fine-tuning on the specific benchmark datasets, the model with data augmentation outperforms strong comparison systems. Moreover, both our proposed Q-BERT model and subnetwork fine-tuning further improve the model performance.
更多查看译文
关键词
Encyclopedias, Online services, Internet, Benchmark testing, Data models, Biological system modeling, Speech processing, Query-focused summarization, natural language processing, data augmentation, neural networks
AI 理解论文
溯源树
样例
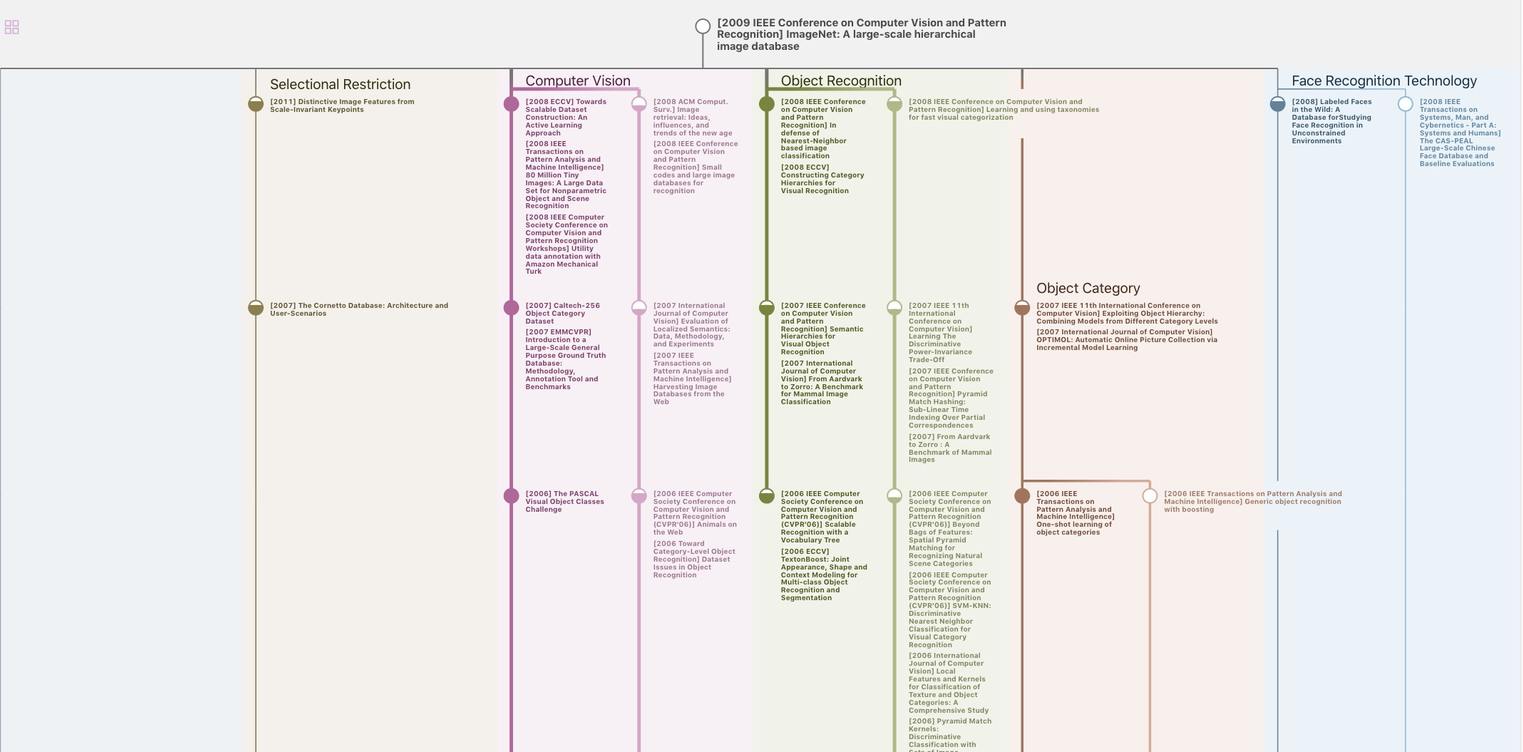
生成溯源树,研究论文发展脉络
Chat Paper
正在生成论文摘要