Using deep convolutional neural networks for multi-classification of thyroid tumor by histopathology: a large-scale pilot study.
ANNALS OF TRANSLATIONAL MEDICINE(2019)
摘要
Background: To explore whether deep convolutional neural networks (DCNNs) have the potential to improve diagnostic efficiency and increase the level of interobserver agreement in the classification of thyroid nodules in histopathological slides. Methods: A total of 11,715 fragmented images from 806 patients' original histological images were divided into a training dataset and a test dataset. Inception-ResNet-v2 and VGG-19 were trained using the training dataset and tested using the test dataset to determine the diagnostic efficiencies of different histologic types of thyroid nodules, including normal tissue, adenoma, nodular goiter, papillary thyroid carcinoma (PTC), follicular thyroid carcinoma (FTC), medullary thyroid carcinoma (MTC) and anaplastic thyroid carcinoma (ATC). Misdiagnoses were further analyzed. Results: The total 11,715 fragmented images were divided into a training dataset and a test dataset for each pathology type at a ratio of 5: 1. Using the test set, VGG-19 yielded a better average diagnostic accuracy than did Inception-ResNet-v2 (97.34% vs. 94.42%, respectively). The VGG-19 model applied to 7 pathology types showed a fragmentation accuracy of 88.33% for normal tissue, 98.57% for ATC, 98.89% for FTC, 100% for MTC, 97.77% for PTC, 100% for nodular goiter and 92.44% for adenoma. It achieved excellent diagnostic efficiencies for all the malignant types. Normal tissue and adenoma were the most challenging histological types to classify. Conclusions: The DCNN models, especially VGG-19, achieved satisfactory accuracies on the task of differentiating thyroid tumors by histopathology. Analysis of the misdiagnosed cases revealed that normal tissue and adenoma were the most challenging histological types for the DCNN to differentiate, while all the malignant classifications achieved excellent diagnostic efficiencies. The results indicate that DCNN models may have potential for facilitating histopathologic thyroid disease diagnosis.
更多查看译文
关键词
Deep convolutional neural network (DCNN),Inception-ResNet-v2,VGG-19,thyroid nodule,diagnostic efficiency,histopathology
AI 理解论文
溯源树
样例
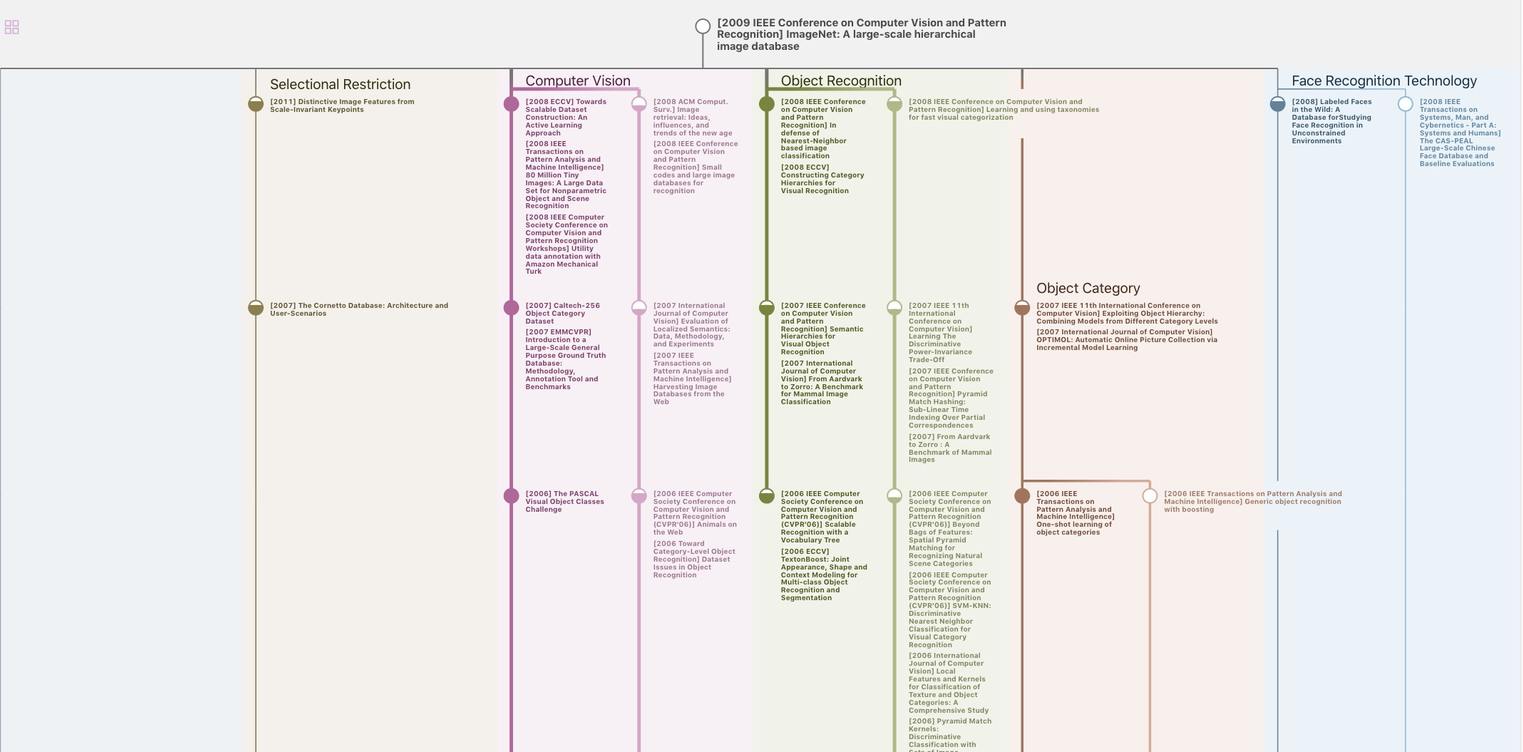
生成溯源树,研究论文发展脉络
Chat Paper
正在生成论文摘要