Where Is The Fake? Patch-Wise Supervised Gans For Texture Inpainting
2020 IEEE INTERNATIONAL CONFERENCE ON IMAGE PROCESSING (ICIP)(2020)
摘要
We tackle the problem of texture inpainting where the input images are textures with missing values along with masks that indicate the zones that should be generated. Many works have been done in image inpainting with the aim to achieve global and local consistency. But these works still suffer from limitations when dealing with textures. In fact, the local information in the image to be completed needs to be used in order to achieve local continuities and visually realistic texture inpainting. For this, we propose a new segmentor discriminator that performs a patch-wise real/fake classification and is supervised by input masks. During training, it aims to locate the generated parts (which we will call fake parts in consistency with the GAN framework), thus making the difference between real and fake patches given one image. We tested our approach on the publicly available DTD dataset, as well as Electo-Magnetic borehole images dataset and showed that it achieves state-of-the-art performances and better deals with local consistency than existing methods.
更多查看译文
关键词
Computer Vision, Texture inpainting, Generative Adversarial Networks, Segmentation
AI 理解论文
溯源树
样例
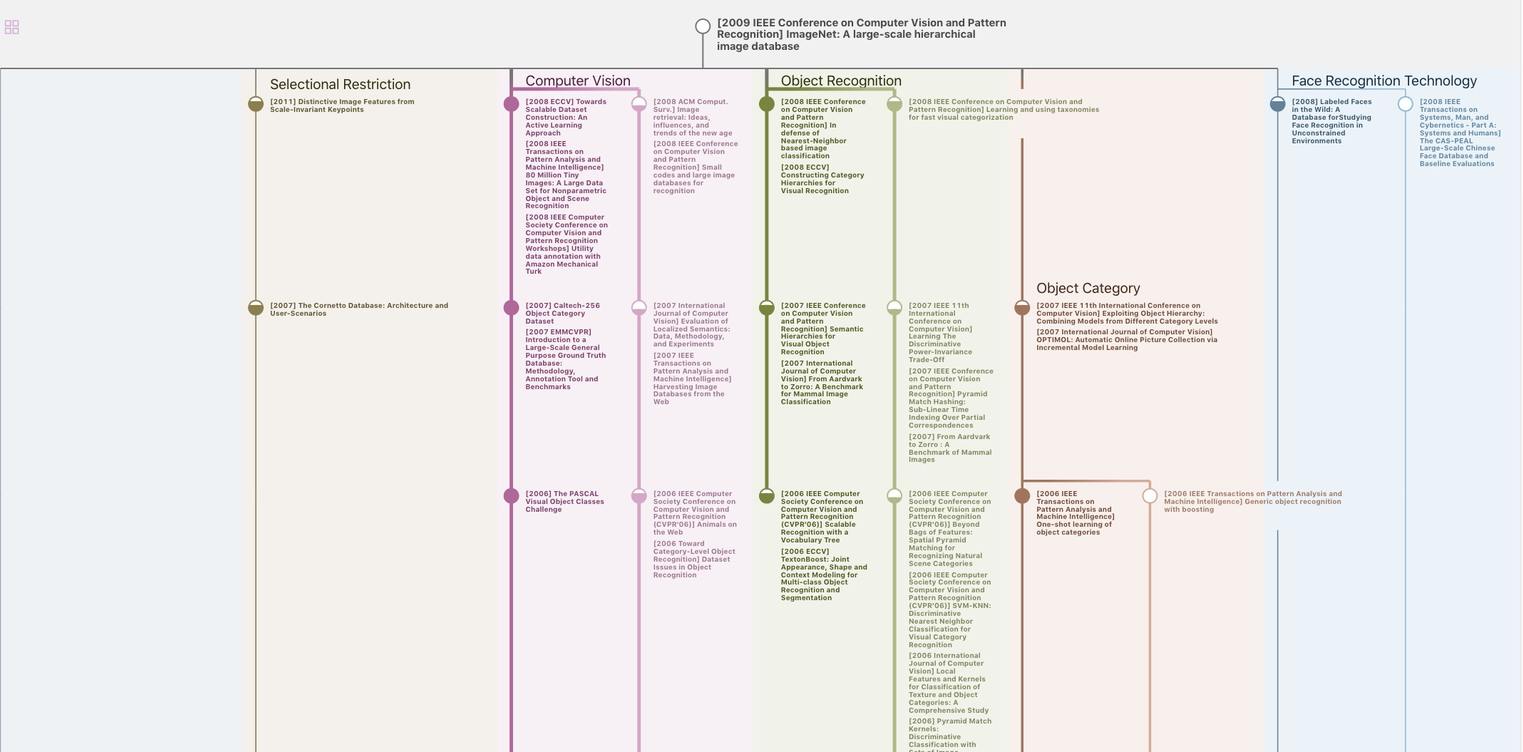
生成溯源树,研究论文发展脉络
Chat Paper
正在生成论文摘要