Joint Optimization Of Sampling Patterns And Deep Priors For Improved Parallel Mri
2020 IEEE INTERNATIONAL CONFERENCE ON ACOUSTICS, SPEECH, AND SIGNAL PROCESSING(2020)
摘要
Multichannel imaging techniques are widely used in MRI to reduce the scan time. These schemes typically perform undersampled acquisition and utilize compressed-sensing based regularized reconstruction algorithms. Model-based deep learning (MoDL) frameworks are now emerging as powerful alternatives to compressed sensing, with significantly improved image quality. In this work, we investigate the impact of sampling patterns on the quality of the image recovered using the MoDL algorithm. We introduce a scheme to jointly optimize the sampling pattern and the reconstruction network parameters in MoDL for parallel MRI. The improved decoupling of the network parameters from the sampling patterns offered by the MoDL scheme translates to improved optimization and thus improved performance. Preliminary experimental results demonstrate that the proposed joint optimization framework significantly improves the image quality.
更多查看译文
关键词
sampling, parallel MRI, deep learning
AI 理解论文
溯源树
样例
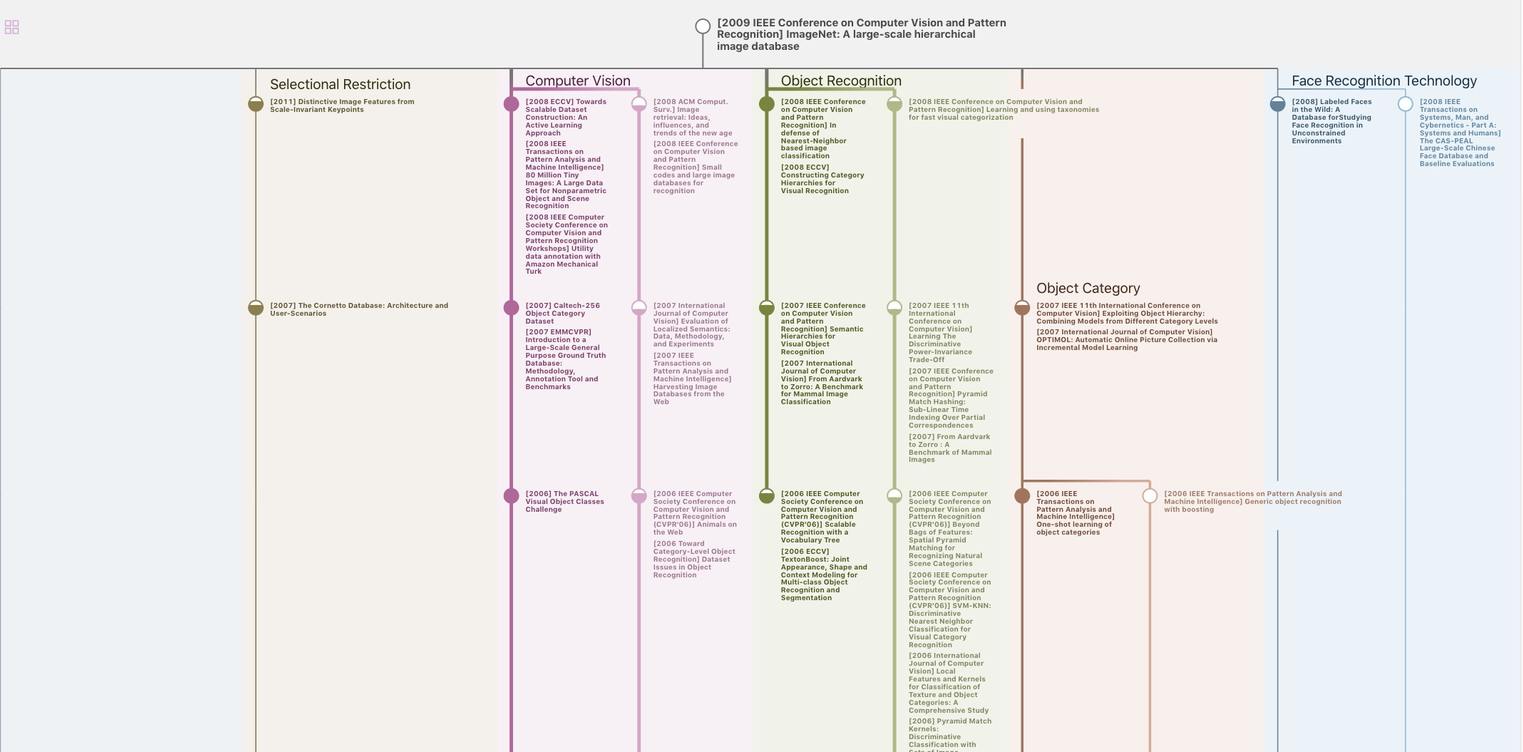
生成溯源树,研究论文发展脉络
Chat Paper
正在生成论文摘要