Modular Ontology Learning with Topic Modelling over Core Ontology.
Procedia Computer Science(2019)
摘要
Nowadays, modular domain ontology, where each module represents a subdomain of the ontology domain, facilitates the reuse of information and provides users with domain-specific knowledge. In this paper, we focus on modular taxonomy learning from text, where each module collects terms with the same topic insights, and in parallel we manage to discover hypernym and 'related' relations among those collected terms.However, it is difficult to automatically fit terms into modules and discover relations.We propose to employ twice trainedLDAto partition termsof each subdomain, and relate subdomains into modules of ontology. Meanwhile, we apply core concept replacement and subdomain knowledge supplementation as supportive information embedding technique over the corpus. This shows that the twice trained LDA strategy can effectively identify topic-relevant terms into subdomains, with nearly two-fold precision comparing to that of normal LDA training. The combination of core concept replacement and subdomain knowledge supplementation contributes to significant improvements in modular taxonomy learning.
更多查看译文
关键词
LDA,term partition,modular ontology,ontology learning,core ontology,knowledge supplementation
AI 理解论文
溯源树
样例
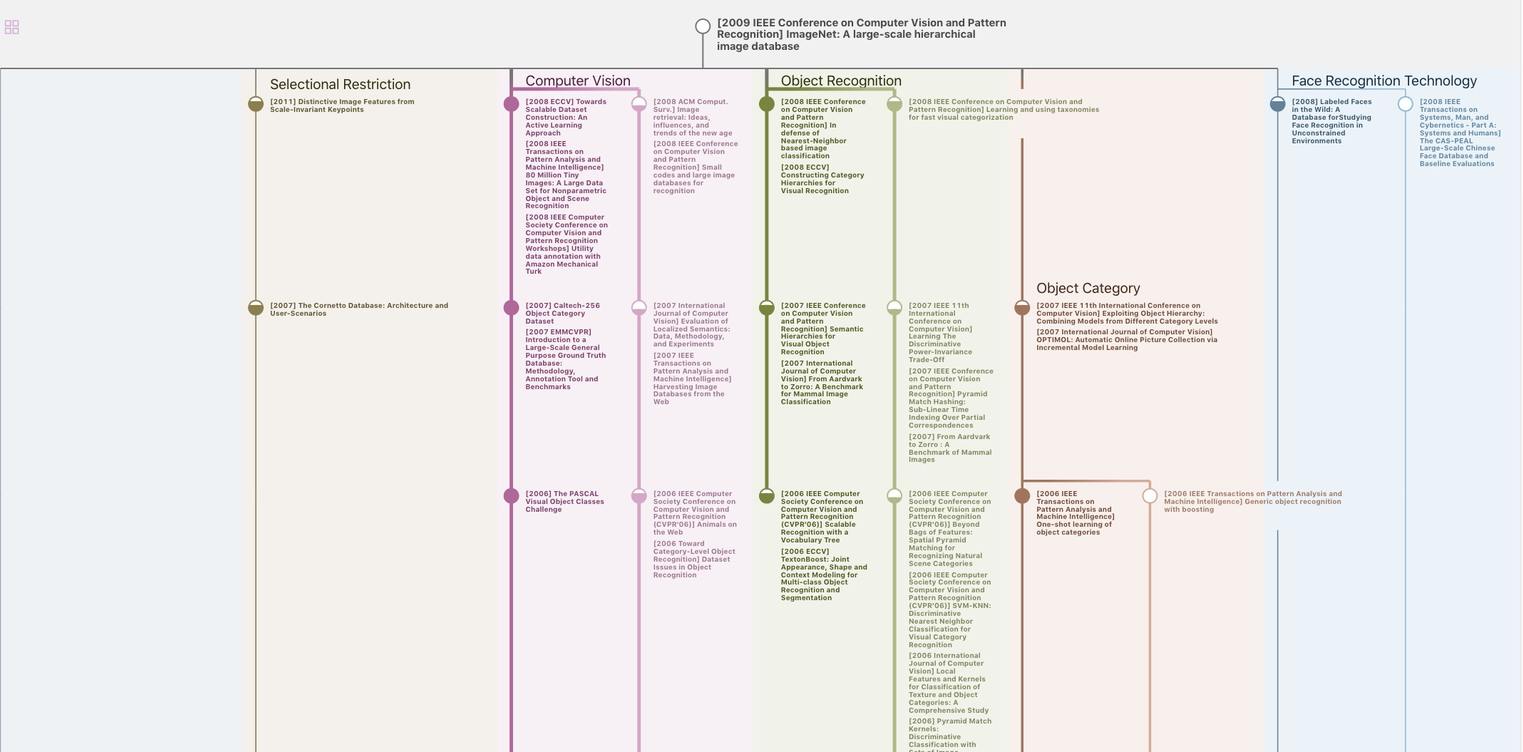
生成溯源树,研究论文发展脉络
Chat Paper
正在生成论文摘要