Deep-Person: Learning discriminative deep features for person Re-Identification
PATTERN RECOGNITION(2020)
摘要
Person re-identification (Re-ID) requires discriminative features focusing on the full person to cope with inaccurate person bounding box detection, background clutter, and occlusion. Many recent person Re-ID methods attempt to learn such features describing full person details via part-based feature representation. However, the spatial context between these parts is ignored for the independent extractor on each separate part. In this paper, we propose to apply Long Short-Term Memory (LSTM) in an end-to-end way to model the pedestrian, seen as a sequence of body parts from head to foot. Integrating the contextual information strengthens the discriminative ability of local feature aligning better to full person. We also leverage the complementary information between local and global feature. Furthermore, we integrate both identification task and ranking task in one network, where a discriminative embedding and a similarity measurement are learned concurrently. This results in a novel three-branch framework named Deep-Person, which learns highly discriminative features for person Re-ID. Experimental results demonstrate that Deep-Person outperforms the state-of-the-art methods by a large margin on three challenging datasets including Market-1501, CUHK03, and DukeMTMC-reID.
更多查看译文
关键词
Person Re-ID,LSTM,Triplet loss,End-to-end
AI 理解论文
溯源树
样例
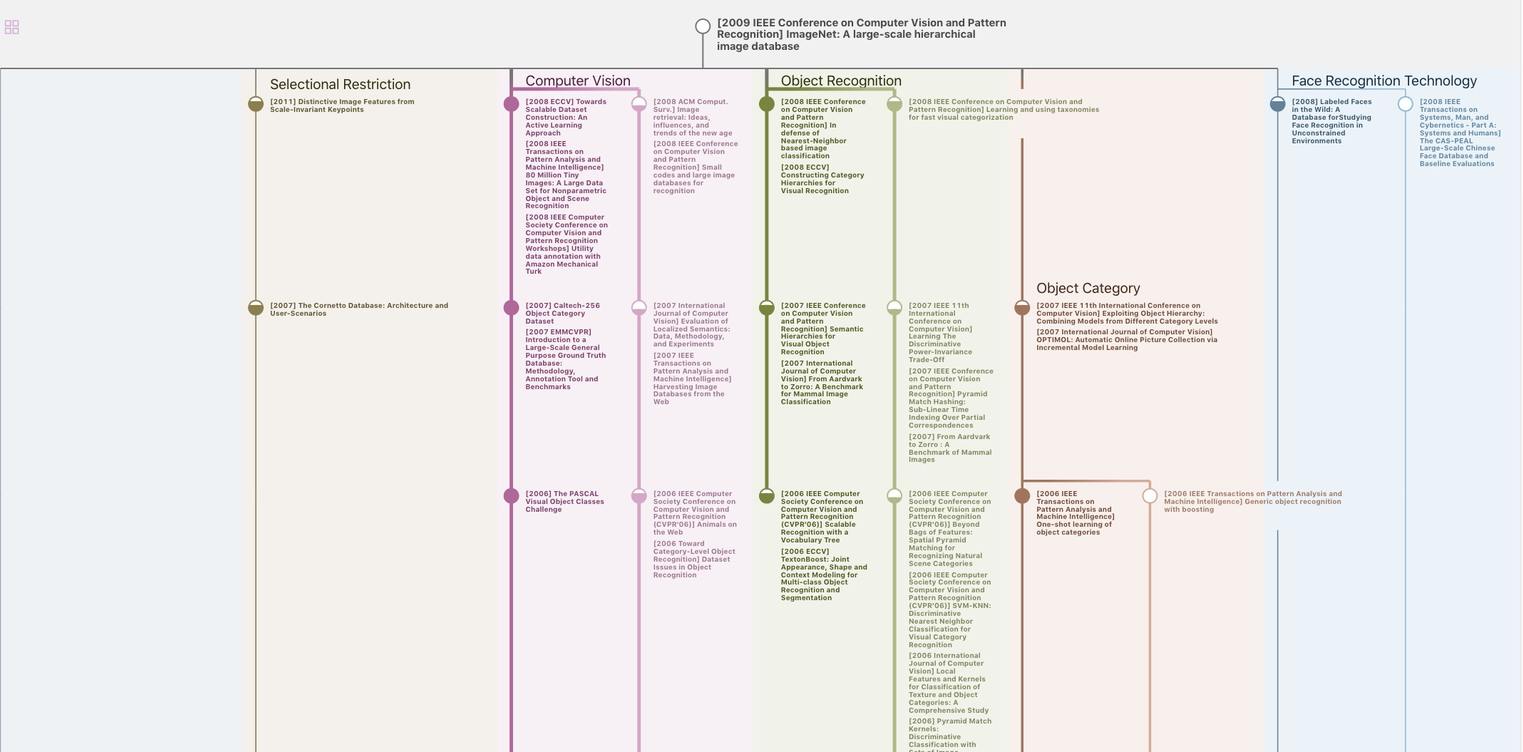
生成溯源树,研究论文发展脉络
Chat Paper
正在生成论文摘要