Balancing Covariates in Randomized Experiments with the Gram-Schmidt Walk Design
JOURNAL OF THE AMERICAN STATISTICAL ASSOCIATION(2024)
摘要
The design of experiments involves a compromise between covariate balance and robustness. This article provides a formalization of this tradeoff and describes an experimental design that allows experimenters to navigate it. The design is specified by a robustness parameter that bounds the worst-case mean squared error of an estimator of the average treatment effect. Subject to the experimenter's desired level of robustness, the design aims to simultaneously balance all linear functions of potentially many covariates. Less robustness allows for more balance. We show that the mean squared error of the estimator is bounded in finite samples by the minimum of the loss function of an implicit ridge regression of the potential outcomes on the covariates. Asymptotically, the design perfectly balances all linear functions of a growing number of covariates with a diminishing reduction in robustness, effectively allowing experimenters to escape the compromise between balance and robustness in large samples. Finally, we describe conditions that ensure asymptotic normality and provide a conservative variance estimator, which facilitate the construction of asymptotically valid confidence intervals. Supplementary materials for this article are available online.
更多查看译文
关键词
Causal inference,Covariate balance,Treatment effects
AI 理解论文
溯源树
样例
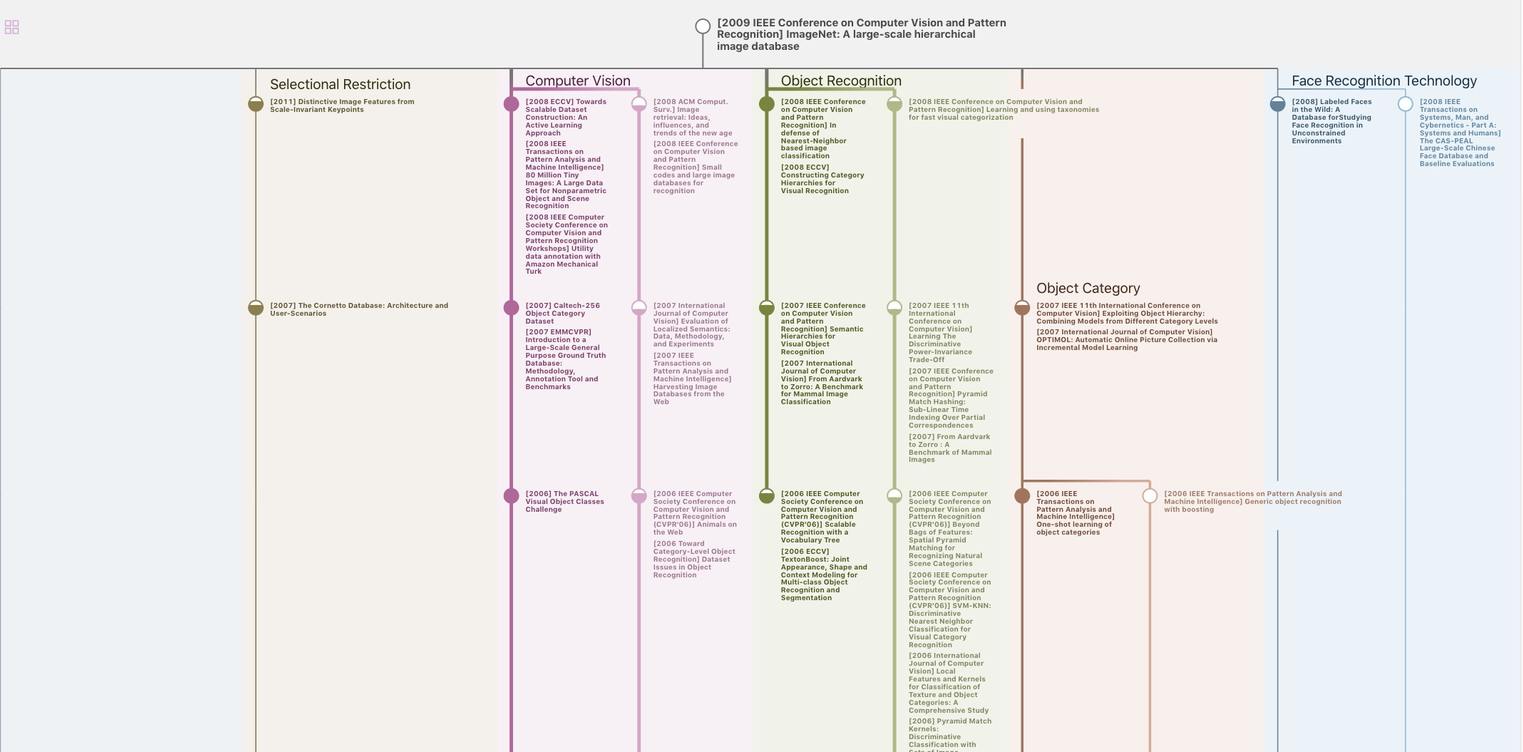
生成溯源树,研究论文发展脉络
Chat Paper
正在生成论文摘要