The Principles and Limits of Algorithm-in-the-Loop Decision Making
Proceedings of the ACM on Human-Computer Interaction(2019)
摘要
The rise of machine learning has fundamentally altered decision making: rather than being made solely by people, many important decisions are now made through an "algorithm-in-the-loop'' process where machine learning models inform people. Yet insufficient research has considered how the interactions between people and models actually influence human decisions. Society lacks both clear normative principles regarding how people should collaborate with algorithms as well as robust empirical evidence about how people do collaborate with algorithms. Given research suggesting that people struggle to interpret machine learning models and to incorporate them into their decisions---sometimes leading these models to produce unexpected outcomes---it is essential to consider how different ways of presenting models and structuring human-algorithm interactions affect the quality and type of decisions made. This paper contributes to such research in two ways. First, we posited three principles as essential to ethical and responsible algorithm-in-the-loop decision making. Second, through a controlled experimental study on Amazon Mechanical Turk, we evaluated whether people satisfy these principles when making predictions with the aid of a risk assessment. We studied human predictions in two contexts (pretrial release and financial lending) and under several conditions for risk assessment presentation and structure. Although these conditions did influence participant behaviors and in some cases improved performance, only one desideratum was consistently satisfied. Under all conditions, our study participants 1) were unable to effectively evaluate the accuracy of their own or the risk assessment's predictions, 2) did not calibrate their reliance on the risk assessment based on the risk assessment's performance, and 3) exhibited bias in their interactions with the risk assessment. These results highlight the urgent need to expand our analyses of algorithmic decision making aids beyond evaluating the models themselves to investigating the full sociotechnical contexts in which people and algorithms interact.
更多查看译文
关键词
behavioral experiment, ethics, fairness, mechanical turk, risk assessment
AI 理解论文
溯源树
样例
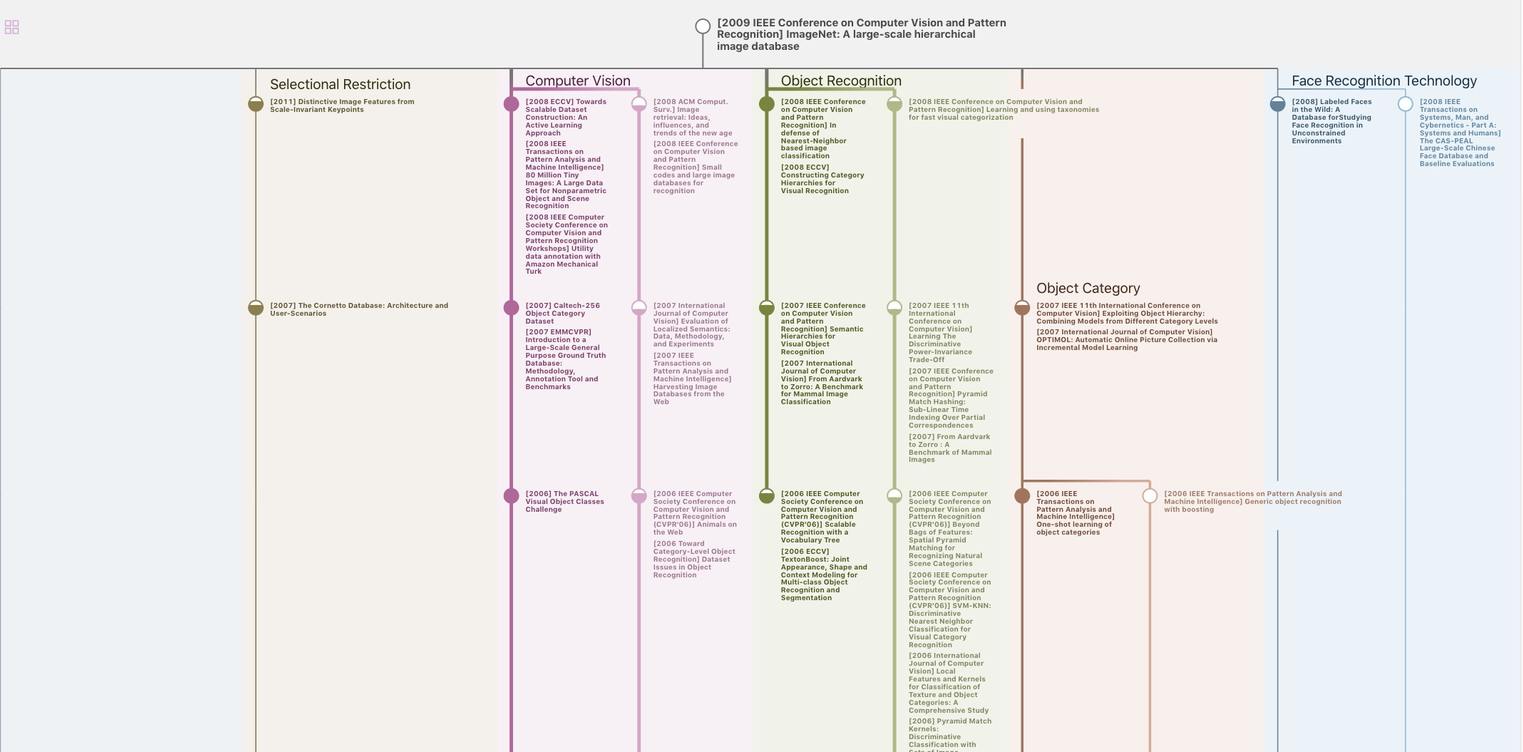
生成溯源树,研究论文发展脉络
Chat Paper
正在生成论文摘要