Change Your Singer: A Transfer Learning Generative Adversarial Framework For Song To Song Conversion
2020 INTERNATIONAL JOINT CONFERENCE ON NEURAL NETWORKS (IJCNN)(2020)
摘要
Have you ever wondered how a song might sound if performed by a different artist? In this work, we propose SCM-GAN, an end-to-end non-parallel song conversion system powered by generative adversarial and transfer learning, which allows users to listen to a selected target singer singing any song. SCM-GAN first separates songs into vocals and instrumental music using a U-Net network, then converts the vocal segments to the target singer using advanced CycleGAN-VC, before merging the converted vocals with their corresponding background music. SCM-GAN is first initialized with feature representations learned from a state-of-the-art voice-to-voice conversion and then trained on a dataset of non-parallel songs. After that, SCM-GAN is evaluated against a set of metrics including global variance GV and modulation spectra MS on the 24 Mel-cepstral coefficients (MCEPs). Transfer learning improves the GV by 35% and the MS by 13% on average. A subjective comparison is conducted to test the output's similarity to the target singer and its naturalness. Results show that the SCM-GAN's similarity between its output and the target reaches 69%, and its naturalness reaches 54%.
更多查看译文
关键词
Generative Adversarial Networks, Song to Song Conversion, Voice to Voice, Transfer Learning
AI 理解论文
溯源树
样例
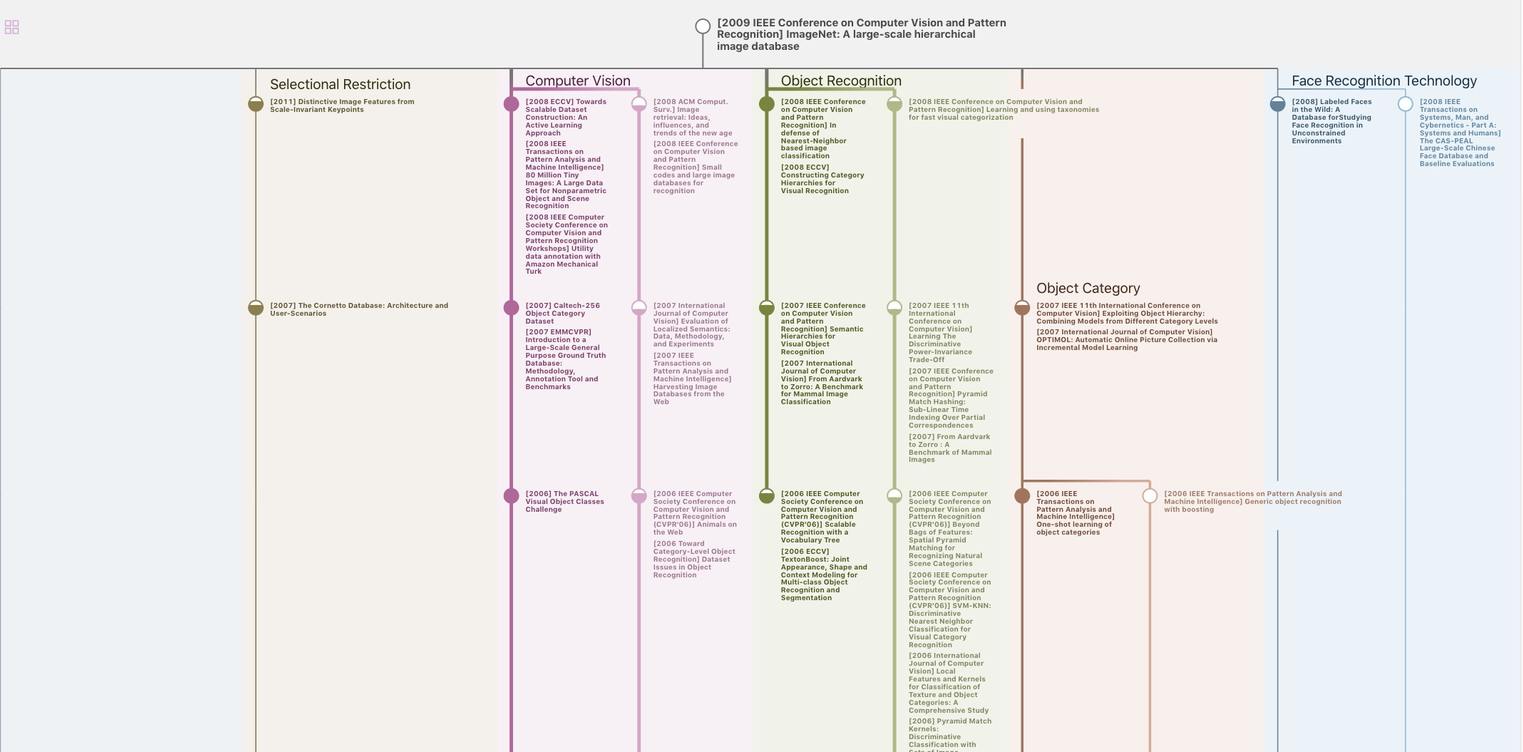
生成溯源树,研究论文发展脉络
Chat Paper
正在生成论文摘要